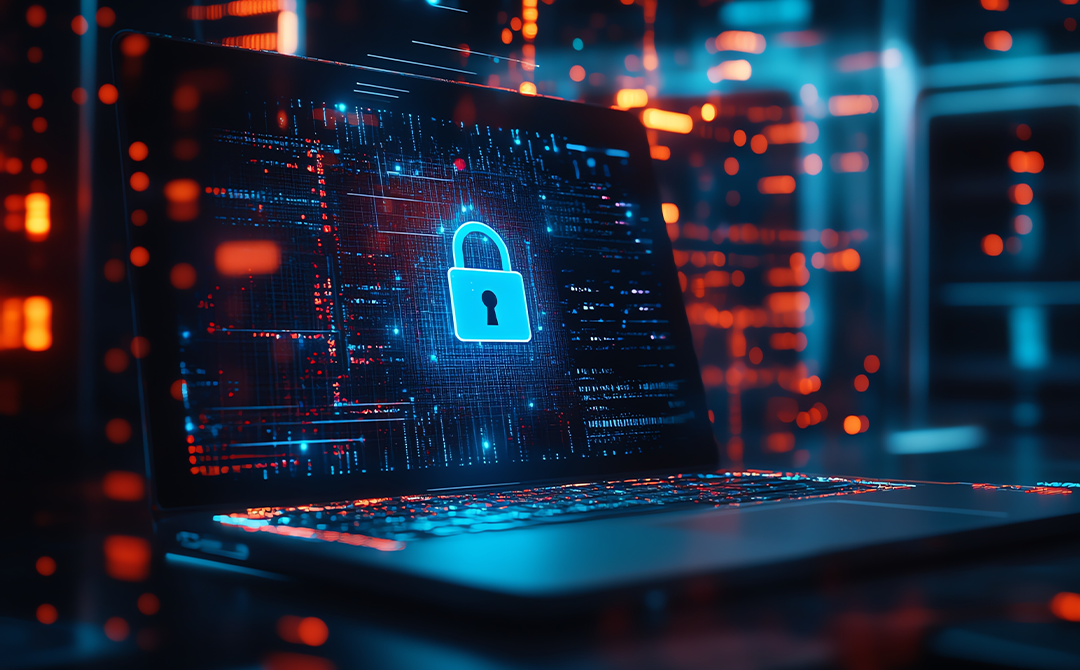
Today, AI drives mission-critical decisions across industries, from healthcare and finance to retail and manufacturing. Ensuring these AI-driven insights remain reliable and secure lies in a single, non-negotiable principle - AI data governance.
As AI adoption accelerates, businesses must implement a comprehensive AI data governance framework to ensure security, transparency, and regulatory compliance. A structured approach with clear policies and validation mechanisms enables companies to responsibly scale AI and unlock its full potential while building stakeholder trust.
The ability to operationalize AI governance and prioritize data integrity, security, and compliance will give businesses a competitive edge in today's AI-driven economy.
This article outlines the foundational steps and strategic considerations for effective AI governance.
What is AI Data Governance?
AI data governance defines the roles, responsibilities, and protocols necessary to ensure AI-driven systems remain secure, accurate, and traceable. Beyond compliance, it connects AI deployment directly to strategic business outcomes.
An effective AI data governance framework eliminates silos. It is structured, scalable, and designed to evolve. Core components include:
Data Classification and Access Control: Clear data labeling paired with tightly managed permissions ensures sensitive data is accessible only to authorized personnel.
- Data Lifecycle Management: From ingestion to retirement, data is governed by policies that protect integrity, ensure usability, and meet regulatory requirements.
- Auditing and Monitoring: Real-time visibility into data lineage and model behavior helps detect anomalies early before they become risks.
- Change Administration: Governance frameworks must grow alongside the business. Dynamic oversight ensures that governance adapts to emerging AI use cases and enterprise priorities.
By 2027, nearly 60 percent of organizations investing in AI will fail to realize its potential value due to weak governance practices. (Source: Gartner, Inc.) This highlights that the AI ecosystem requires rigorous governance across the entire lifecycle, from data ingestion and model training to deployment and continuous monitoring.
Why AI Data Governance is Indispensable for Businesses
AI adoption allows organizations to unlock new efficiencies and accurate insights. However, it introduces structural challenges, including fragmented data, inconsistent controls, and limited visibility into decision-making pipelines—all symptoms of insufficient data governance. Without a foundation of trust in the data fueling AI systems, even the most sophisticated models produce outputs that lack contextual alignment and ethical grounding.
This disconnect became evident when experts at a recent enterprise AI summit acknowledged the increasing pressure to deliver measurable outcomes within two years.
Some even admitted that AI-generated insights, at times, outperformed seasoned experts. However, accuracy without accountability is a dangerous proposition. AI-driven decisions must reflect analytical rigor, organizational intent, and values.
AI cannot operate in silos. Every insight it generates and every decision it supports is only as reliable as the framework behind it. This is where a robust AI data governance framework becomes indispensable. When woven into the operational fabric, governance enables organizations to de-risk AI initiatives, uphold compliance, and build resilient, explainable, and scalable systems.
Turning AI Data Governance Into a Strategic Advantage
AI continues to disrupt industries at an unprecedented speed. However, without a structured governance approach, businesses risk losing more than they gain. A forward-thinking AI data governance framework does more than reduce risk. It enables data-driven, informed decisions, operational agility, and seamless collaboration, unlocking competitive differentiation at scale.
Innovation Without the Guesswork
Companies embedding AI governance into their digital fabric move faster with greater confidence. By establishing clear ethical and operational guardrails, governance accelerates innovation while managing risk. Teams no longer hesitate to explore new tools. They build, test, and deploy, knowing the foundation is secure.
Collaboration Drives Results
Unclear data ownership and inconsistent standards stall AI initiatives. AI data governance breaks through friction, defining roles, aligning access, and ensuring data quality. This empowers data teams, ensures compliance, and fosters structured collaborations, driving faster value and greater traction for AI projects.
Decisions You Can Stand Behind
A mature AI data governance framework ensures data is clean, consistent, and traceable, making outcomes explainable and defensible. Whether internal strategy shifts or external audits, organizations can confidently stand behind their choices with data.
Staying Ahead by Staying in Control
Unchecked AI can introduce bias, expose private data, or create operational blind spots. Strong governance allows teams to detect issues early and act fast to preserve continuity and protect brand integrity. AI data governance becomes the difference between reacting to problems and preventing them.
Building Market Trust Through Leadership
Organizations leading with structured governance stand out in a market crowded with experimentation. They build responsibly, perform consistently, and earn trust across stakeholders. Reputation is a lasting advantage in the intelligence economy.
AI data governance goes beyond compliance. It is a strategic enabler that drives innovations, fosters collaborations, and allows businesses to lead confidently in a data-driven economy.
Understanding the Drawbacks of Not Implementing AI Data Governance
AI’s potential is undeniable, but when deployed without thoughtful oversight, the systems designed to bring efficiency and insight can introduce risk, bias, and reputational harm instead. It all starts with the data. If the foundation is not governed, the results can become unreliable at best and damaging at worst. AI data governance is the mechanism that identifies blind spots before they impact trust, compliance, or ethical operations. Without this, companies are exposed to several critical vulnerabilities.
Privacy at Risk: Re-identification Through Unstructured Data
AI systems thrive on large-scale data, but scale without structure invites exposure. Even anonymized datasets can be reverse-engineered, revealing personal identities when safeguards are not built into the framework. The absence of a clear AI data governance framework increases the likelihood of data misuse, privacy violations, and legal consequences.
Regulatory Pressure and Ethical Accountability
Regulations are evolving fast, and AI systems are expected to be explainable, transparent, and accountable. Organizations face a growing gap between innovation and compliance without clear policy guidance and a traceable audit path. A robust governance framework positions businesses to lead responsibly.
The Grey Zone of Intellectual Property
Generative AI introduces a new layer of complexity. When trained on vast datasets, models can inadvertently reproduce proprietary or copyrighted content. Without governance, companies risk IP disputes, unclear licensing, and exposure to litigation. A strong data governance approach defines boundaries, manages sourcing, and ensures AI outputs stay within legal limits.
Unmanaged AI introduces complexity, not clarity. But when built on a governed foundation, AI becomes a strategic advantage—secure, ethical, and aligned with long-term goals. AI data governance is not a cost center but the backbone of trust, equity, and defensibility in enterprise AI systems.
The Dos and Don'ts of AI Data Governance
Setting up robust AI data governance requires both strategic planning and proactive day-to-day management. Companies must ensure their systems follow the rules and match their own values and business plans. This section discusses what works well and what to avoid when managing AI. By tackling these points organized, companies can create a space where AI is accountable and holds integrity.
The Dos: Best Practices
- Data Quality: Ensure that all datasets for AI models are complete and well-managed. Organized data makes models reliable and reduces biases and mistakes.
- Clear Data Management Roles: Give people the job of looking after datasets from start to finish. These managers help enforce rules, clear up confusion, and ensure everything follows the policies.
- Privacy and Security: Establish stringent access controls and encryption while following laws like GDPR and CCPA. Keeping data safe is key to AI systems you can trust.
- Transparency and Accountability: Record each step in the AI process, from where you obtain your data to how the model makes decisions. This clarity allows for informed oversight and helps build trust with stakeholders.
- Regular Audits: You need to review regularly how well your governance works. This helps you adapt to new risks, rules changes, and ways to use AI. Governance is something you set up once and keep evolving.
The Don’ts: What to Avoid?
- Poor Data Quality Control: Mixed-up data leads to models you cannot trust and incorrect results. This impacts how well they work and makes people doubt them.
- Missing Ethical Checks: Not thinking about ethics can make AI unfair or harmful. Leaders need to make sure ethics are checked at every step.
- Regulatory Non-Compliance: Ignoring data protection laws in your area or worldwide can expose your company to legal troubles and reputational damage.
- Absence of Ongoing Audits: AI systems change over time. Without continuous monitoring, data patterns may lead to inaccurate decisions.
- Inadequate Documentation: Poor record-keeping makes tracking accountability, maintaining consistency, and effectively responding to audits challenging.
The success of any AI project depends on strict governance. You can scale AI with confidence and control by implementing these best practices into daily operations and avoiding common errors.
Real-World Applications of AI Data Governance
The gap between testing and full-scale success depends on how well the businesses manage their data resources. AI data governance helps turn theoretical models into practical value by making AI systems responsible, flexible, and aligned with business goals. This section combines the hands-on uses of AI Data Governance and its measurable impact.
Boosting Strategic Alignment in AI Projects
Companies with AI data governance see better teamwork between data scientists and business leaders. For example, if a big consumer goods company uses a well-planned governance program to set rules for data use, its models are more accurate, and it ensures AI suggestions match business goals for different product categories.
Ethical and Legal AI
AI tools used to create content have raised serious debates about ethics and false information. Big news stories about AI-generated legal references and biased content have led to new rules and public doubt. On the other hand, companies with governance plans, such as ethical checks and regulations, for use are in a better position to avoid these problems and follow changing laws.
Making Public Sector AI Use More Clear
US Government agencies are starting to use AI systems to make administrative tasks easier. But people will trust these new technologies only when they are easy to understand and fair. Agencies that have implemented AI data governance rules have had better luck getting public support when they use AI. These rules include tracing where training data comes from and keeping records of how decisions are made (Source: U.S. Government Accountability Office).
These real-life cases show that AI data governance is a practical way to ensure responsible AI use, bringing greater industry results. Whether in business or government, using governance rules turns AI from a potential problem into a reliable business tool.
How AI Data Governance Has an Impact on Shaping a Secure Future
As AI moves from testing to large-scale use in businesses, it greatly influences security, ethics, and public trust. With worldwide worries about data abuse, unclear algorithms, and built-in bias on the rise, companies need to look past quick AI setups and consider the long-term effects of their choices on management. AI data governance lays the groundwork to build resilient, ethical systems ready for what lies ahead. It sets up the company structure to spot and handle new risks while boosting openness, following rules, and sparking new ideas
Readiness for Faster Rule-Making
Governments and regulatory bodies worldwide are introducing new rules to control AI use. Examples include the European Union's AI Act and executive orders in the United States. These new rules often require companies to show they can explain their AI, reduce bias, and take responsibility for their algorithms. A good AI data governance framework helps businesses stay ahead of these changes instead of just trying to avoid fines.
Building Trust Through Transparency
AI's success hinges on trust from the public and institutions in industries like healthcare, finance, and shipping that deal with huge volumes of data. This trust cannot be gained just through good technical performance. It needs transparency about where data comes from, how models work, and how decisions are made. AI data governance ensures each step of the AI process, such as getting data ready, training, checking, and using, is recorded and can be traced.
Helping Responsible New Ideas
Many companies find it hard to balance new ideas with managing risks. By building governance into the early parts of AI development, businesses can try new uses without giving up ethical oversight or following rules. This speeds up innovation while keeping control, letting companies grow AI safely and effectively. AI data governance builds a long-term competitive edge. Companies adopting a forward-thinking governance approach can navigate the changing AI scene and lead the way in responsible change.
The next big thing for AI will depend on how well businesses manage their data and follow the rules. Companies that use AI without proper safeguards might lose people's trust, break laws, and fall behind their competitors. Keeping AI data in check ensures that AI systems are safe, ethical, and meet business requirements. It helps make decisions clearer, results easier to track, and everyone is more responsible.
At Tredence, our AI consulting services aim to help companies integrate AI governance into the core of their analytics plan. We partner with businesses to make AI adoption game-changing and long-lasting, from crafting AI ethics rules to rolling out complete governance systems.
Contact us to find out more about how your company can implement responsible AI on a large scale.
FAQs
How does AI data governance differ from traditional data governance?
AI data governance deals with unique AI hiccups like lessening bias, making models transparent, and owning up when bots make decisions. Traditional data handling is more about getting the facts right and keeping them safe, but when we are talking about AI, it is all about keeping things moral, clear to understand and up to date with the latest rules.
Why is AI data governance critical for regulatory compliance?
AI's role in data governance ensures businesses stick to data protection standards like GDPR, CCPA, and emerging AI regulations. By setting definite protocols for data management, displaying how models function, and accepting accountability for algorithm behavior, companies can avoid legal issues and gain trust from important individuals while maintaining AI's ethical aspects.
How can AI data governance help prevent model drift?
Model drift is when AI models decline in accuracy over time due to changing data trends. AI data governance aids in this by monitoring data quality with frequent evaluations and ensuring regular retraining of models. This proactive stance helps keep AI models effective, unbiased, and dependable.
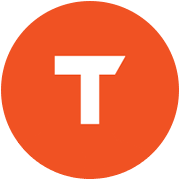
AUTHOR - FOLLOW
Editorial Team
Tredence
Next Topic
Databricks AI Builder: Revolutionizing LLM Fine-Tuning Without Labeled Data
Next Topic