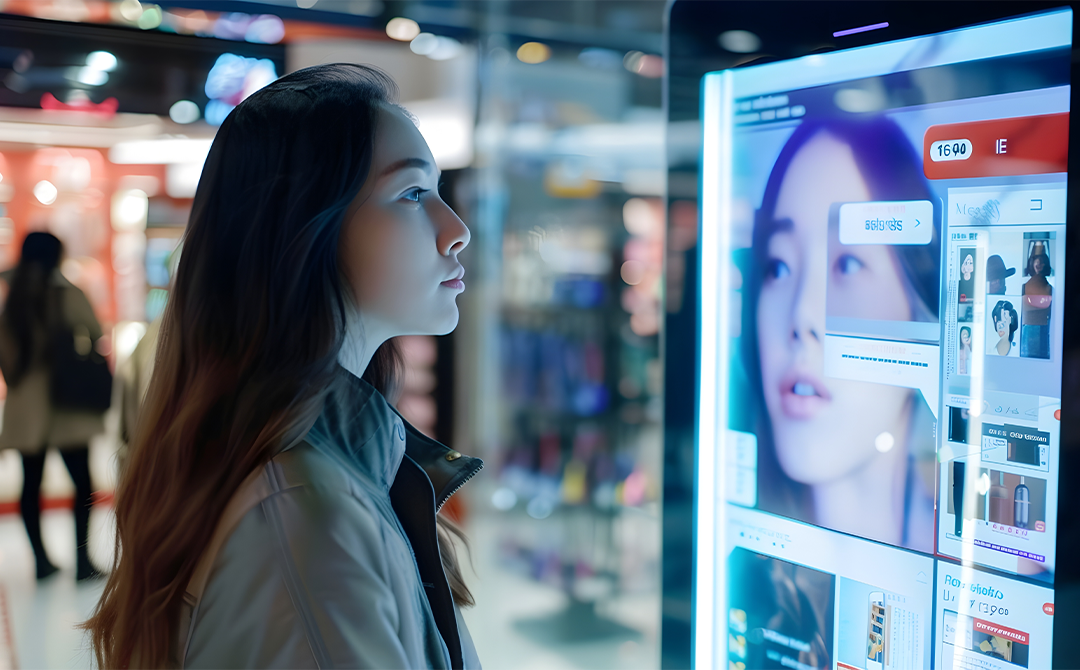
If you've worked in marketing, you've undoubtedly heard about data clean rooms-touted as the next big thing for personalized targeting, campaign efficiency, and marketing measurement. With the deprecation of third-party cookies, these secure environments offer a privacy-safe way to personalize campaigns, boost efficiency, and accurately measure marketing impact.
In this article, we move beyond the basic "what" of clean rooms to explore the practical applications for marketers: how clean rooms go beyond standard reporting, how to build effective marketing solutions within them, and where expectations meet reality when it comes to their limitations towards unified marketing measurement.
Beyond Just Standard Reporting
With the decline of third-party cookies, marketers have had to rethink how they measure campaign performance. Traditional methods-like first-party attribution and publisher-based attribution-offer some visibility, but each comes with its limitations. Clean rooms have emerged as a step forward, providing more control, granularity, and privacy-compliant measurement. But how exactly do they compare to existing methods?
The table below outlines how attribution has evolved, highlighting the benefits and trade-offs at each stage:
Evolution of Attribution: From First-Party Data to Clean Rooms
To understand this shift, let's first define the key attribution methods and then explore their pros and cons to see how measurement has evolved:
- First-Party Attribution: Advertisers collect first-party cookies directly from their websites, tracking where customers came from before landing on their site. By combining this data with their first-party advertising data, they can reconstruct the customer journey and measure campaign impact.
- Publisher-Based Attribution: Advertisers share their first-party transaction data with publishers through data-sharing mechanisms like Conversions API. The publisher then applies its own attribution models to calculate revenue and Return on Ad Spend (ROAS), providing advertisers with insights but limiting transparency and customization.
- Clean Room-Based Measurement: Both publishers and advertisers contribute data to a privacy-controlled environment, allowing advertisers to build their own attribution models while maintaining compliance. This approach grants more flexibility and control over measurement but comes with its own set of complexities.
Clean rooms provide the most advanced measurement capabilities while ensuring privacy compliance. However, they aren't a silver bullet-data remains siloed across platforms, limiting a unified view of marketing performance. While they offer better control over attribution modeling and customer matching, privacy constraints make implementation complex.
Next, we'll break down the strategies for creating a powerful clean room measurement solution by comparing the implementation approaches of two giants in the space-Meta AA and Google's ADH. Let's dive in and see how these walled gardens stack up!
Crafting Clean Room Measurement: Meta AA vs. Google ADH in Action
Attribution modeling in clean rooms in theory seems straightforward-publishers provide customer-level impression data, advertisers upload transaction data, and a clean room-specific customer matching process is applied. From there, attribution models are built using SQL-based query languages, and once processed, data is extracted at an aggregated level for analysis. However, while the process may appear simple, there are complexities involved in building and managing clean rooms effectively.
To better understand this process, let's break it down step by step by comparing two of the largest walled garden clean rooms: Meta Advanced Analytics (AA) and Google Ads Data Hub (ADH) [Meta AA is often referred to as a clean room, but its capabilities are quite limited in practice.
- Manual query approvals slow down analysis.
- No access to raw records for debugging.
- No cross-platform interoperability (walled gardens). | - Data extraction limits are dependent on tables (10 for clicks/conversion, 50 for impressions - estimated)
- Privacy restrictions prevent user re-identification.
- No real-time data access (potential delays).
- Limited cross-channel visibility. | | Best Practices | - Validate data early with simple queries. - Use macros for efficiency.
- Plan for delays due to manual approvals. | - Validate data with test queries in the sandbox.
- Account for data latency in reporting timelines.
- Leverage Looker Studio for visualization. |
Clean rooms like Meta AA and Google ADH offer advanced attribution capabilities while ensuring data privacy. However, challenges such as cross-platform data fragmentation, privacy restrictions, and delayed query processing remain. Marketers must navigate these constraints carefully to build scalable, privacy-compliant attribution models.
The Realities of Clean Room Implementation: What to Expect
Clean rooms offer a powerful framework for unifying marketing measurement, but marketers need to be aware of the challenges and limitations that come with their implementation. While they enable data collaboration without sacrificing privacy, clean rooms often work with aggregated data, which may limit the granularity of insights typically available through first-party data. This trade-off can impact the depth of analysis, especially when marketers are accustomed to more detailed customer-level data.
Another challenge lies in integrating data from various sources. While clean rooms promise a unified approach, aligning data across different platforms often introduces complexity, leading to potential discrepancies and difficulties in achieving a holistic view of the customer journey. In practice, achieving this view is particularly difficult-while walled gardens like Meta and Google restrict sharing data outside their ecosystems, the data from other platforms is more of a potential, often discussed in theory, rather than a fully realized reality.
In conclusion, while clean rooms offer a valuable framework for collaborative data analysis, marketers should approach them with a clear understanding of their limitations and manage expectations accordingly. Clean rooms are not a one-size-fits-all solution but rather an important piece of a larger, integrated marketing measurement ecosystem.
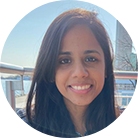
AUTHOR - FOLLOW
Charvi Nagpal
Manager, CXM
Next Topic
Mastering Databricks Apps: From Creation to Deployment of Interactive Data Applications
Next Topic