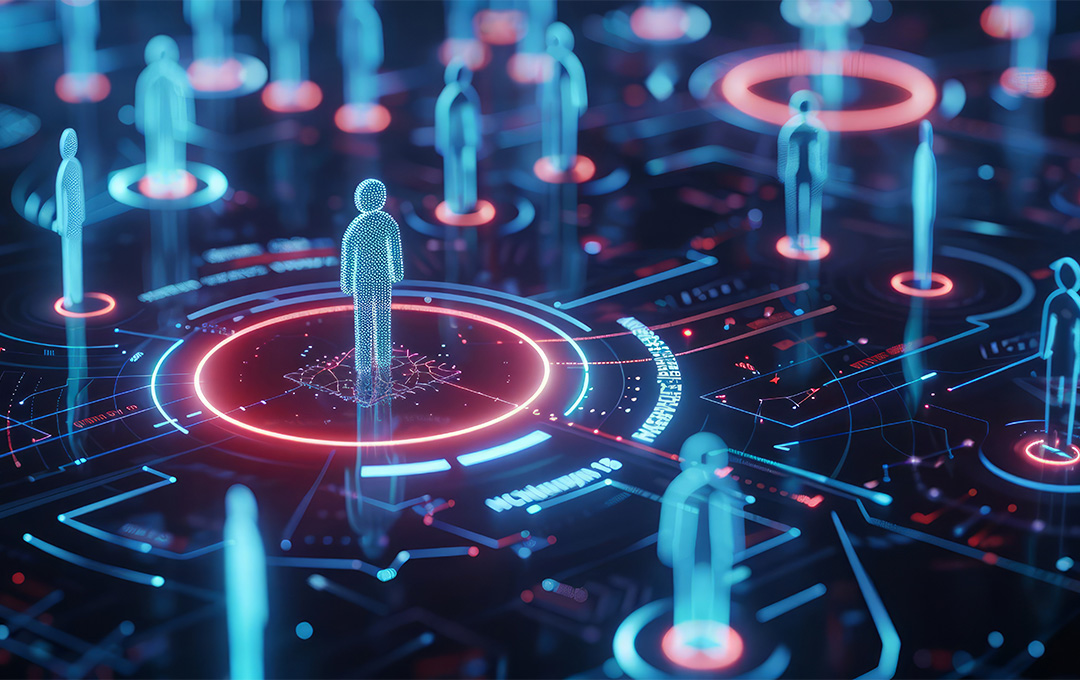
Imagine trying to sell umbrellas by guessing who might need them without ever checking the weather forecast. That is precisely what traditional customer segmentation feels like in our current business reality. You might occasionally succeed, but more often, you are wasting valuable resources broadcasting messages into the void.
The reality is that customers do not fit neatly into predefined categories like "Millennial professionals" or "urban parents." Their behaviors shift based on mood, context, season, and even the device they are using. Treating them as static groups is similar to navigating with outdated maps. You will inevitably lose your way in the competitive marketplace.
Consider this impact: According to McKinsey & Company, companies with faster growth rates derive 40% more of their revenue from personalization than their slower-growing counterparts. Yet many organizations still rely on outdated, assumption-heavy models that fail to capture the nuances of real customer behavior. [Source: Mckinsey]
This is where AI customer segmentation transforms the landscape. It listens rather than assumes. It adapts instead of generalizes. By analyzing real-time behavioral signals, it helps you understand not just who your customers are but what they are likely to do next, enabling truly data-driven decision making.
In this article, we will explore how AI customer segmentation works, common pitfalls to avoid, and the real-world use cases where it delivers exceptional business outcomes.
Unpacking AI Customer Segmentation
AI customer segmentation is the process of grouping customers using machine learning based on behavior, preferences, and predicted outcomes. Unlike traditional models that rely on static demographics, AI segments evolve in real time, making them highly responsive and accurate.
Core AI techniques powering this innovation include:
-
Clustering Algorithms: Group customers based on behavioral patterns. An e-commerce platform might identify late-night deal hunters, weekday business buyers, and weekend impulse shoppers.
-
Natural Language Processing: Analyzes unstructured text from reviews and feedback. A hotel chain can detect complaints about room cleanliness or identify what loyal guests value most.
-
Predictive Analytics: Forecasts future actions using historical data. A telecom company can flag customers likely to churn based on usage drops or service complaints.
-
Recommendation Engines: Identifies what customers want next. A music app can create segments like "gym-time listeners" for targeted messaging.
With these powerful AI techniques in mind, let's explore the step-by-step process that makes AI segmentation so effective.
Understanding the Workings of AI Customer Segmentation
AI customer segmentation is a continuous loop that evolves with your customers, following a clear sequence from data gathering to execution. Here is how it works:
- Data collection and integration Gather data from all customer touchpoints including CRM records, app usage, website activity, and support logs. A streaming platform might aggregate viewing duration, search history, and device preferences to create unified user profiles.
- Preprocessing and cleansing Clean and standardize raw data to remove missing fields, duplicates, or inconsistencies. For a streaming service, this might mean resolving multiple accounts in one household or removing incomplete viewing logs.
- Segmentation modeling Apply machine learning models to identify patterns and group customers. A platform might detect "weekend binge watchers" or "mobile-first viewers" segments that emerge from behavior patterns rather than demographics.
- Real-time updates Monitor new signals and update segments automatically as behavior changes. A "weekend-only viewer" who starts watching during weekday commutes would shift to a more relevant segment immediately.
- Actionable outputs Map segments to business actions including personalized recommendations, targeted promotions, or loyalty rewards. The platform might show long-form content to binge watchers and short videos to mobile users, improving engagement without treating all users the same.
Understanding the mechanics is important, but businesses also need to recognize the tangible benefits driving adoption across industries.
Why Smart Companies Are Adopting AI Customer Segmentation
Traditional segmentation treats customers like static labels. Modern customers evolve constantly in their shopping habits and engagement patterns. AI segmentation enables companies to keep pace with these changes:
- Adapt instantly to shifting behaviors AI models monitor activity and update segments in real time, ensuring your messaging reflects current behaviors, not last quarter's assumptions.
- Prioritize high-value users through predictive insights AI estimates future customer value by analyzing past behavior and engagement, helping teams focus on customers likely to generate long-term revenue.
- Spend smarter by targeting likely converters Instead of broad demographic targeting, AI enables precise targeting of high-intent customers, reducing acquisition costs and increasing campaign returns.
- Avoid churn by detecting risk patterns early By identifying signals of disengagement like reduced usage, AI helps businesses act before customers leave, improving retention and lifetime value.
- Improve personalization with detailed personas AI creates behavior-driven segments beyond basic demographics, powering personalized messaging and recommendations across channels.
While the advantages are clear, implementing AI segmentation comes with challenges that organizations must navigate carefully.
Biggest Mistakes to Avoid When Implementing AI Customer Segmentation
Even with the best tools, segmentation can fail if the fundamentals are not in place. Here is a breakdown of common mistakes, how to avoid them, and practical tips to get it right:
Mistake |
How to Avoid It |
Pro Tip |
Using limited or outdated data |
Incorporate behavioral and real-time data along with historical transactions. |
Ensure your data pipeline includes live sources like web, app, and CRM feeds. |
Not aligning segments with business goals |
Tie every segment to a measurable outcome—sales, retention, or engagement. |
Start with KPIs, then build segments around what actually moves them. |
Overengineering the model |
Keep models simple and interpretable for business users to understand and use. |
If stakeholders cannot explain the segment logic, simplify it. |
Ignoring ethical considerations |
Avoid using sensitive attributes or proxies that could introduce bias. |
Run fairness checks on your segmentation criteria before rollout. |
Failing to act on insights |
Ensure segmentation feeds directly into campaign workflows and execution tools. |
Align marketing, product, and sales teams early so insights turn into action. |
After understanding what not to do, let's focus on the strategic approach that ensures successful implementation.
How to Get AI Customer Segmentation Right
Implementing AI segmentation the right way is not just about having the right tools. It is about aligning technology with strategy and ensuring the entire organization is set up to act on insights. Here is a simple but effective roadmap:
1. Set clear objectives
Start with a well-defined goal. Whether it is reducing churn, increasing lifetime value, improving reactivation, or lowering acquisition costs, clarity on what success looks like will guide every decision from which data to collect to how results are measured.
2. Audit your data infrastructure
Strong segmentation depends on strong data. Ensure your systems can capture behavioral signals in real time, store them in a structured format, and make them accessible across teams. Also confirm that your data collection methods comply with regulations like GDPR and CCPA to avoid legal risks.
3. Choose the right tools
Look for platforms that support automation, offer explainable AI, and update segments in real time. The tools should integrate easily with your existing stack and allow business users not just data scientists to access and act on insights.
4. Build cross-functional teams
Effective segmentation is not just a data science task. Bring together marketers, analysts, product managers, and compliance officers. This ensures segments are relevant, insights are actionable, and campaigns stay within legal and ethical boundaries.
5. Test, learn, and iterate
Segmentation is not static. Start with pilot campaigns targeting a few high-value segments. Track performance, gather feedback, and refine your models continuously. Each cycle improves accuracy, relevance, and business impact.
Tredence has helped several global enterprises build segmentation strategies from the ground up. For instance, a Fortune 100 tech company partnered with Tredence to create a suite of AI-powered platforms, including a customer lifecycle manager and a real-time content engine, that automated segmentation, personalization, and campaign execution. This significantly improved engagement while reducing manual intervention.
Let us now examine specific applications that demonstrate the transformative impact of AI segmentation across different business functions.
The Best Use Cases on AI Customer Segmentation
AI segmentation can shift how businesses operate across industries. Here is how six key use cases would typically look before and after AI is applied:
These use cases aren't just for theoretical purposes, as leading companies are already seeing remarkable results from their AI segmentation initiatives.
Exploring Examples of AI Customer Segmentation
Leading organizations across industries are already leveraging AI segmentation to transform their customer experiences and business outcomes. These real-world applications demonstrate the tangible benefits of this technology:
Example 1: Netflix's Personalized Content Recommendations
Challenge: With a vast library of content, Netflix needed to ensure users could easily discover shows and movies that matched their preferences to enhance engagement and reduce churn.
AI Impact: Netflix developed an advanced recommendation system utilizing collaborative filtering, content-based filtering, and deep learning algorithms. [Source: teqfocus] By analyzing user behavior such as viewing history, ratings, and interaction patterns the AI segmented users into distinct profiles, including:
- Nighttime Binge-Watchers: Users who watch multiple episodes late at night.
- Weekend-Only Viewers: Subscribers who primarily watch content on weekends.
- Kids Content Families: Households with frequent views of children's programming.
- Global Explorers: Viewers who enjoy international or multi-language content.
Each segment received tailored recommendations, personalized homepage layouts, and customized notifications. This strategic segmentation led to increased user engagement and improved retention rates. [Source: edrawmind]
Example 2: IQVIA's Enhanced Patient Identification
Challenge: Healthcare providers often struggle to identify patients who could benefit from specific treatments, leading to delayed interventions and suboptimal care.
AI Impact: IQVIA leveraged AI and machine learning to analyze extensive real-world data, including medical records, treatment histories, and demographic information. The AI segmented patients based on factors such as:
- Appointment Frequency: Regularity of medical visits.
- Type of Medical Service: Specialties or services utilized.
- Payment Behavior: Insurance claims and payment patterns.
By identifying patients with chronic conditions or those at risk of non-adherence, healthcare providers could proactively offer tailored interventions, educational resources, and prioritized appointment scheduling. This approach significantly improved patient outcomes and satisfaction. [Source: IQVIA]
Similarly, Tredence worked with a global retailer to unify customer data from online, offline, and mobile channels using Google Cloud. This created a 360-degree view of every customer and enabled real-time segmentation across marketing, personalization, and forecasting functions.
As impressive as current applications are, the evolution of AI segmentation is accelerating toward even more sophisticated capabilities.
Where Is AI Segmentation Headed?
AI segmentation is moving from reactive to predictive. The next wave is smarter, more context-aware, and deeply personalized. Here is what is coming:
Real-time emotional segmentation
AI will analyze sentiment from chats, emails, and voice calls to detect customer satisfaction or frustration. A customer showing signs of irritation could be auto-routed to priority support or receive softer messaging before they churn.
Omnichannel segmentation
Data from apps, websites, stores, and support channels will merge into one profile. This allows businesses to segment based on full customer journeys, not isolated touchpoints.
Self-learning models
As user behavior shifts, AI will adjust segments automatically with no retraining needed. A once-weekly shopper who starts browsing daily will not stay in the same bucket for long.
AI-generated microsegments
AI will surface niche patterns like "holiday pet spenders" or "weekday wellness buyers." These microsegments unlock targeting opportunities that humans might overlook.
Tredence has already helped enterprise clients move toward this future. In one engagement, they built a Customer Data Platform (CDP) that enabled advanced machine learning segmentation, cross-functional access to customer insights, and person-level engagement across all owned assets
Given these advancements and opportunities, businesses need the right partner to navigate this complex but rewarding journey.
AI Customer Segmentation: How Tredence Leads the Way
AI customer segmentation is not just a marketing upgrade, it is a business advantage. It moves you from assumptions to insights, from static lists to dynamic audiences, and from missed opportunities to measurable growth.
Tredence makes that shift possible with enterprise-grade AI-consulting services. We combine deep industry expertise with advanced AI to help you build segmentation strategies that are not only intelligent but actionable, scalable, and ROI-driven.
What you get with Tredence:
- Real-time behavioral segmentation powered by machine learning
- Predictive models tailored to your KPIs including churn, LTV, upsell, or retention
- Transparent, explainable outputs for fast business adoption
- Integrated execution support across marketing, sales, and CX platforms
- End-to-end guidance from data readiness to activation
Do not just reach your customers, but understand them. Contact Tredence today to build segmentation that adapts, performs, and delivers results where it matters most.
FAQs
1. What data sources do AI models use for customer segmentation?
AI customer segmentation models analyze multiple data sources to create comprehensive customer profiles. These include CRM data, website interactions, purchase history, app usage, social media activity, support interactions, and demographic information. The integration of these diverse data points enables AI to identify patterns and behaviors that traditional segmentation methods might miss.
2. How can AI customer segmentation improve personalized marketing?
AI customer segmentation dramatically improves personalized marketing by creating dynamic, behavior-based segments that evolve in real time. Instead of static demographic groups, AI identifies patterns in customer interactions, preferences, and purchase history to deliver highly tailored content, recommendations, and offers. This leads to increased engagement, higher conversion rates, and improved customer loyalty through genuinely relevant experiences.
3. Can AI customer segmentation help businesses predict customer churn?
Yes, AI customer segmentation is highly effective at predicting customer churn. By analyzing behavioral signals like decreased usage, fewer logins, or changing engagement patterns, AI can identify at-risk customers before they leave. These early warning systems allow businesses to proactively implement retention strategies, such as personalized offers or enhanced support, significantly reducing churn rates and preserving customer lifetime value.
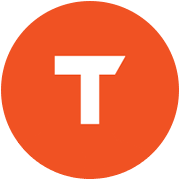
AUTHOR - FOLLOW
Editorial Team
Tredence