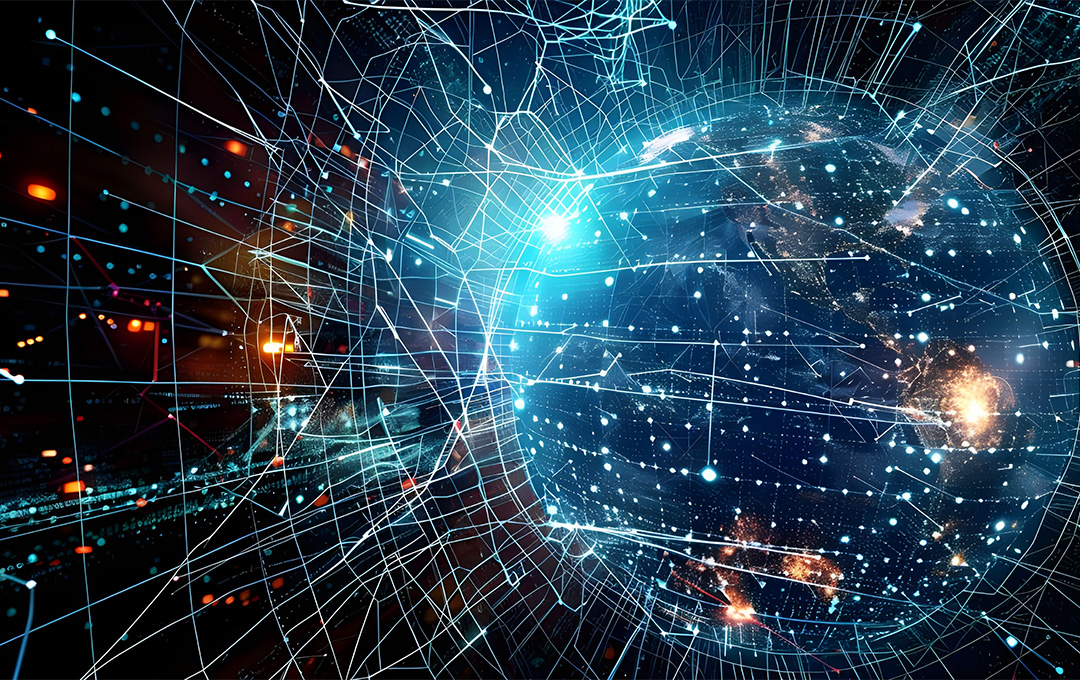
Today, large language models (LLMs) transform how enterprises serve customers, automate workflows, and generate insights at scale. However, as their influence grows, so does the risk of deploying them unchecked. Without strong LLM governance, organizations risk legal exposure, reputational harm, and critical failures in decision-making; it is not just an option but a business imperative. 71 percent of CEOs agree that establishing trusted AI requires robust AI governance. Even the most advanced models can undermine trust, compromise compliance, and erode business value without a structured governance approach. (Source: IBM)
In this article, we will explore what LLM governance entails, how it supports risk management and compliance, and why it is the backbone of enterprise-scale generative AI strategy. You will also see how industry leaders are building their LLM governance framework from the ground up—avoiding common pitfalls and unlocking long-term value. Let us break it down.
What is LLM Governance?
LLM governance is the set of principles and procedures defined for the management of large language models to ensure ethical use, regulatory compliance, risk mitigation, and alignment with business objectives.
LLMs are incredibly powerful—but they are not plug-and-play tools. Their ability to generate, interpret, and act on language at scale comes with real risks, including data privacy concerns, bias amplification, misinformation, and operational unpredictability. This is where LLM governance steps in—not as a limiter but as a necessary framework to ensure these models act responsibly, transparently, and in alignment with enterprise goals.
It combines principles of LLM observability, accountability, compliance, and control to make sure AI-powered systems are not just smart but safe, sound, and reliable.
Key Components of LLM Governance
LLM governance is not just about setting policies—it is about building a structured, transparent ecosystem where large language models can operate safely, ethically, and effectively. A well-rounded governance approach ensures that organizations do not just innovate with LLMs but do so responsibly and sustainably. Here are the foundational components every enterprise should consider:
1. Model Lifecycle Management
Managing the entire lifecycle of an LLM—from development and deployment to maintenance and retirement—ensures continuous oversight. This includes version control, performance benchmarking, and tracking changes in model behavior over time. It enables teams to trace decisions back to specific versions, reducing ambiguity and improving accountability during audits or investigations.
2. Responsible Data Sourcing and Usage
Data is the backbone of LLMs, and poor data practices can introduce biases, inaccuracies, or compliance issues. Governance ensures that only legally sourced, representative, and high-quality datasets are used for training and fine-tuning. This includes validating data lineage, handling personal data with care, and avoiding content that could perpetuate stereotypes or misinformation.
3. Risk and Compliance Monitoring
Ongoing risk monitoring helps organizations detect when a model starts to generate harmful, biased, or non-compliant content. A good governance framework includes automated testing, scenario simulations, and red-teaming exercises to flag anomalies and escalate risks. This ensures that LLMs remain within ethical and legal bounds as they evolve in real-world use.
4. Access Controls and Role-Based Permissions
Who can interact with, configure, or modify the model is just as important as what the model can do. Role-based access controls prevent unauthorized changes, safeguard sensitive prompts or data, and reduce the risk of model misuse. Governance ensures that these controls are enforced and regularly reviewed and updated.
5. Prompt Auditing and Output Evaluation
Governance extends to how prompts are designed and how outputs are assessed. Prompt auditing involves reviewing the structure, intent, and results of prompts to ensure they align with business rules and ethical guidelines. Output evaluation, on the other hand, tests whether responses are accurate, consistent, and appropriate for production environments.
6. Stakeholder Alignment and Training
A successful governance model relies on collaboration between technical, legal, operational, and ethical teams. This requires clear roles, shared understanding of model risks, and continuous training. Governance helps establish communication channels and escalation paths, making sure that everyone involved knows how to respond to unexpected behavior or regulatory shifts.
Together, these components form the bedrock of any robust LLM governance framework. They help organizations balance innovation with accountability, allowing them to scale LLM use confidently while minimizing exposure to ethical and operational risks. Without these pillars, even the most sophisticated AI strategy can quickly become unmanageable.
What are Some Core Principles of LLM Governance?
Building a responsible AI ecosystem starts with getting the fundamentals right. While LLMs may feel like a different category of AI, the governance principles that guide them are rooted in well-established ethics and enterprise accountability. These principles are not theoretical—they form the foundation for deploying models that are trusted, compliant, and business-aligned.
Here are the essential principles of LLM governance that every organization must prioritize:
1. Transparency
You cannot govern what you cannot see. Transparency means documenting your model's training data sources, intended use cases, decision pathways, and limitations. It also means making model behavior interpretable to both technical and non-technical stakeholders. Without transparency, it is impossible to detect hallucinations, biases, or misuse—let alone fix them.
2. Accountability
Who is responsible when an LLM makes a harmful decision? Governance demands clarity on ownership and roles—whether data scientists tuning the model, legal teams ensuring compliance, or business leaders setting use policies. Accountability ensures someone is always answerable for the model’s actions, even when it acts autonomously.
3. Fairness and Non-Discrimination
LLMs often reflect and amplify societal biases present in their training data. A solid LLM governance framework must actively test for and mitigate discrimination across age, race, gender, and other protected categories. It is about fairness, trust, brand integrity, and legal compliance.
4. Privacy and Data Security
LLMs process sensitive information, including customer chats, internal documents, and employee data. Effective LLM data governance ensures this data is protected through anonymization, limited access, and detailed audit logs. In highly regulated sectors, maintaining privacy is not just crucial—it is a non-negotiable foundation for trust and compliance.
5. Human-in-the-Loop Oversight
Autonomy does not mean isolation. Human oversight is critical for high-risk or ambiguous scenarios. Whether reviewing LLM outputs in customer service or validating decisions in legal workflows, human-in-the-loop design balances speed with sound judgment.
6. Resilience and Monitoring
LLMs can drift over time—what is safe and accurate today may not be tomorrow. That is why governance must include ongoing monitoring for accuracy, relevance, and safety. Alert systems and feedback loops allow quick course correction before reputational or financial damage occurs.
These principles are not just checkboxes—they are operational guardrails. When embedded into your workflows, they turn LLM governance risk management and compliance into a competitive advantage instead of a compliance burden.
Benefits of Deploying LLM Governance Models
Deploying large language models without a clear governance framework is scaling without proper security. With the right approach, LLM governance does more than reduce risk—it builds trust, increases efficiency, and keeps your AI efforts reliable and defensible.
Here is how organizations are gaining from a mature LLM governance framework:
1. Faster Time to Value
Governance clears the clutter. With defined roles, workflows, and risk thresholds, LLM deployments move from pilot to production without roadblocks. Teams know what is acceptable, what needs review, and where automation fits. This accelerates innovation while maintaining control over risks.
2. Reduced Legal and Compliance Risk
With regulations like GDPR and HIPAA tightening around AI, data governance and compliance are no longer an afterthought. LLM governance helps embed privacy, consent, explainability, and data protection into every stage of the model lifecycle. This proactive approach reduces the risk of violations and costly penalties—well before they become a crisis.
3. Greater Organizational Trust in AI
No model will be trusted if leaders lack visibility into how it works, what it can process, and when it might fail. Governance creates explainability and oversight that build confidence. Whether it is the legal team, marketing, or frontline service reps, everyone feels safe when guardrails are clear.
4. Improved Model Accuracy and Reliability
Governance mandates continuous evaluation—on drift, bias, hallucinations, or performance decay. This loop of continuous monitoring and feedback keeps LLMs aligned with business needs over time. It also reduces the likelihood of rogue outputs that damage brand credibility.
5. Better Customer Experience
An LLM that hallucinates or leaks data kills trust instantly. Strong AI LLM governance ensures outputs are safe, relevant, and privacy-compliant. This ensures reliable and consistent customer interactions without the unpredictability of a black box system.
Each benefit contributes to a broader goal: turning generative AI from a speculative tool into a reliable enterprise asset. With the right governance structure, your LLMs perform better, evolve responsibly, stay compliant, and drive business value at scale.
The Hidden Challenges of Implementing LLM Governance
While the benefits of LLM governance are clear, its implementation comes with a unique set of challenges. If not addressed early, these hurdles can stall enterprise adoption or expose organizations to regulatory and reputational risks. Understanding them is key to crafting a robust and practical governance strategy.
1. Unclear Ownership and Accountability
One of the most common challenges in LLM governance is determining who is accountable for the outputs of a model that constantly evolves. When an LLM generates misinformation or biased results, does accountability fall on the data team, product owner, or AI governance council?
2. Balancing Innovation With Oversight
Excessive governance can create a bottleneck, while insufficient oversight exposes you to risk. Many organizations find it difficult to strike the right balance between enforcing oversight and encouraging innovation. This challenge intensifies with LLMs, whose use cases often span departments—from legal to marketing to customer support.
3. Lack of Standardized LLM Governance Frameworks
There is no one-size-fits-all blueprint for governing LLMs. Most existing AI governance frameworks were built around traditional ML systems and fail to address the nuances of prompt-based learning, model drift, or hallucinations unique to large language models.
Only 26 percent of organizations have fully integrated generative AI standards into their governance frameworks. This gap indicates that there is still a significant scope in terms of developing LLM specific governance standards. (Source: Webpronews)
4. Model Transparency and Explainability
LLMs, by design, are complex and opaque. Unlike traditional AI models that work on structured data and clear features, LLMs derive outcomes from vast and often ambiguous training sets. This makes explainability—a core pillar of governance—incredibly challenging.
5. Data Privacy and IP Protection
LLMs trained on vast internet-scale corpora often come with risks around intellectual property and personal data exposure. Without governance guardrails, enterprises risk violating privacy laws like GDPR or inadvertently reproducing copyrighted content in model outputs.
Mitigating these risks requires a well-defined governance strategy that ensures LLM remains compliant and ethically sound.
Exploring Applications of LLM Governance
While much of the conversation around LLM governance focuses on risk mitigation, its true value unfolds in enabling responsible, secure, and scalable AI applications. As LLMs power mission-critical workflows, LLM governance and LLM evaluation transform from a back-office checklist into a business enabler.
Here are some real-world application areas where LLM governance plays a pivotal role:
1. Customer Service Automation
Enterprises are using LLMs to power intelligent agents, chatbots, and support tools—delivering faster resolution and improved customer experiences. Without governance, these systems can hallucinate, respond inappropriately, or expose private data.
What if the LLM assistant returns private information of other customers like phone numbers, addresses when asked about it? That is a clear violation of data privacy law.
LLM governance frameworks ensure role-based access, prompt controls, red-teaming of outputs, and human-in-the-loop escalation paths. This ensures that bots remain helpful, non-biased, and compliant across every interaction.
2. Internal Knowledge Management
LLMs like Chat GPT and Gemini are being used to sift through internal documents, knowledge bases, and emails to answer employee queries, boosting productivity. However, without access governance and content filtering, there is a risk of exposing sensitive IP or HR data.
Governance layers like document-level access control, real-time monitoring, and audit trails keep enterprise knowledge flows secure, helping organizations safely scale their internal copilots.
3. Automated Content Generation
Marketing, legal, and product teams increasingly use LLMs to draft documents, blogs, contracts, or training material. Governance is critical here to ensure factual accuracy, brand voice consistency, and legal safety.
A governed LLM workflow includes prompt testing, plagiarism checks, tone/style calibration, human review stages, and secure publishing—ensuring generated content meets enterprise standards every time.
4. Decision Support in Regulated Industries
In sectors like healthcare, finance, and insurance, LLMs are being used to summarize reports, generate treatment options, or provide underwriting support. The risks here go beyond brand; they involve legal liability, patient safety, and market impact.
LLM governance frameworks enforce role restrictions, explainability protocols, confidence scoring, and audit logs—enabling safe augmentation of critical decisions without crossing compliance lines.
5. Code Generation and DevOps
Developers use LLMs to write boilerplate code, generate test cases, and recommend improvements. Without proper controls, this can lead to insecure code, licensing issues, or embedded bias.
Governed AI systems validate generated code against enterprise security policies, flag deprecated functions, and ensure open-source compliance—turning LLMs into safe, productive coding partners.
6. Risk Management and Fraud Detection
LLMs trained on historical incidents and transactional patterns can help spot anomalous behavior, trigger alerts, and suggest mitigation actions. However, accuracy and bias are constant threats.
Governance ensures LLMs do not learn from false positives or discriminatory patterns and integrates model outputs with human reviews for final decisions, reducing reputational and legal risk.
Across use cases, AI LLM governance acts as the invisible scaffolding, enabling enterprises to move fast without breaking things. This turns experimentation into execution, pilots into platforms, and AI into lasting value.
Real-World Examples of LLM Governance
As organizations deploy LLMs at scale, ensuring compliance with ethical and regulatory standards has become a top priority. Companies and governments are implementing llm governance risk management and compliance frameworks to mitigate risks related to bias, misinformation, and security vulnerabilities
EU AI Act Checker Finds Compliance Gaps in Big Tech’s AI Models
The EU AI Act Checker, developed by Swiss startup LatticeFlow in collaboration with researchers from ETH Zurich and INSAIT, has exposed significant compliance gaps in major AI models. Designed to align with the EU’s AI Act, the tool tested generative AI models from companies like Meta, OpenAI, and Alibaba across multiple categories, awarding scores from zero to one.
Key findings highlight deficiencies in cybersecurity resilience and discriminatory output, two critical areas of regulatory concern. OpenAI’s GPT-3.5 Turbo received a low 0.46 score for discriminatory output, while Alibaba Cloud’s Qwen1.5 72B Chat scored 0.37. In cybersecurity tests for prompt hijacking, Meta’s Llama 2 13B Chat scored 0.42, and Mistral’s 8x7B Instruct scored 0.38. The highest-performing model, Anthropic’s Claude 3 Opus, achieved 0.89 overall.
With the EU AI Act set to take effect in phases over the next two years, these results provide early indicators of compliance risks. LatticeFlow’s CEO, Petar Tsankov, emphasized that while the results are generally positive, they underscore the need for AI developers to fine-tune models for compliance. The LLM Checker will be freely available for developers to assess their models, supporting proactive alignment with regulatory requirements. (Source: Business Standard)
Anthropic’s AI Constitution: A Blueprint for Ethical and Transparent AI
Anthropic has introduced a groundbreaking AI constitution for its conversational model, Claude, to promote ethical AI behavior. This constitution is built on widely accepted principles, drawing from sources like the UN Declaration of Human Rights, AI ethics research, and digital platform policies. It sets guidelines for helpfulness, honesty, and harm reduction, ensuring that Claude handles sensitive topics responsibly.
The constitution was developed through extensive research, trials, and collaboration among Anthropic’s AI scientists, policy experts, and operational leaders. It helps mitigate bias, misinformation, and security risks as well as serves as a model for other AI developers aiming for greater transparency and accountability.
Anthropic emphasizes that this constitution is evolving—open to research and public feedback, reflecting the company’s commitment to adaptable and democratic AI governance. While not a complete solution to AI ethics, this approach establishes clear value systems within AI models, making them more transparent and steerable.
As AI becomes more autonomous, Constitutional AI offers a promising path toward aligning models with human ethics and societal norms, moving beyond optimization for narrow tasks. Anthropic’s approach signals a shift toward responsible AI development, urging industry-wide collaboration to refine ethical AI frameworks. (Source: Venturebeat)
These real-world implementations highlight the growing emphasis on LLM governance risk management and compliance, proving that proactive oversight and structured frameworks are essential for ensuring AI models operate responsibly, ethically, and in alignment with societal expectations.
LLM Governance is the Bridge Between Innovation and Accountability
Large language models rapidly shape how businesses operate—from enhancing customer service to reimagining internal workflows. However, the real impact does not come from building fast but from building right. Without clear governance, even the most promising Gen AI initiatives risk becoming unscalable, untrustworthy, and non-compliant.
That is why LLM & AI governance matters. It turns complex AI systems into accountable, transparent, and repeatable tools that business leaders can rely on. When done well, it is not a blocker—it is an accelerator that helps you move faster with fewer surprises.
At Tredence, we help enterprises do just that. With deep experience across industries and a portfolio of production-ready Gen AI solutions, we design governance-aware LLM applications that deliver value—safely and at scale.
Our Gen AI solutions span various use cases, building an organization assistant from your knowledge base using KNOWDY, data analytics and assistant Analytics CoPilot, coding assistant GENFLOW and building a LLMOps CoE for your enterprise are some of our offerings
Whether you are building a customer-facing agent or a domain-specific copilot, we partner with you to ensure your Gen AI investments stand up to scrutiny and deliver measurable results.
Let us make your Gen AI ambitions resilient, responsible, and real. Talk to Tredence experts to see how we can help you govern with clarity and scale with confidence.
FAQs
Q1: How do companies ensure compliance when using LLMs?
To ensure compliance, companies embed regulatory checks directly into the LLM lifecycle. This includes aligning model training and usage with data privacy laws like GDPR or HIPAA, setting clear consent and retention policies, and maintaining auditable records. A strong LLM governance framework standardizes these practices across teams, reducing the risk of non-compliance and enabling proactive oversight.
Q2: What role does transparency play in LLM governance?
Transparency is central to LLM governance. It ensures stakeholders understand how a model makes decisions, what data it is trained on, and how risks are monitored. Transparent documentation and explainability protocols foster trust, simplify audits, and make it easier to troubleshoot bias or unexpected outputs—especially in regulated industries.
Q3: Can LLM governance improve data privacy and security?
Yes, LLM governance significantly strengthens data privacy and security. It enforces safeguards like data anonymization, role-based access, and real-time monitoring to prevent sensitive information exposure. By treating data protection as a continuous discipline—not a one-time control—governance helps organizations manage risk while keeping AI deployments resilient and compliant.
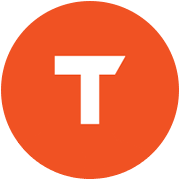
AUTHOR - FOLLOW
Editorial Team
Tredence