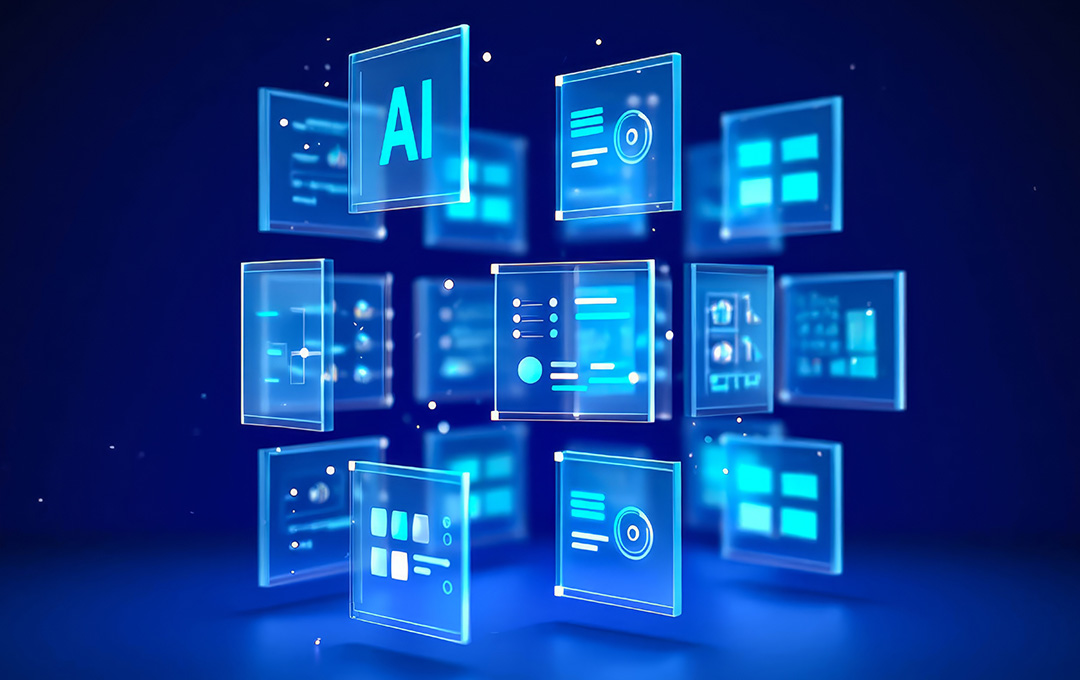
Imagine hiring a team of specialists who never sleep, never complain, and get smarter every week. One handles customer support, another manages your finances, and another writes code. They don't ask for raises. They just ask for clean data.
This is what AI agents have become in 2025: not just tools, but tireless digital teammates embedded across your organization. They handle the repetitive, routine tasks so your people can focus on what humans do best: strategy, creativity, and decision-making.
The shift is already happening. A recent LangChain survey of over 1,300 professionals revealed that performance quality, not cost or safety, is the top concern for companies deploying AI agents. (Source: LangChain) For small businesses, it matters twice as much as cost. This tells us something important: AI agents are no longer experiments. They're operational assets expected to deliver real value.
In this article, we'll explore what AI agents actually do, profile the best AI agents in 2025, show how leading companies are deploying them, and share insider tips to help you stay ahead. Whether you're automating sales, service, or code, the age of intelligent delegation has arrived and is transforming how businesses run.
Before evaluating specific AI agent solutions, it's critical to understand the fundamental architecture that powers these systems and how their continuous perception-decision-action cycle delivers value across business processes.
Understanding AI Agents
An AI agent is an autonomous system that observes its environment, analyzes inputs, and takes action without human intervention. Unlike traditional AI tools, agents operate in a continuous perception–decision–action loop to perform tasks proactively.
What sets the best AI agents apart isn't just that they automate. It's that they adapt. They learn from feedback, context, and evolving data, making them smarter and more reliable over time. They're not rigid scripts. They're responsive systems built for real-world complexity.
With a clear understanding of AI agent functionality, let's examine the most sophisticated enterprise solutions available today, each designed to address specific business challenges across industries and functional domains.
Top 10 AI Agents in 2025
The AI agent landscape has evolved dramatically, with solutions now available for virtually every business function. These aren't simple chatbots or passive assistants. They're autonomous systems that observe, analyze, and act without constant human direction.
Here's our analysis of the ten most powerful and best AI agents in the world that are transforming business operations in 2025:
-
Oracle's Miracle Agent: Oracle's Miracle Agent is embedded within its Fusion Cloud suite, designed to automate workflows across finance, HR, and supply chain. It can process structured and unstructured data, trigger tasks, and handle approvals end-to-end without manual intervention.
- Strength: End-to-end automation for finance, HR, and supply chain
- Ideal for: Enterprises using Oracle ERP
-
Microsoft's Copilot Vision Agents: These agents live inside Microsoft Dynamics 365 and Microsoft 365. Unlike passive assistants, they take full ownership of tasks—updating CRM records, managing service workflows, and executing commands across apps.
- Strength: Task execution across sales, finance, and service
- Bonus: Copilot Studio lets you build custom agents for any workflow
-
Anthropic's Claude 3.5: Claude 3.5 mimics human behavior in digital environments. It can browse websites, click buttons, log into systems, and retrieve information just like a human navigating a desktop or web app.
- Strength: Human-mimicking behavior for desktop automation
- Ideal for: Enterprises automating internal tooling.
-
NVIDIA's Eureka: Eureka is a GPT-4-powered AI agent built for robotics. Instead of assisting humans, it teaches robots new tasks using reinforcement learning—watching performance, providing feedback, and helping machines improve through trial and error.
- Strength: Autonomous robot training
- Ideal for: Manufacturing and logistics firms using robotics.
-
SAP's Joule: Joule is SAP's built-in AI agent that works across its enterprise software suite. It combines business data with LLMs like Llama 3 to surface insights, flag anomalies, and recommend corrective actions across HR, finance, and operations.
- Strength: Collaborative intelligence across enterprise functions
- Ideal for: SAP-driven enterprise ecosystems
-
Replit Agent: Replit Agent turns plain-language prompts into working software. It can scaffold codebases, fix bugs, write documentation, and even deploy basic applications, enabling rapid prototyping without a dedicated engineering team.
- Strength: End-to-end development support
- Ideal for: Startups and product teams
-
OpenAI's Operator: Operator is designed to move beyond static prompts. It works across software interfaces to carry out complex, multi-step workflows like booking travel, writing reports, or completing transactions without needing constant hand-holding.
- Strength: Task automation across software
- Bonus: Early use cases include travel, coding, and research
-
Salesforce Agentforce 2.0: Agentforce provides prebuilt AI agents that act as virtual sales reps, support agents, or service personnel. These agents run inside Salesforce, leveraging CRM and Data Cloud integrations to automate frontline tasks.
- Strength: Role-based agents, deep CRM integration
- Ideal for: Sales and customer service teams
-
Fujitsu's Kozuchi: Kozuchi is a strategic decision-making agent. It synthesizes structured data, legal documents, and regulatory frameworks to help executives make better calls under uncertainty, especially in compliance-heavy environments.
- Strength: Executive-level decision support
- Ideal for: Legal, strategic, and regulatory teams
-
AutoGPT: AutoGPT is a framework, not a finished product. It lets developers build their own autonomous agents that execute long chains of logic like performing research, interacting with APIs, and handling multi-step tasks across applications.
- Strength: Extreme customization
- Ideal for: Tech-savvy teams and internal innovation labs
While understanding the capabilities of individual agent platforms is valuable, examining real-world implementations reveals how market leaders are integrating the best AI agents into their operational strategies to drive measurable business results.
How Leading Companies are Using AI Agents to Stay Ahead
AI agents are no longer experimental. They're operational. Companies across industries are deploying them to scale workflows, reduce manual effort, and drive faster decisions with higher accuracy. Here's how Amazon, Sephora, and Tredence are putting some of the best AI agents to work right now.
Amazon
Amazon uses generative AI agents to enhance multiple touchpoints in the customer journey.
- It recently rolled out AI-generated review summaries, helping shoppers quickly grasp product pros and cons without reading hundreds of reviews. These summaries appear directly on product pages, drawing from verified customer feedback. (Source: Amazon)
- Its new AI-powered shopping assistant, Rufus, answers customer queries, compares products, and suggests options based on search context. Rufus is trained on Amazon's product catalog and user reviews to provide personalized guidance. (Source: Amazon)
- In physical retail, Just Walk Out technology combines sensors and AI agents to automatically detect products taken from shelves and bill users directly without requiring checkout. (Source: Amazon)
These agents aren't just reactive. They're redefining how customers browse, choose, and buy across channels.
Sephora
Sephora has deployed AI agents to bridge online and in-store customer experiences.
- Its virtual assistant, launched across Singapore and Malaysia, handled over 330,000 customer conversations in its first year. It offers personalized product recommendations, beauty advice, and supports product discovery across channels. This rollout contributed to an estimated $30,000 in additional monthly revenue. (Source: etailasisa)
- The assistant integrates seamlessly with Sephora's loyalty program and ecommerce platform, ensuring context-aware responses.
- In parallel, Sephora uses AI agents to enhance product tagging and personalization, helping users find the right shade, formulation, or routine in seconds.
These agents are not only improving conversion rates but also making the shopping experience feel like a one-on-one consultation at scale.
Tredence
Tredence works with enterprises to build custom AI agents that solve deeply embedded business challenges.
- For a Fortune 100 tech firm, Tredence created Word Craft, an AI content engine that personalizes messages across 100+ languages and platforms. Alongside this, ABI (an A/B testing agent) and Customer Lifecycle Manager were deployed to orchestrate omnichannel experiences.
- For a global payments company, Tredence built an AI/ML deduplication agent to clean millions of incoming leads from multiple sources, improving marketing efficiency and prioritization.
- For a distributor managing over 3 million SKUs, Tredence implemented a product classification agent that categorized products using machine learning, enhancing user search experience and minimizing lost sales.
These use cases reflect the practical power of AI agents, not just as digital workers, but as decision-making enablers that evolve with the business.
Successfully deploying AI agents requires more than selecting the right technology—it demands a structured approach to implementation, data governance, and performance management to maximize returns while minimizing operational risk.
Insider Tips to Making the Most Out of AI Agents
If you're investing in the best AI agents, it's not just about plugging them in and letting them run. The difference between a high-performing agent and one that causes more problems than it solves lies in how you deploy, monitor, and evolve it.
Here are five non-negotiables to get it right:
1. Start with narrow use cases
AI agents perform best when they're deployed in focused, high-clarity environments. Don't expect them to handle a dozen different workflows from day one.
How to do it:
- Begin with tasks that are repeatable and well-defined such as meeting transcription, customer FAQ handling, or lead scoring.
- Choose areas where outcomes can be clearly measured, like reduction in ticket response time or accuracy of invoice processing.
- Once the agent proves consistent in these narrow domains, you can gradually expand its scope into adjacent processes.
Starting small builds confidence, reveals edge cases early, and gives you time to build internal expertise.
2. Ensure data accuracy
AI agents are only as smart as the data they receive. Poor data quality leads to flawed decisions, redundant work, or worse—customer-facing errors.
How to do it:
- Audit your data sources for completeness, consistency, and timeliness before deployment.
- Use data catalogs and governance tools to standardize definitions and relationships across systems.
- Ensure agents have access to live, real-time data wherever possible, especially in customer-facing scenarios.
- Avoid feeding agents from spreadsheets or fragmented systems unless absolutely necessary.
Clean data is not a nice-to-have. It's the foundation the agent builds all its decisions on.
3. Set clear rules for escalation
Not every situation should be handled by an agent. In sensitive scenarios like customer complaints, financial anomalies, or regulatory alerts, agents must know when to stop and escalate.
How to do it:
- Define clear thresholds or trigger conditions that require human intervention. For example: "Escalate any customer ticket with more than two failed resolution attempts."
- Set up role-based fallbacks. If the agent reaches a dead end, who should take over, and how?
- Ensure escalation workflows are logged and traceable, so nothing slips through.
- Build this logic into the agent's design, not as an afterthought, but as a safety mechanism from day one.
This balances speed with control and builds trust across internal teams.
4. Test in sandbox environments
Even the smartest AI agents need time to learn your data, your edge cases, and your operating rhythm. Rushing into production is a recipe for errors.
How to do it:
- Run the agent in a staging environment that mirrors real systems but without the risk.
- Use synthetic or historical data to simulate interactions and measure response accuracy.
- Set up A/B tests. One workflow run by humans, the other by the agent, and compare outcomes.
- Bring in actual users to observe agent behavior and flag unexpected outputs.
Treat it like hiring a new employee: you wouldn't give them full autonomy on Day 1, so don't give it to your agent either.
5. Monitor continuously
AI agents are not set-and-forget. They evolve based on new data and context, which means performance can drift if left unchecked.
How to do it:
- Build dashboards that track agent performance over time: accuracy, speed, errors, and escalations.
- Set up alert systems that flag anomalies, such as sudden spikes in incorrect responses or dropped tasks.
- Schedule monthly reviews where business and tech teams analyze agent behavior, review edge cases, and fine-tune parameters.
- Encourage frontline users to submit feedback on agent performance. It's often the fastest path to spot issues.
Continuous monitoring keeps the agent aligned with business goals and gives you an early warning when things start to go off-track.
As organizations master current AI agent capabilities, understanding the upcoming evolution of this technology will be essential for building scalable, future-proof strategies that deliver sustained competitive advantage.
From Now to Next for AI Agents
2025 is just the beginning. Today's AI agents are capable, but what comes next is transformational. The next generation won't just execute instructions. They'll understand context, learn from behavior, and drive decisions proactively.
The companies preparing for this shift now will gain a compounding advantage because tomorrow's agents will only be as good as the foundations laid today.
What the future of AI agents could look like:
Imagine a business environment where AI agents anticipate needs before they're articulated. A CFO receives instant cash flow risk analysis for supply chain disruptions; customer support agents proactively identify product issues and recommend solutions; R&D teams benefit from automated analysis of lab results; and organization-wide, voice-first agents manage meetings, decisions, and follow-ups while adapting to individual work styles.
Realizing this potential demands more than technology. It requires a strategic partner with domain expertise and enterprise implementation experience to transform AI capabilities into measurable business outcomes.
Turn AI Agents into Real Impact with Tredence
AI agents are reshaping how businesses operate, from automating daily tasks to enabling strategic, cross-functional decision-making. But success doesn't come from simply adopting the latest tools. It depends on having the right data foundations, clear use cases, and a partner who can turn intelligent automation into real business outcomes.
That's where Tredence comes in.
Tredence brings deep expertise in building, customizing, and deploying AI agents across complex enterprise ecosystems. From designing multimodal agents that understand your data and workflows to integrating them with systems like Salesforce, SAP, and internal platforms, Tredence helps organizations move from pilot projects to production-scale impact.
Whether it's an agent that personalizes digital experiences across 100+ languages, deduplicates millions of marketing leads, or auto-classifies SKUs in a 3-million product catalog, Tredence delivers AI agents that don't just automate but accelerate growth.
If you're planning your first deployment or scaling across business units, Tredence helps you define the right use cases, operationalize AI responsibly, and drive measurable ROI quickly and reliably.
Explore how Tredence can help you deploy the best AI agents for your business. Contact us today.
FAQs
1. What criteria should businesses consider when selecting an AI agent?
Businesses should evaluate AI agents based on task specificity, integration capabilities with existing systems, data requirements and security, scalability potential, and governance features, including monitoring and escalation mechanisms. The best AI agent solutions are purpose-built for specific use cases rather than general-purpose tools.
2. What main limitations do current AI agents have?
Current AI agents primarily struggle with contextual understanding, often missing nuance in complex business scenarios. They require extensive training data, typically operate in silos rather than across systems, and remain largely reactive instead of proactive. Most agents need explicit instructions rather than identifying automation opportunities independently.
3. What role do AI agents play in conversational AI?
AI agents execute the actions requested through conversational interfaces. They transform natural language inputs into concrete tasks, such as scheduling appointments, processing transactions, or retrieving information across systems. While conversational interfaces handle user interactions, AI agents perform the actual work that fulfills user requests.
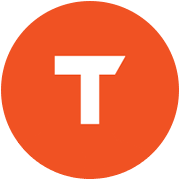
AUTHOR - FOLLOW
Editorial Team
Tredence