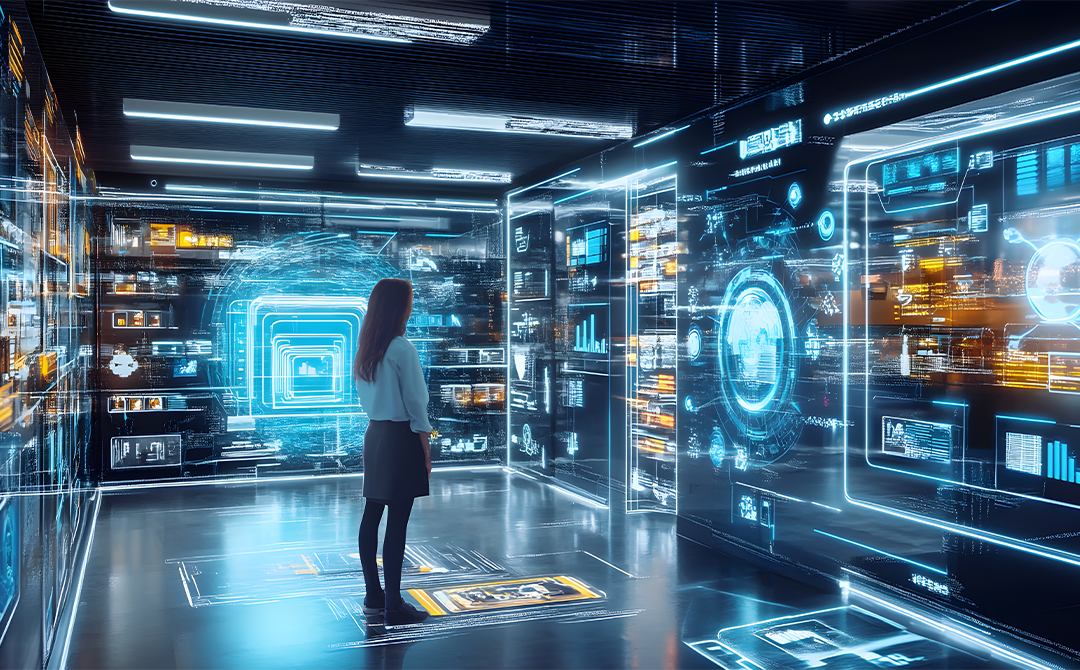
Imagine this: On a Monday morning, a merchandiser at a fashion retail chain logs in, expecting routine reports. Instead, Agentic AI in retail has already flagged a decline in denim sales for a specific region. But it does not stop at detection—it has identified the cause, such as delayed influencer campaigns and competitor discounts, tested counter strategies overnight, and launched a geo-targeted promotion via email and mobile. Inventory levels have also been adjusted based on projected demand. This isn’t just automation; AI is acting autonomously to keep retailers ahead of shifting market dynamics.
Retail has always been about being in the right place, at the right time, with the right offer. But today, what is “right” changes by the minute—driven by shifting demand, new channels, and customers who expect experiences to be instant and personalized.
Globally, retailers are recognizing the potential of AI to enhance customer experiences, optimize operations, and drive profitability. For instance, during the recent Black Friday sales, U.S. e-commerce revenue reached $10.8 billion, a 10.2 percent increase from the previous year. AI-powered chatbots contributed to a 1,800 percent surge in retail site traffic. (Source: Yahoo Finance)
What is Agentic AI, and Why is Everyone in Retail Talking About it?
Agentic AI refers to autonomous AI systems capable of independent decision-making and action, mimicking human cognitive functions such as sensing, reasoning, and acting. In the retail sector, this translates to AI applications that can dynamically adjust pricing, manage inventory levels, personalize marketing strategies, and place orders—finding the best offers on preferred brands and styles—without direct human intervention.
Why is this gaining traction now? A few factors are:
- Increasing Retail Complexity: From omnichannel fulfillment to dynamic pricing, the demand exceeds what humans can manage manually.
- Massive Data and Delayed Insights: While data is everywhere, insights often arrive late. Agentic AI shortens the loop between sensing and acting.
- Maturing AI Tools: Advances in large language models, edge computing, and reinforcement learning make AI tools more capable.
The impact of AI on consumer behavior is also noteworthy. In the UK, for instance, retail traffic from AI sources increased tenfold between July and September 2024, with approximately 30 percent of Britons using AI for online shopping, showcasing consumers’ readiness and willingness to leverage AI as their shopping assistant. (Source: TimesUK)
These trends highlight the potential of Agentic AI in retail, offering opportunities for enhanced efficiency, customer satisfaction, and profitability.
The Impact of Agentic AI in Retail: What Your Business Could Be Missing
Let us face it—traditional retail automation has significantly advanced but still relies heavily on static rules and human-triggered workflows. If your retail systems need someone to push a button every time conditions change, you are already falling behind competitors embracing Agentic AI.
Here is what you risk missing without Agentic AI in retail:
What You are Missing |
What Happens Without Agentic AI |
What Agentic AI Enables |
Real-Time Responsiveness |
Adapting to market shifts, inventory changes, or customer behavior takes hours or days. |
Autonomous agents respond in seconds across channels without requiring manual triggers. |
Personalization at Scale |
Static segmentation cannot deliver the one-to-one experiences customers expect. |
Hyper-personalized interactions in real time, tailored to each customer. |
Operational Efficiency |
Teams spend time making repetitive decisions and chasing anomalies manually. |
Agents monitor, detect, and act on issues instantly—freeing teams to focus on strategy. |
Revenue Opportunities |
A slow response to trends means missed chances to restock, promote, or optimize offers. |
AI agents act proactively to capitalize on sales triggers at the moment. |
Frictionless Customer Journeys |
Inconsistent messaging and delays erode trust and reduce retention. |
Seamless, context-aware experiences that adapt in real time to each shopper’s journey. |
To stay competitive in a high-speed, high-expectation environment, retail systems need to think, act, and adapt without always asking for permission. Agentic AI enables this in retail.
Understanding How Agentic AI Works
Agentic AI is not just another layer on top of existing AI systems—it requires a purpose-built architecture that enables autonomous decision-making and action. Unlike traditional AI setups focusing on predictions or classifications, agentic systems are designed to continuously sense, reason, decide, and act with minimal human oversight.
Here are the key components of the architecture of Agentic AI in retail:
1. Sensing Layer (Data Ingestion and Contextual Awareness)
This is where the agent gathers data from internal and external sources:
- Transaction and POS systems
- Inventory databases
- Customer interactions (online/offline)
- Market signals (social media, weather, competitor pricing)
This layer creates a real-time, contextual picture of the retail environment.
2. Reasoning Engine (Cognitive Processing)
At the heart of the architecture is a reasoning layer that mimics decision logic:
- It applies business rules, AI models—such as demand forecasting and customer segmentation—and optimization frameworks to simulate outcomes.
- Instead of just flagging insights, it evaluates trade-offs and chooses optimal actions based on predefined goals.
3. Memory and Learning Module
Agents need to remember outcomes and learn over time:
- This module stores interaction history, actions taken, and performance feedback.
- It enables continuous improvement, making agents smarter and more effective with each cycle.
4. Action Layer (Autonomous Execution)
Once a decision is made, agents act:
- They can launch a promotion, reprice products, trigger replenishments, or send customer messages without human approval.
- Integration with ERP, CRM, and eCommerce platforms is critical for a seamless execution.
5. Guardrails and Governance
Autonomy requires responsibility. This layer ensures:
- Ethical boundaries, including fair pricing and data privacy
- Business constraints, such as preventing over-discounting or violating vendor agreements
- Escalation protocols for decisions exceeding scope or confidence levels
6. Human Feedback Loop
While agents act independently, they are not black boxes. A feedback interface allows:
- Business users to monitor decisions
- Adjust goals and constraints
- Step in for override or course correction when needed
A well-designed Agentic AI architecture changes retail operations from reactive to proactive. It enables systems to interpret real-world signals, make high-stakes decisions, and execute them instantly—enabling teams to focus on complex tasks.
Breaking Barriers: Overcoming Retail’s Biggest Challenges with Agentic
In recent years, retailers have relied on AI for demand forecasting, personalized recommendations, and inventory management. However, traditional AI models operate within predefined rules, requiring manual intervention to act on insights. Agentic AI in retail goes beyond static predictions—it autonomously makes decisions, adapts to real-time changes, and takes proactive actions. Without this level of intelligence, retailers struggle to keep pace with evolving consumer behavior and dynamic market conditions. Yet, implementing Agentic AI comes with hurdles.
Major Operational and Technological Roadblocks in Adoption
- Legacy System Integration: Many retailers still operate on outdated infrastructure not designed for AI-driven automation. These legacy systems often lack APIs or seamless connectivity, making integrating Agentic AI without costly upgrades difficult. Without a unified data architecture, AI agents cannot access the full spectrum of information needed for decision-making.
- Data Silos and Quality Issues: Retailers collect vast amounts of data across inventory, sales, customer interactions, and supply chains, but much of it remains trapped in silos. Disparate systems prevent AI from gaining a holistic view, reducing its ability to generate accurate insights. Additionally, poor data quality—such as missing, inconsistent, or outdated records—undermines the effectiveness of AI-driven automation.
- Scalability and Performance: Implementing Agentic AI requires computing power and infrastructure to handle real-time decision-making at scale. Many retailers struggle with cloud migration, bandwidth constraints, or the high costs of AI training and model deployment. AI adoption remains limited to small-scale pilots rather than full-fledged implementations without scalable solutions.
- Balancing Automation with Human Oversight: While Agentic AI can autonomously execute decisions, retailers must ensure it aligns with business goals and ethical guidelines. Over-reliance on AI without human validation can lead to unintended outcomes, such as pricing errors or misaligned promotions. Establishing governance frameworks and approval mechanisms is essential for responsible AI adoption.
- Workforce Readiness and Change Management: AI-driven automation alters traditional retail workflows, requiring employees to adapt to new working methods. Resistance to change, lack of technical skills, and concerns over job displacement can slow adoption. Retailers must invest in AI literacy programs and ensure employees see AI as an enabler rather than a replacement.
- Security, Privacy, and Compliance: Retail AI systems process sensitive customer data, making them targets for cyber threats and regulatory scrutiny. Ensuring AI models comply with data protection laws, such as GDPR or CCPA, while maintaining robust cybersecurity measures is critical. Without proper safeguards, AI adoption can expose retailers to financial and reputational risks.
Despite these barriers, retailers that successfully implement Agentic AI unlock new levels of efficiency, agility, and customer engagement. The key lies in understanding how to mitigate risks and adopt best practices. In the next section, we will explore how we overcome these barriers.
Unlocking Competitive Advantage: Expert Strategies for Agentic AI Success in Retail
Agentic AI in retail implementation often faces significant operational and technological hurdles that can slow implementation. Overcoming these challenges requires a well-defined approach that ensures seamless integration, data accessibility, scalability, and responsible AI governance.
Legacy System Integration
Many retailers operate on legacy infrastructure that lacks the flexibility for AI-driven automation. To overcome this, businesses can invest in middleware solutions, cloud-based integrations, or API layers that enable seamless connectivity without replacing entire systems. A gradual modernization approach ensures AI agents can access critical data while minimizing operational disruptions.
Data Silos and Quality Issues
Retailers must establish a unified data architecture to break down silos across inventory, sales, and customer interactions. Implementing real-time data validation, automated cleansing, and standardized data governance ensures AI agents have reliable, comprehensive information for accurate decision-making. Cloud data lakes and AI-powered ETL (Extract, Transform, Load) processes help streamline data accessibility.
Scalability and Performance
Ensuring AI models can operate at scale requires a strong computing infrastructure with cloud or edge computing capabilities. Retailers should optimize AI models for efficiency, leverage distributed computing, and prioritize cost-effective AI deployment strategies. Investing in scalable machine learning platforms prevents AI adoption from being confined to small-scale pilots.
Balancing Automation with Human Oversight
Retailers should implement AI governance frameworks that define decision-making thresholds requiring human approval. Role-based access controls, real-time monitoring dashboards, and AI explainability tools help ensure AI-driven actions align with business goals and ethical considerations. Regular audits of AI decisions can further enhance reliability.
Workforce Readiness and Change Management
AI adoption requires upskilling employees and fostering a culture that views automation as an enabler rather than a threat. Retailers should launch AI literacy programs, provide hands-on training for AI-assisted workflows, and involve employees in the transition process. Clear communication about AI’s role in augmenting—not replacing—human expertise helps mitigate resistance.
Security, Privacy, and Compliance
AI-driven retail operations must comply with data protection regulations like GDPR and CCPA while maintaining robust cybersecurity measures. Implementing AI-specific security protocols, encryption, and anomaly detection systems helps prevent data breaches. Retailers should conduct regular compliance audits to ensure AI solutions adhere to evolving regulatory standards.
By proactively addressing these challenges, retailers can ensure a smooth, scalable, and responsible adoption of Agentic AI, unlocking its full potential to drive efficiency and competitive advantage.
KPIs That Would Help You Track How Your AgenticAIi Is Performing Better:
How do you measure whether your Agentic AI implementation yields positive results for your business, and how do you determine in what areas your Agentic solution must be refined further? Here’s a comprehensive list of KPIs that would help you judge and monitor your agentic AI in retail performance:
1. Revenue Uplift & Conversion Rates
- KPIs: Revenue per visitor (RPV), conversion rate (CR), average order value (AOV).
- How to Measure: Track RPV and CR before and after AI-driven personalization is implemented. Use A/B testing to compare AI-powered recommendations versus static experiences.
- Why It Matters: A higher conversion rate indicates that AI effectively guides customers toward purchases, while an increase in AOV suggests better upselling and cross-selling strategies.
2. Customer Retention & Engagement
- KPIs: Customer lifetime value (CLV), repeat purchase rate, churn rate, engagement score.
- How to Measure: Use predictive models to estimate CLV based on past purchases and engagement frequency. Track the percentage of customers making repeat purchases and analyze churn trends.
- Why It Matters: Improved engagement and retention mean, AI is delivering relevant, timely recommendations, increasing customer loyalty and reducing acquisition costs.
3. Operational Efficiency Gains
- KPIs: Order fulfillment time, automation rate, employee productivity.
- How to Measure: Track the percentage of tasks automated by AI (e.g., chatbots handling customer inquiries or AI-driven inventory management). Measure fulfillment times and reductions in manual workload.
- Why It Matters: AI should streamline operations, allowing human employees to focus on higher-value tasks while reducing fulfillment delays.
4. Inventory Optimization & Waste Reduction
- KPIs: Stock turnover ratio, demand forecast accuracy, markdown rate.
- How to Measure: Compare AI-driven demand forecasts with actual sales data to assess prediction accuracy. Monitor stock turnover and track unsold inventory requiring markdowns.
- Why It Matters: AI should improve stock efficiency by reducing overstock and understock scenarios, leading to lower holding costs and higher profitability.
5. AI-Driven Decision Accuracy
- KPIs: Recommendation acceptance rate, pricing accuracy, demand prediction error (Mean Absolute Percentage Error - MAPE).
- How to Measure: Track how often customers accept AI-driven recommendations. Assess pricing models against market fluctuations. Use MAPE to evaluate AI’s ability to predict demand.
- Why It Matters: Higher accuracy ensures AI makes well-informed decisions, reducing costly pricing errors and inventory mismatches.
By proactively addressing these challenges and measuring these KPIs, retailers can ensure a smooth, scalable, and responsible adoption of Agentic AI, allowing it to reach its full potential to drive efficiency.
Agentic AI Use Cases in Retail That Will Inspire Your Next Move
Agentic AI is already making waves across retail—from streamlining operations to elevating customer experiences. Here are six compelling examples of Agentic AI in retail examples showing how autonomous agents can make an impact on daily business operations for the retail industry:
1. Autonomous Customer Support Agents
Agentic AI can power intelligent virtual agents that go beyond answering FAQs. These systems learn from every interaction, update their knowledge base automatically, and escalate to human agents only when necessary. They can handle queries regarding order tracking, returns, product availability, and even size recommendations 24/7.
By 2029, Agentic AI is expected to handle 80 percent of routine customer service inquiries, lowering operational costs by up to 30 percent through reduced reliance on human intervention. (Source: Gartner)
Business Benefit: Reduced customer service costs and higher resolution rates.
Customer Benefit: Faster, more consistent support with minimal wait times.
Operational Benefit: Enables human agents to handle complex issues, improving workforce efficiency.
2. Dynamic Pricing and Promotion Agents
In real time, these AI agents autonomously monitor product demand, competitor pricing, inventory levels, and customer behavior. Based on this data, they dynamically adjust prices and promotional offers—online or at the point of sale.
Business Benefit: Maximizes revenue and margin by optimizing pricing in response to market conditions.
Customer Benefit: Relevant offers and personalized discounts.
Operational Benefit: Reduces manual intervention in markdown planning and accelerates decision-making cycles.
3. Smart Inventory Replenishment Agents
Agentic AI systems predict product demand with higher accuracy at the SKU and store level, automate stock reordering, and reroute inventory based on real-time sales velocity and external factors such as weather or local events.
Business Benefit: Reduces stockouts and overstocking, directly impacting revenue and profitability.
Customer Benefit: Ensures product availability when and where needed.
Operational Benefit: Streamlines supply chain and warehouse operations by reducing manual planning and logistics inefficiencies.
4. AI-Powered Merchandising Agents
These agents continuously analyze shopper behavior, in-store movement (via IoT or sensors), and digital engagement to optimize product placement, signage, and assortments across channels. Based on live behavioral insights, they adapt store layouts or e-commerce experiences.
Business Benefit: Increases conversion rates and basket size by optimizing real-time merchandising.
Customer Benefit: Enhances product discovery and shopping ease.
Operational Benefit: Reduces reliance on periodic audits or subjective visual merchandising, replacing it with data-backed decisions.
5. Autonomous Loyalty and Engagement Programs
Agentic AI personalizes rewards programs, email campaigns, and mobile app notifications based on individual preferences, past interactions, and predicted behavior. These systems act autonomously, testing and optimizing messages, timing, and channels.
Business Benefit: Improves retention and customer lifetime value by nurturing long-term relationships.
Customer Benefit: Receives highly relevant, timely offers without being bombarded with generic promotions.
Operational Benefit: Marketers can focus on strategy while AI handles micro-level personalization at scale.
As agentic AI continues to evolve, the opportunity lies in moving fast, starting small, and scaling what works to stay ahead of the curve.
What Happens When Agentic AI Is Done Right in Retail? These Examples Prove it
Agentic AI in retail shapes the industry by streamlining operations and enhancing customer experiences. Leading brands leverage AI-driven automation to improve efficiency, reduce costs, and increase engagement.
AI Agents Will Now Take Your Orders at Taco Bell and Pizza Hut
Yum Brands, the parent company of Taco Bell, Pizza Hut, and KFC, is redefining quick-service restaurants with AI-driven automation. Partnering with Nvidia, Yum is rolling out AI-powered voice ordering at drive-throughs and phone lines in 500 locations. The goal? Seamless, human-free ordering leads to higher sales and better efficiency.
Beyond ordering, Yum is deploying computer vision to detect order errors and AI-powered analytics to extract insights from customer feedback. This shift ensures better accuracy, enhanced customer experiences, and streamlined operations. By building in-house AI solutions, Yum maintains innovation control while optimizing costs. Their success showcases how Agentic AI can transform retail, making processes smarter, faster, and more responsive to market demands. (Source: WSJ)
Amazon’s Vision: AI Shopping Agents That Can Shop for You
Amazon is pushing the boundaries of AI-driven shopping experiences, envisioning autonomous AI agents that anticipate and fulfill customer needs. The company aims to develop AI-powered shopping assistants that recommend products, add items to carts, and even complete purchases—all based on user preferences and trends.
Amazon’s Rufus large language model (LLM) is at the forefront, which powers AI-generated shopping guides, streamlining product discovery and decision-making. Future iterations may allow customers to set shopping budgets and delegate purchases entirely to AI. By integrating generative AI with vast retail data, Amazon is transforming e-commerce into a hyper-personalized, frictionless experience—showcasing how Agentic AI can redefine retail convenience and engagement. (Source: Wired)
These Agentic AI in retail examples demonstrate how it can transform the industry by making processes smarter and more customer-centric. As AI continues to evolve, retailers that embrace these advancements will gain a competitive edge in an increasingly digital marketplace.
What the Future Holds for Agentic AI in Retail
The future of Agentic AI in retail is not just about improving what already exists but redefining the retail experience altogether. As customer expectations grow and operational complexities deepen, retailers need AI systems that learn, act, and adapt without constant human direction. That is where Agentic AI becomes essential—not optional.
This is what lies ahead:
1. Autonomous Store Operations
Imagine stores that can manage themselves—monitoring foot traffic, adjusting in-store promotions in real time, reordering inventory, and even shifting staffing needs based on demand predictions. With edge computing and Agentic AI working together, stores could become self-optimizing ecosystems. Companies like Amazon are already experimenting with this through initiatives like Amazon Go, where agents run the store experience with minimal staff input.
2. Real-Time Customer Companions
Retailers will likely deploy customer-facing agents that act as digital shopping assistants. These AI companions will engage with customers across channels, offering style advice, suggesting add-ons, and managing returns. These companions will understand context and intent, not just keywords. They will make interactions feel personalized and human-like, especially as LLMs become more capable and affordable.
3. Collaborative Human + AI Decision-Making
Agentic AI will not replace humans but augment their capabilities. Retail teams will work alongside autonomous agents, focusing on creativity, strategy, and empathy, while agents handle the complexity and data crunching. Think of AI proposing 10 pricing strategies based on predicted competitor moves—and the merchandiser simply confidently selects the best one. The future will be “human-in-command,” not human-in-the-loop.
4. Ethics, Governance, and Trust as Differentiators
With greater autonomy comes greater responsibility. Retailers embracing Agentic AI must incorporate explainability, bias mitigation, and consent into every workflow. Companies that provide responsible AI-based products and services can expect a 25 percent increase in customer satisfaction and loyalty. By being transparent and ethical, the retailers who win customer trust in this area will have a significant competitive edge. (Source: Accenture)
Agentic AI is not a one-time project—it is a long-term strategy. Retailers that adopt this mindset will lead and define the future of commerce instead of simply following trends.
Retail is Being Rewritten—and Agentic AI is the Author
Agentic AI in retail is not just another wave of automation. It is a shift in how retailers operate, customers shop and make decisions. By empowering AI agents to act independently—while aligning with business goals and human oversight—retailers can move faster, serve smarter, and adapt in real time.
While organizations race to implement this technology to gain an early-mover advantage, it requires more than technology—it demands the right strategy, ethical guardrails, and domain expertise.
At Tredence, we help retailers bring this vision to life. Our Agentic AI-powered solutions go beyond predictive models—we build decision-ready systems that blend structured and unstructured data, automate workflows, and bring responsible autonomy into your operations.
We have been working closely with retailers by providing their customers with personalization through AI-driven recommendations, improving demand forecasting using machine learning, and optimizing inventory with advanced analytics.
Partner with Tredence and explore our AI Consulting and Retail AI Services to begin your journey toward an autonomous, customer-first enterprise.
FAQs
1. What role does Agentic AI play in dynamic pricing strategies?
Agentic AI monitors real-time demand, competitor pricing, inventory levels, and market trends to autonomously adjust prices across products and channels. This allows retailers to optimize margins, respond instantly to market shifts, and stay competitive without constant manual oversight.
2. How does Agentic AI help retailers optimize supply chain logistics?
Agentic AI continuously analyzes sales velocity, shipment delays, weather disruptions, and vendor performance to automatically reorder stock, reroute shipments, and flag supply chain bottlenecks. This ensures faster response times and better inventory availability.
3. What are the security risks of using Agentic AI in retail operations?
Agentic AI systems operate autonomously and access sensitive data, posing potential risks, including data breaches, unauthorized actions, and unintended bias in decision-making. Mitigating these risks requires strong guardrails, ethical constraints, real-time monitoring, and rigorous testing to ensure safe, responsible deployment.
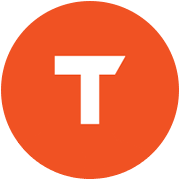
AUTHOR - FOLLOW
Editorial Team
Tredence