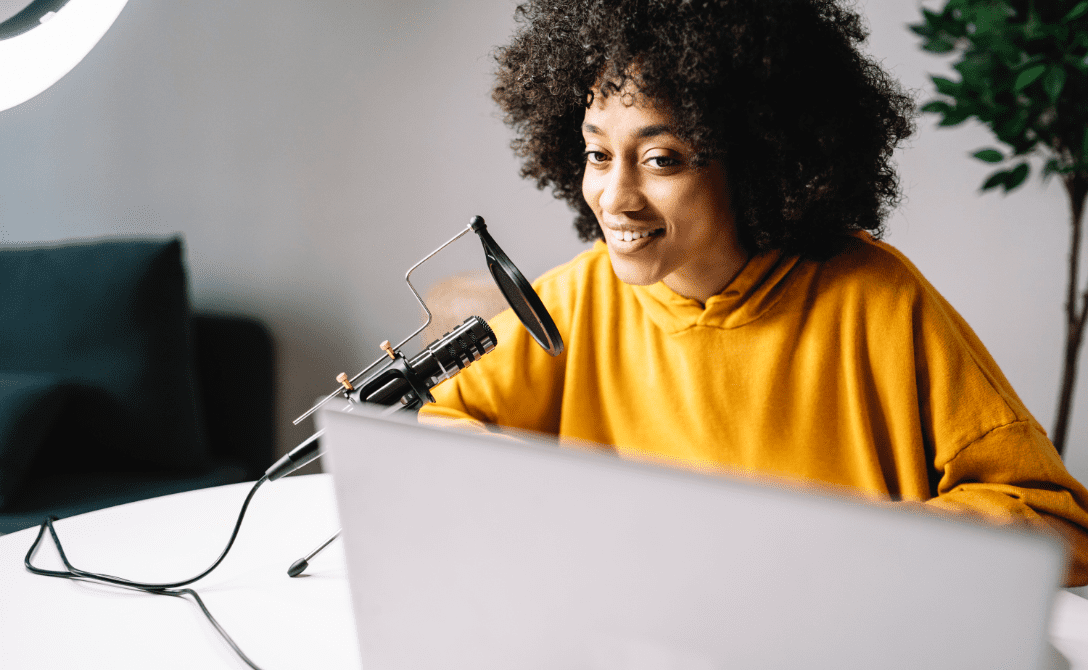
Companies that offer products and services often rely on Customer Experience for their growth. Brands that deliver personalized customer experiences are said to have five times more revenue than their counterparts. Therefore, they need to understand and keep track of changing customer needs through constant research.
In this era of speed, customer satisfaction, and pandemic, attracting new customers and retaining them is the prime focus of any business. However, with a vast amount of unstructured data, it can become tedious to narrow down and find customer’s pain points. To do this effectively, we need to analyze the data and identify the sentiment of each feedback.
Sentiment analysis is a tool to connect with the customer and their problems and find ways to elevate all customers to the “brand loyalist” level, which would prompt them to buy more, stay longer, and refer other customers.
The concept of sentiment analysis is simple yet complicated. There are complex new models available that are setting new benchmarks in our understanding of human language. But we are still figuring out how to solve simple problems like the sentiment in specific use cases where traditional algorithms fail. However, with an increasing amount of data each day, text is not the only medium of communication. 74% of Americans contact customer service on call, and more than 1T$ consumer spending comes from click-to-call influences. Audio calls are fast becoming a goldmine for obtaining insights.
The Need for Sentiment Analysis
Imagine a group of people in the hotel industry dealing with thousands of phone calls and emails from customers every day. It is difficult for them to understand each customer’s feelings about the establishment, brand, or service. In this case, sentiment analysis can help by analyzing data and deriving sentiment from it. It can understand ‘what’ the customer meant and ‘how the customer was feeling about the service.
A regular voice call with customer service or support personnel takes about 10 minutes on average. This call is transcribed, and the sentiment of each statement spoken by the customer and the representative is evaluated. The usual response to a specific query can also be used for internal customer service training and process optimization.
Tredence’s Advanced Customer Feedback Analytics Solution
At Tredence, we have developed a sentiment analysis solution to gain better visibility into the customer experience. The sentiment of both the text and the audio can be analyzed to gain insights. This solution can help you highlight key negative phrases, identify segments where the speaker tone was negative, listen to the selected parts of the call, evaluate speaker’s call time and create an overall sentiment score of the call transcript. This data then can be collated and analyzed from thousands of calls to get an overall sentiment profile of customers in a dashboard, as shown below.
In the image below, we can see that the sentiment score is moving in a negative direction. This can help us drill down into that section and pick the most negative interactions to see the customer call journey.
n a typical call, the sentiment score graph will have some crests and troughs. By analyzing the troughs, we can understand what went wrong, where, and why?
This snapshot gives an overview of how a 15-minute customer call progressed. We can see that the sentiment score dipped when the customer was frustrated. This was not just because of the text they spoke about but also due to the customer’s tone during the call.
How Does Tredence’s Sentiment Analysis Application Work?
Each audio stream here is broken down into small uniform segments to classify them into three types:
- Positive with a score of 1
- Neutral with a score of 0
- Negative with a score of -1
We labeled each audio segment into these categories using a combination of deep learning methods and frequency-based features (such as Mel frequency cepstral coefficients and Mel spectrogram).
The text from the audio transcript can be used to evaluate sentiment simultaneously. Once a baseline is set, multiple deep learning approaches can be taken based on word embeddings to represent text in the numerical space. We used a hybrid of a lexicon-based model and a transformer-based embedding with a Bi-directional LSTM model to achieve accuracy upwards of 90% on real-time data.
Sentiment Analysis Solutions to Augment Business Growth
When the two approaches mentioned above are applied parallelly to an audio call, it gives an idea about the customer experience. Using the intelligence from this data, we can analyze the pulse of the customer and take a multitude of actions to improve business.
This solution is offered as a part of Voice of Customer, impacting businesses in the hospitality and retail sectors. Your customers may feel the need to reach out to you for business or iron out any issues or concerns. Using this tool, you can rest assured that you fully understand their problems and take corrective measures to retain them. Not only will this help in preserving business but also attract new customers owing to the customer-centric focus. This would also help in improving your brand reputation and increasing revenue.
Would you like to turn your customer feedback into actionable insights that improve your product or service? Drop a line to the experts at Tredence who can help you with a tailored solution that can act on the customer sentiment by recalibrating your business strategies.
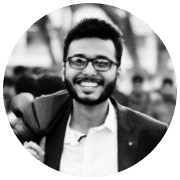
AUTHOR - FOLLOW
Shubham Pandey
Data Scientist
Topic Tags