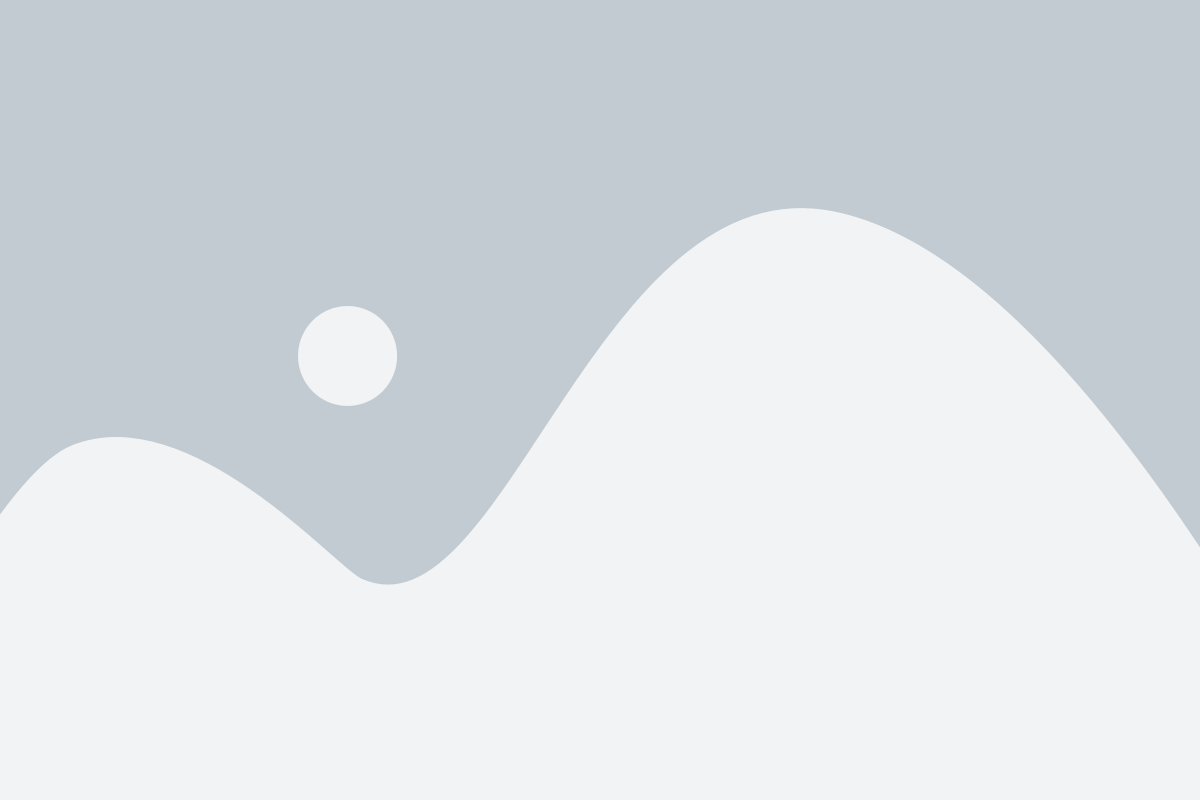
The Role of Data Science in Predicting Demand and Managing Phantom Inventory
Retailers go to extreme lengths to ensure they minimize stock-outs on both in-store and digital shelves. Over the last few years, their teams have leveraged data science to assess a huge volume of historical transactions and customer interactions and accurately predict future demand many times at an SKU level.
This visibility has helped buyers, warehouse managers and store ops evaluate demand with current inventory levels and plan replenishment in advance. However, there are times when the inventory system shows a product in stock, even though, in reality, the product is not available. As a result of this phantom inventory, planners end up delaying the replenishment which, in effect, derails the entire operation.
One of the most common and notorious reason for phantom inventory is shrinkage, where loss of product happens due to theft or fraud. Today, shrinkage is at an all-time high at 1.6%, with over 15% of retailers experiencing more than 3% shrinkage, according to the National Retail Federation. Errors in recording products received, damaged goods, sales, and more also lead to phantom inventory.
Implications of Phantom Inventory
- Revenue loss: If a product is not physically in stock, it can’t be sold, directly affecting the retailer’s topline.
- Customer loyalty dilution: For an average shopper, product availability is one of the top three reasons they choose a retailer. If they don’t find what they need, they are more likely to switch. In fact, research finds that over 24% of Amazon’s revenue is a result of a physical retailer’s on-shelf availability issues. So, this is not just loss of revenue from the sale of a particular product, but lifetime loss of the customer itself.
- Omnichannel disruptions: If in-store stockouts is a customer experience problem, it gets multiplied in an omnichannel setup including e-commerce delivery and curbside pickups. Customer dissatisfaction is significantly higher when an order has been placed and is then only partially fulfilled or, worse, cancelled for lack of stock.
- Replenishment inefficiencies: Most retailers have an auto-replenishment mechanism that orders products when the system shows the inventory to be lower than a predetermined threshold. However, if the system doesn’t reflect the out-of-stock situation, no reorders will be placed, extending the timeline of the problem.
- Inaccurate future demand prediction and demand forecasting: When a product has phantom inventory, it is likely to see no scans or ‘significantly lower than usual’ scans. Retailers might assume this is due to extraneous factors causing sales drop, leading to inaccurate forecast of future demand and demand forecasting.
Challenges in Addressing Phantom Inventory
Despite significant direct and indirect revenue leakage, most retailers leave the phantom inventory problem unaddressed due to:
- Lack of data to correlate stock levels on the systems with on-shelf availability.
- Thorough and regular inventory audits can also be time- and labor-intensive.
Supply Chain AI Strategies for Phantom Inventory Optimization
However, an emerging solution for this Gordian Knot lies in a robust data management solution and a comprehensive supply chain strategy. Here’s how you can leverage that for your retail organization.
#1 Consolidate data siloes and connect the dots
As a retailer, it is important to have to a connected data strategy which enables acquiring and linking of data from suppliers, manufacturers, wholesalers, stores, point-of-sale (POS), etc. Clean, standardized data creates end-to-end visibility and makes predicting phantom inventory easier. For instance, if POS data shows sales anomalies/outliers for specific SKUs between last week and the current week, the analytics engine can automatically flag this situation and conduct a rapid reconciliation across systems.
#2 Set up the right metrics
A FMI/GMA Trading Partner Alliance report on the out-of-stock problem finds that the very definition of on-shelf availability varies widely from physical audits to zero-on-hand metrics to minimum display values. Standardized metric definitions enable an entire organization to speak one single language, minimizing confusions and maximizing actionability.
Set baselines for on-shelf availability, out-of-stock, zero scans and more. Track zero scan and off-scan events using POS data streams. Consider seasonality and cyclicity while identifying off-scan events. Recognize the safety stock of each SKU at the store level. Based on all these insights, get clear visibility into phantom inventory.
#3 Drive actionability through a rapid alert in mechanism
Going beyond just building a dashboard for insights, set up an analytics engine that sends real-time alerts and recommendations to various personas throughout the supply chain.
- Set up alerts for key metrics such as on-shelf availability, out-of-stock and zero scan
- Customize alerts by changing the conditions based on business requirements
- Identify push opportunity (land grab) alerts
- Prioritize alerts about high-value SKUs at the store-level to optimize every dollar spent
- Prioritize alerts based on each user persona, sending what’s relevant to them
- Pool alerts from SKU-level analysis for focussed action
- Suppress repetitive/redundant alerts based on business rules
#4 Forecast better
With the foundation of such comprehensive data, forecasting becomes much simpler. Leverage advanced ML algorithms to forecast sales from historical data across ??demand, supply, inventory, pricing and logistics. Based on these forecasts, you can strengthen your supply chain to prevent OSA, OOS and phantom inventory.
Conclusion: Strengthening the Supply Chain with Analytics and AI
As the retail landscape across the globe becomes increasingly competitive, especially with greater push from data-savvy online players, traditional retailers can no longer ignore key supply chain issues such as phantom inventory. Even a 1% loss of sales due to phantom inventory can not only translate to millions of dollars in losses, but also have a long-lasting impact on customer retention and loyalty.
A robust on-shelf availability analytics engine can make the supply chain more resilient to disruptions and empower store and warehouse teams with transformative insights in real time, allowing them to create better customer experience every time.
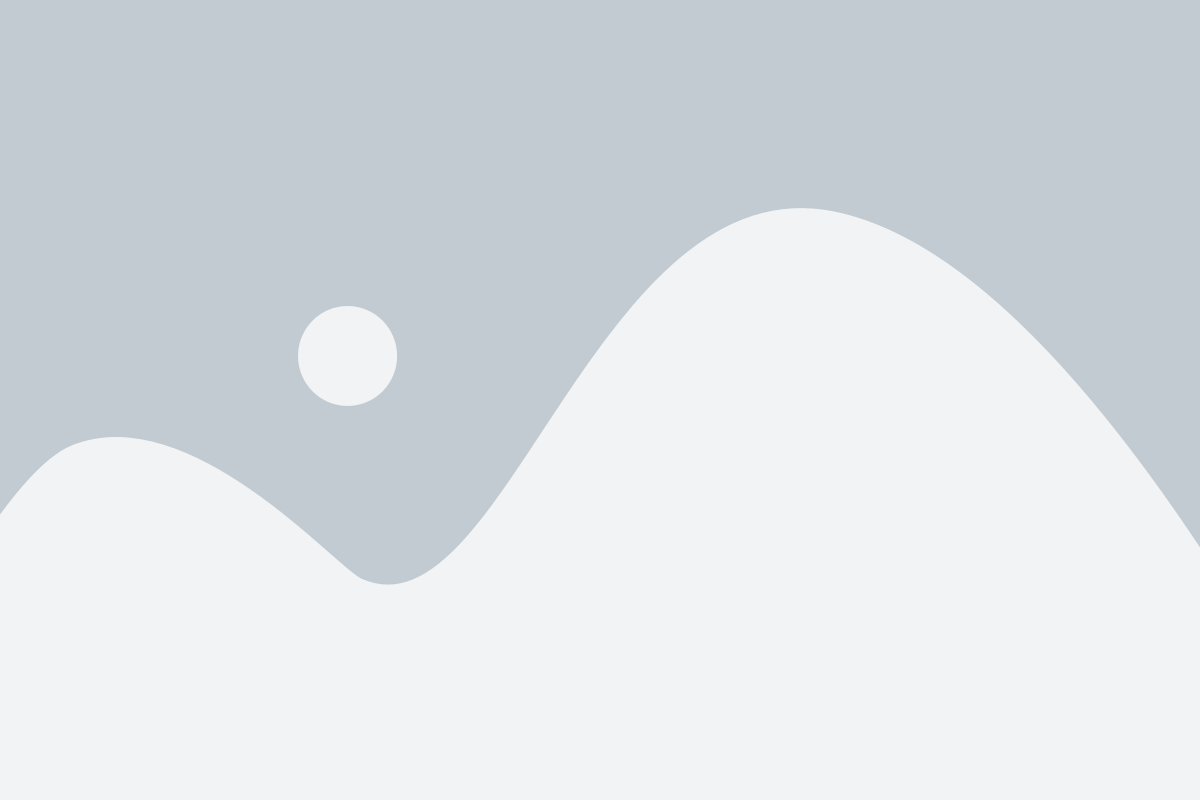
AUTHOR - FOLLOW
Vidit Agarwal
Senior Director, Tredence Inc.
Topic Tags