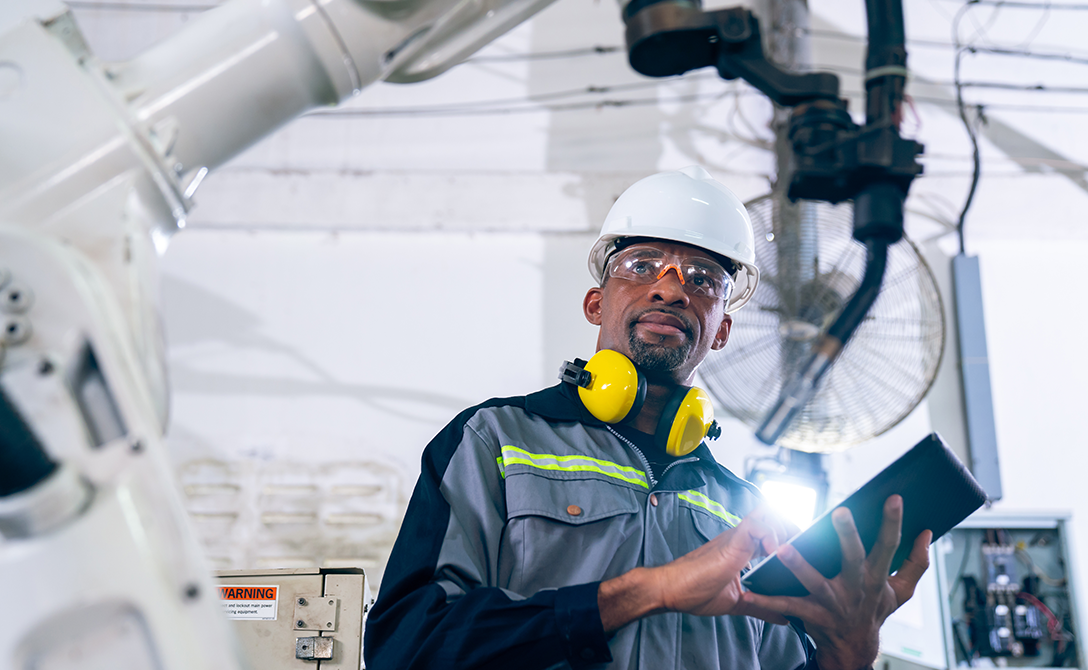
The recent supply chain disruptions have demonstrated that history is no longer reliable when comprehending customer behavior. Systems predicting demand haven't been well-suited to deal with worldwide disturbances in our daily lives. However, as unanticipated panic buying took hold across 2020, causing product shortages, consumer packaged goods (CPG) manufacturers and retailers were caught off guard. As it turned out, the Covid-19 outbreak in 2020 was a prelude to what was to come. Ongoing AI disruption, supply chain disruptions, inflation, and the emergence of Covid-19 variants have severely hampered demand forecasting.
Understanding demand forecasting in an AI-driven world
A new chapter is beginning in deploying the most recent generation of AI technology: AI application development and implementation are becoming smoother as capabilities increase, and businesses are noticing major benefits from adoption. According to a PwC study, with AI-based extraction techniques, companies can expect to save up to 40% of the time and resources typically spent on processing documents and simplifying operational procedures.
Additionally, AI demand forecasting provides real-time insights into customer preferences and trends, allowing organizations to anticipate changes in order to adjust their operational strategy better. With the right tools and processes, businesses can leverage AI to increase efficiency throughout their supply chain, from material resource planning to financial forecasting. Doing so can ensure they remain competitive while staying on top of rapidly shifting customer needs.
Harnessing the power of demand forecasting to improve planning and efficiency
Demand forecasting has become increasingly important for businesses as it allows them to plan accurately for the future. With improved forecasting, companies can drive better decisions regarding cash flow, risk management, capacity planning, and workforce planning.
For example, according to McKinsey, companies can mitigate risk by 20% to 50% by implementing AI for forecasting in their supply chains. This makes demand forecasting a critical tool for any company looking to maximize operational efficiency while minimizing operating costs. In addition, using demand forecasting tools can help companies save up to 15% in inventory costs and reduce the need for manual labor by up to 40%.
Now, before we jump guns on the nitty-gritty of demand forecasting, let's shed light on what it means and why it is so relevant in the current disruption-ridden world.
Demand forecasting: Unpacking its relevance in a disruption-prone world
Demand forecasting is a subfield of predictive analytics, and, as the name suggests, it is the process of projecting future demands. Organizations implement demand forecasting tools to prevent inefficiencies brought on by supply and demand misalignment throughout their business processes. Utilizing demand forecasting solutions, businesses can enhance their decision-making about cash flow, risk assessment, capacity planning, and workforce planning.
Demand forecasting is crucial to efficient supply chain management. When combined with advanced analytics, demand forecasting can help companies make informed decisions about cash flow, risk assessment, capacity planning, and workforce planning. To do this, organizations must examine all the internal and external variables that impact their supply infrastructure, providing them with a comprehensive picture of any patterns and anomalies that could influence their demand forecasts.
Publicly available surveys have shown that properly implementing demand forecasting leads to a 3-5% decrease in inventory levels while increasing customer satisfaction by up to 20%. With so many advantages, demand forecasting has become a must-have tool for any modern business looking to reach its full potential.
An in-depth examination of the parameters that could impact an industry's supply infrastructure is essential for accurate demand forecasting. For example, demand forecasting in the supply chain requires rigorous research of several variables, ranging from historical sales patterns to specific events on the retail calendar, to assure business readiness, control, continuity, increased planning, and a superior end-user experience.
How does demand forecasting in the supply chain work?
Supply chain demand forecasting plays a pivotal role in anticipating market needs and optimizing inventory management. The two kinds of demand forecasting—qualitative and quantitative—can be distinguished by the resources and data sets they employ to infer valuable sales data.
The quantitative forecasting approach is applied when there are previous sales figures for certain products and a known demand. It necessitates the application of mathematical formulas and data sets, including financial reports, sales, revenue, and website analytics.
On the other hand, the qualitative approach is based on the forecasters' intuition and experience, developing technologies, shifts in pricing and availability, product lifecycles, and product upgrades. Finally, using qualitative methodologies, the demand planner can incorporate internal and external expert viewpoints. Integrating supply chain demand forecasting techniques enables businesses to adapt swiftly to market fluctuations and enhance overall operational efficiency.
Creating accurate demand forecasts with proven strategies
Demand forecasting solutions include several submethods from both quantitative and qualitative methodologies. For example, many organizations implement multiple demand forecasts and employ more than one forecasting approach. For the astute demand planner, a blend of both is ideal.
For supply chain management, quantitative forecasting frequently uses Big data. Quantitative forecasting techniques that are widely used include:
- Barometric Forecasting: It utilizes statistical analysis to predict future demand. This approach is based on existing data and market trends. By analyzing historical patterns, like seasonality and long-term growth, as well as external factors, like economic indicators and consumer behavior, it's possible to get a more accurate picture of the future market. Using powerful analytical tools such as regression analysis and time series methods, barometric forecasting allows businesses to plan and make informed decisions that can impact their bottom line.
- Trend Projection: It is an essential business forecasting and planning tool. It uses historical data to create a projection of future market demand, considering factors such as sales volume, growth patterns, seasonality, and other related trends. By utilizing this technique, businesses can more accurately forecast their sales goals and better plan for production and inventory needs. Trend Projection also allows companies to recognize emerging market trends and adjust strategies accordingly to capitalize on new opportunities or mitigate potential risks.
- Exponential Smoothing: This powerful forecasting approach considers historical data and seasonal variations when predicting future sales. It uses an algorithm to recognize patterns in the data and then applies a smoothing factor to ensure that the result is as accurate as possible. This technique allows for more accurate predictions than traditional forecasting methods since it can account for the fact that sales may fluctuate from one season to the next. On the other hand, exponential smoothing also provides greater flexibility than other methods since it can be adjusted to reflect changes in market conditions or consumer preferences over time. Additionally, this method is often used alongside other techniques, such as regression or time series analysis, to increase accuracy.
- Econometric Forecasting: This method utilizes advanced statistical models and equations to forecast future demand. This method takes into account the various external factors that can impact a market, such as economic conditions, consumer behavior, industry trends, and technological advancements. These variables are combined with historical demand data to generate a more accurate demand forecast than other methods. Furthermore, econometric models can be tailored to a business's specific needs, allowing for an even more precise estimation of future demand.
Machine learning and AI-based demand forecasting solutions can be applied to all quantitative demand forecasting tools. For example, you can optimize your inventory management and stay ahead of supply chain pitfalls with the help of software that employs AI to react swiftly to market developments.
Methods for qualitative forecasting rely more on human inputs and less on statistics. Several of the most popular techniques for qualitative forecasting include:
- Sales Force Composite: The approach combines multiple teams' skill sets to create a more reliable and accurate analysis of market demand than traditional forecasting methods. It leverages the knowledge and insight of members across various departments, such as sales, supply chain management, and other stakeholders. This combined expertise enables a better understanding of customer preferences and behaviors, allowing business owners to decide what products to stock and when. Additionally, this strategy helps optimize inventory levels based on anticipated future demand while reducing waste. All in all, the Sales Force Composite approach provides businesses with the data-driven insights they need to stay competitive in today's ever-changing market.
- Market Research: This forecasting technique involves considering factors such as customer preferences, economic and political trends, competitor analysis, industry trends, and the overall environment to understand the current market and predict future demand. It is important to consider how the supply chain works in a given market, including production capacity, distribution channels, availability of raw materials, and other potential constraints. Additionally, changing consumer demands should be considered when forecasting market trends. Market research can also help develop expansion plans and promotional strategies to help meet current and future demands.
- The Delphi Method: This is a systematic approach used to efficiently and accurately reach a consensus regarding the forecasted demand for a given product. It involves gathering a panel of experts who are then asked questions about their predictions. After the initial responses are compiled, the demand planner will present this summary to the board for further discussion. This process continues until all parties agree on an exact forecast, ensuring that each expert's opinion is considered to arrive at a well-informed decision. With its iterative process and focus on collaboration between experts, the Delphi Method has been proven to be an effective tool in predicting future demand and making accurate decisions regarding product supply.
What demand forecasting brings to the table
Retail chains that depend on precise stock projections for their long-term survival and health can benefit tremendously from demand forecasting. Furthermore, the perks go beyond preserving high levels of customer service; they can spur improvements across several areas, boosting corporate confidence and aiding an organization in attaining its objectives for growth.
- Reduced uncertainty: When it comes to supply chain management, uncertainty is never good. Demand forecasting in the supply chain can eliminate ambiguity by ensuring that supply-related tasks are adequately resourced and that managers are well-informed to make wise decisions that drive growth and progress.
- Enhanced supply infrastructure: For the health of your supply infrastructure, predicting demand peaks and troughs is critical. Demand forecasting contributes to enhanced supply chain management by maximizing capacity, stock replenishment, and the efficient management of warehouse workers.
- Increased revenue: Revenue can grow significantly by knowing when and in what volume a product is likely to sell. Maximum-suited product availability ensures you never miss a sale thanks to demand forecasting.
- Reduced demand for safety and surplus inventory: The more inventory you have in your warehouse, the longer it takes to turn around stock, and the more it affects your bottom line. Demand forecasting in the supply chain minimizes the need for safety stock, allowing you to concentrate on your most lucrative products while eliminating inventory expenses.
- Better fulfillment results in longer-lasting consumer loyalty: Demand forecasting tools make your products available more consistently, which can benefit customer advocacy. Accurate demand forecasting in the supply chain can improve order fulfillment by driving customer loyalty and word-of-mouth recommendations, boosting demand and your clientele.
Putting AI at the heart of Supply Chain Management
Predictive maintenance is one area where AI can enhance business performance. This is because deep learning can examine huge amounts of high-dimensional data. AI can streamline delivery traffic routing in logistics, increasing fuel efficiency and cutting down on delivery times. Thanks to enhanced speech recognition, AI has emerged as a useful tool in call centers for managing customer care. Individualized "next product to buy" suggestions can be created in sales by merging customer demographic and historical transaction data with social media monitoring, which many retailers now employ often.
More and more businesses have been experimenting with AI recently, developing their data-related capabilities, obtaining new knowledge and equipment, and incorporating AI into their operational procedures. In addition, businesses should strive to push the limits of what they can do with AI in an era of pervasive AI when capabilities are easily accessible to everyone. Supply chain leaders should aim to develop fresh AI-enabled products and services and use AI to unearth novel insights, enable new business paradigms, and give them a competitive edge in the industry.
How businesses will combat AI disruption and thrive with AI demand planning
A significant technological tipping point has been reached in recent years, even though specialized analytics solutions for supply chain management and AI demand planning have been around for a while. As a result, enterprises can process data and automate processes at a volume, speed, and sophistication beyond traditional analytics thanks to the development of machine learning and artificial intelligence solutions.
Organizations want to enhance their present BI insights, whether it be a bank enhancing fraud detection, a healthcare provider switching to telehealth, or a retailer or manufacturer striving to increase supply chain efficiency. Your organization's success can be aided by knowing how different industries intend to employ AI to recover from the pandemic and the conflict. For almost every organization and industry, the gradual recovery from COVID-19 and the Russia-Ukraine conflict is of the utmost importance.
BANKING
Banks and other financial institutions will be on the lookout for both significant opportunities and perceived risks. The prolonged suppression of interest rates will be a huge problem since constricted spreads will hurt profitability. Banks that continue to adopt antiquated models will gradually lose revenue, market share, and, in some cases, reputation. It will therefore be crucial to have the ability to rapidly update models in fields like underwriting, customer management, fraud, and collections, to highlight a few.
FINANCIAL MARKETS
The securities industry benefited from the government's responses to the pandemic in terms of the financial markets. Given that volumes appear destined to decline, we might see some retrenchment; consequently, cost containment will likely receive more attention.
Given how quickly things will continue to change, agility will be more important than ever. This is where automated machine learning model development excels; by utilizing advanced AI consulting services. This way, an AI model's development and governance lifecycle can be accelerated from months to days or weeks. However, machine learning operations will become even more crucial due to a growing emphasis on effectively monitoring and securely implementing AI solutions.
RETAIL
Consumer behavior and the competitive environment will continue to undergo radical changes in the retail market. Jobs, the economy, and the logistics of reducing the war and pandemic restrictions in various regions will continue to pose hurdles to business. Retailers will be compelled to incorporate AI into their business decisions—especially to comprehend the underlying data that is changing swiftly. Adopting AI will put retailers in a better position to deal with demand volatility and stabilize needs, gain a first-mover advantage over time, and eventually reimagine and reform their operations.
AI will better relate to evolving consumer wants as retailers move their operations online and become more functional. As a result, retailers can make better store location and repurposing decisions, optimize pricing, and alter demand forecasting.
HEALTHCARE
The healthcare sector has experienced significant disruption to its operational models, an inversion of revenue drivers and cost centers, new patient categories, and pervasive illness among frontline personnel. Moreover, they have observed the growth of current operational efficiency use cases, staffing forecasts, and staff attrition AI use cases.
The repercussions of COVID-19 have changed health insurers' claims and payment environment by causing a rapid decline in overpriced elective operations, new regulatory guidelines, and new pandemic-related revenue sources. As conventions are disrupted, AI will be a core capability as healthcare industry institutions strive to integrate operational and healthcare-specific AI use cases.
MANUFACTURING
Global manufacturers can estimate demand for their products downstream and reduce supply chain risks upstream with the help of machine learning. However, since the hard reset in early 2020 diminished the predictive value of historical data, AI and machine learning approaches will aid in creating the most accurate demand forecasts with this limited data set. In other words, machine learning algorithms can achieve more with less when estimating the underlying demand for produced items.
Machine learning will also enhance the accessibility of sophisticated upstream supply chain management by enabling global manufacturers to better anticipate and mitigate risks brought on by geopolitical, transportation, and economic developments.
Connecting demand forecast to supply chain process
Without a time-to-action that follows suit, an expedited time-to-insight is futile. This is another critical area where AI and machine learning set themselves apart from traditional forecasting. For example, ML and AI can automate process decision-making, while conventional predictive analytics may supplement decision-making with insights. This results in an integrated demand sensing and response mechanism that can keep up with real-time market changes.
ML and AI can run endless simulations based on their predictive models to evaluate the effectiveness of every decision made regarding pricing, cost changes, inventory forecasting, merchandise planning, and other demand response actions. This results in the best decisions possible that drive profits, optimize stocks, and move excess inventory.
Increased planner productivity is a secondary benefit of automated decision-making. Demand planners are liberated from non-value-adding tasks like generating reports and using demand planning tools, allowing them to concentrate on more strategic objectives. In addition, it enables businesses to grow while limiting the rise in overhead that often comes along with such expansion. Enterprises can expedite their business outcomes while also improving the overall quality of their processes and the resilience of their supply chains by implementing ML and AI as decision automation mechanisms.
The future of ML and AI in demand forecasting
Businesses will enhance forecast accuracy and streamline restocking strategies by integrating AI and ML into their supply chain management. For example, demand forecasting in the supply chain is advanced by data analytics, which allows for enhanced forecasts based on real-time data from internal and external data sources, including demographics, weather, traffic, events, consumer tastes, economic indicators, online reviews, and social media. In addition, supply chain networks can perform better than those maintained manually by data analysts and react to external changes using external data and sophisticated AI/ML techniques.
Businesses have shown a significant performance improvement in their models over conventional and spreadsheet-based forecasting after using AI-driven demand forecasting across a wide range of industries with varied data environments and will continue doing so in the coming years.
Bottomline: Invest in AI demand forecasting for accurate predictions & resilience in the supply chain
The most important criterion for a robust forecasting process has the correct data. The benefits of applying ML- and AI-powered demand forecasting solutions for this are manifold. False forecasts from incorrect data sets may affect an organization's sales and profitability. As a result, organizations could create accurate predictions at all levels by implementing these approaches. According to Gartner, 87% of supply chain experts intend to invest in resilience and AI/ML in the coming future.
AI can even consider external factors that may influence demand, saving time and funds compared to conventional processes. For example, demand forecasting solutions will be driven by next-generation AI and ML for most businesses. Therefore, you must optimize demand forecasting to streamline your supply chain to raise sales while minimizing excessive expenditure profitably. The vertical-specific demand forecasting accelerators from Tredence combine cutting-edge analytics and distribution data to guide business decisions, enhance inventory flow, and equip you with the resources required to expand your e-commerce store. Get in touch with us today!
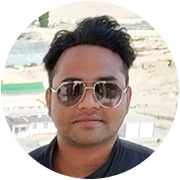
AUTHOR - FOLLOW
Abhishek Kumar
Associate Manager, Marketing
Topic Tags
Detailed Case Study
Driving insights democratization for a $15B retailer with an enterprise data strategy
Learn how a Tredence client integrated all its data into a single data lake with our 4-phase migration approach, saving $50K/month! Reach out to us to know more.
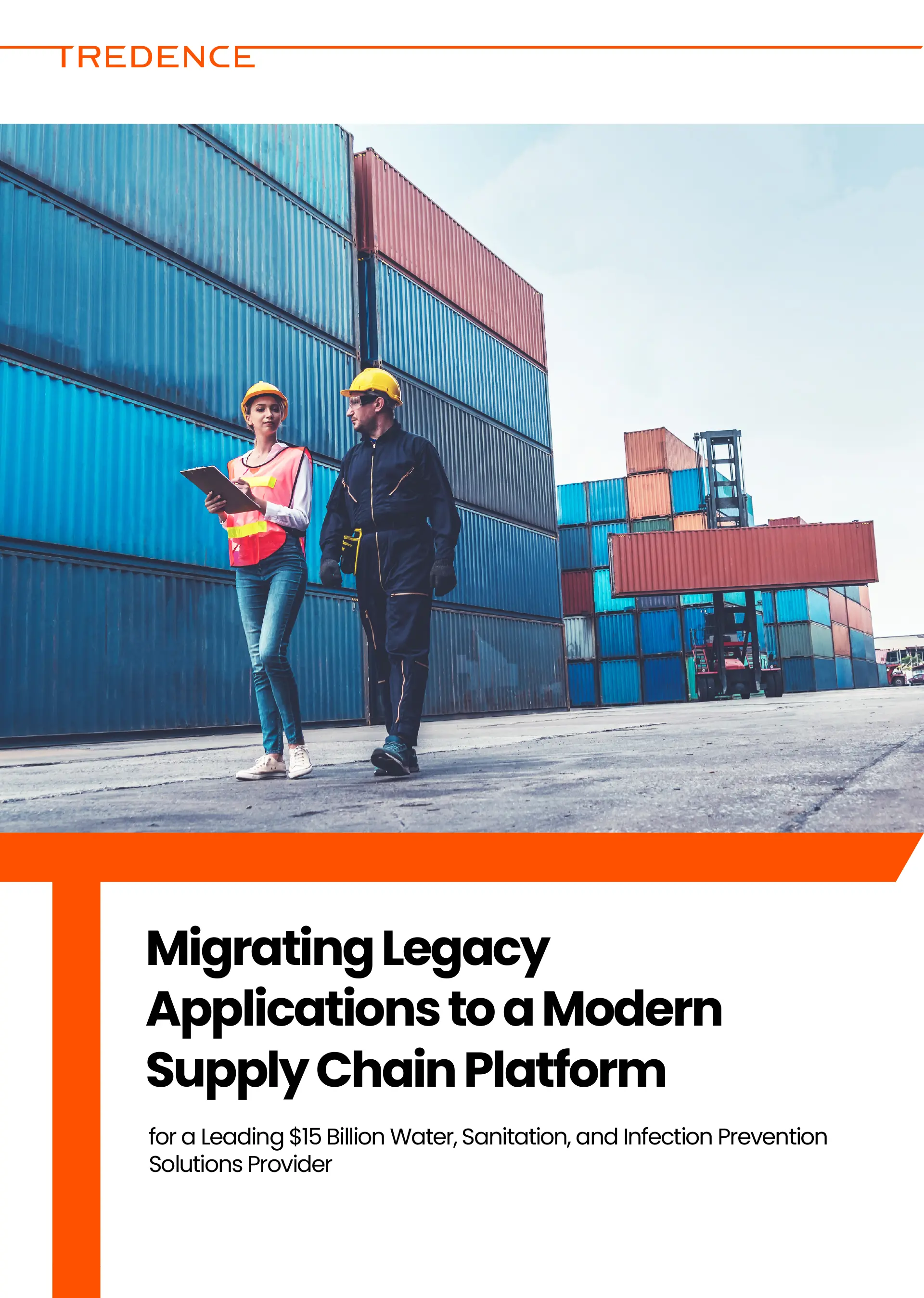
Detailed Case Study
MIGRATING LEGACY APPLICATIONS TO A MODERN SUPPLY CHAIN PLATFORM FOR A LEADING $15 BILLION WATER, SANITATION, AND INFECTION PREVENTION SOLUTIONS PROVIDER
Learn how a Tredence client integrated all its data into a single data lake with our 4-phase migration approach, saving $50K/month! Reach out to us to know more.
Next Topic
History of Supply Chain Disruptions: The Past, Present, and Future | Tredence
Next Topic