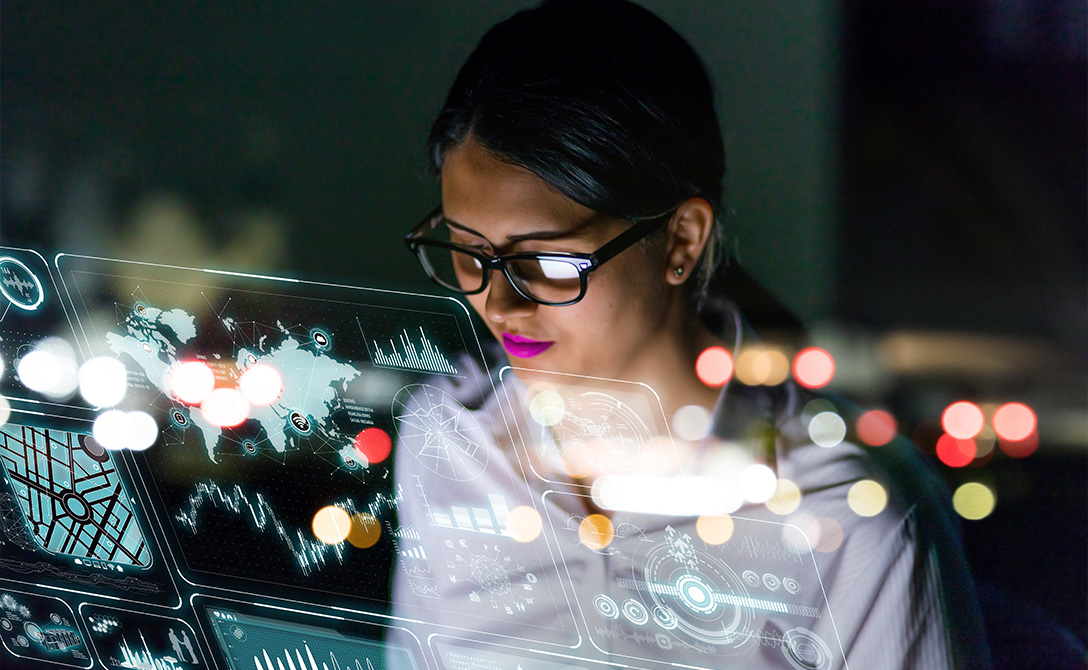
Enterprise data is all the data that retail organizations generate, including customer purchase history, punched feedback, loyalty forms, and real-time inventory levels and trends from social media channels.
In the retail industry, data is everything
Retailers rely on data to make informed decisions about product assortment, pricing, and promotions. Enterprise data is all the data that retail organizations generate, including customer purchase history, punched feedback, loyalty forms, and real-time inventory levels and trends from social media channels. This is utilized to develop a more personalized shopping experience, improve operations, and make better investment decisions for the future.
To use this data effectively, retailers need to have systems and processes to collect, manage, and analyze enterprise data. They also need to have the right team in place with the skills and knowledge necessary to glean insights from the data that can be used to improve customer service and products. When done effectively, enterprise data can help build strong loyalty and customer advocacy, and give retail organizations a differentiated competitive edge.
Utilizing Customer Data to Increase Sales and Improve Customer Experiences
Any retailer worth their salt understands that happy customers are the soul of their business. They do not only return customers, but they also operate as walking (or scrolling) advertising, bringing in new business through word-of-mouth.
So how can retailers create a positive customer experience that will keep shoppers coming back for more?
Today, retailers must replace generalized experiences with customized consumer pathways. Data plays an essential role in deciphering the customer journey and understanding consumer preferences. Being truly customer-centric involves keeping the customer at the heart of all decisions, ranging from layout to product assortment to product price. For example, the deli section could ideally be placed next to the snacks section, even though this may yield lower margins. This recognizes a consumer need and contributes to customer delight, ensuring repeat visits and long-term returns. Trader Joe’s runs a discovery-based ‘treasure hunt’ pattern planogram while IKEA executes a scripted path through their stores. Both decisions, though contradictory, are based on an extremely incisive understanding of core consumer behavior.
Such an integrated data platform merges real-world experience with digital exploration to build a 360-degree view of the customer. With data points like what products are being viewed and added to carts but not purchased, retailers can change their inventory and merchandising to better meet customer needs. For example, if a customer frequently buys coffee beans, the retailer could suggest related items like filters or mugs.
Developing similar insights involves organizing the data and developing an analytics and data science layer to reach the right customers through the right channel with the right products and prices at the right time. At the end of the day, isn’t that what it’s all about?
Key Applications of Enterprise Data
Analytics has become increasingly significant in the retail industry in recent years. By analyzing large data sets, retailers can gain insights into consumer behavior and make more informed decisions about their business operations. Here are some key applications of enterprise data in the retail industry:
Demand Forecasting: By analyzing past sales data, retail businesses can better understand future demand and plan their inventory accordingly.
Meeting Changing Customer Expectations: Analytics help organizations understand customer behavior and preferences so that retailers can adjust their offerings accordingly.
Consumer Sentiment Analysis: Monitoring social media and other online chatter provides a pulse on consumer sentiment around brands or products.
Data Monetization: In the brick-and-mortar world, premium planogram spaces were auctioned to vendors. This was the first step in data monetization. Increasingly, retail businesses are finding ways to monetize their omnichannel data, by partnering with third parties or using it for better-informed marketing and advertising efforts.
Trend Analysis: By analyzing data on industry trends, enterprises can stay ahead of the curve and capitalize on emerging opportunities, or do an immediate course correction if an offering isn’t resonating with its intended customer base.
Impact of Data Science on Retail Businesses
Data science has a significant impact on the retail industry. By leveraging data to improve productivity, efficiency, and scalability, retail businesses can stay ahead of the competition and keep pace with the ever-changing retail landscape. Data analytics is helping retail businesses to optimize their operations, understand customer behavior, and make better decisions. In this context, there are several use cases for detecting unhappy customers through computer vision, using security cameras to identify stock shrinkage, and recognizing when delivery trucks need maintenance. Analyzing these situations allows retailers to improve their productivity and effectiveness in a scalable manner.
Other than the customer-facing aspects of data science, we have powerful analytical ecosystems which ingest SNEW – Social, News, Events, and Weather – external factors which impact consumer choices and supply chain factors. While the approach and toolkits to be used depends on individual business needs and strategic focus areas, every function and scenario definitely benefits from data science and analytics.
Potential Challenges or Risks When Using Enterprise Data Analytics Tools
As businesses design their data strategy, adoption is likely a major risk factor. Therefore, a flexible, adaptable, and customizable tool for local knowledge is essential to mitigate the lack of adoption.
Second, retail companies should be aware of data bias when using these tools. Third, data bias can lead to inaccurate results and bad decision-making if not properly managed. Finally, retail companies should ensure that they have the necessary security measures to protect their data when using enterprise data analytics tools.
Using enterprise data to enhance customer experience is also a slippery slope since targeted messaging can also lead to consumer distrust, annoyance, or resentment if not done carefully. Retailers must tread with caution to ensure that enterprise data is utilized to benefit both their business and customers.
By understanding these potential risks, retail companies can be better prepared to leverage the power of enterprise data analytics tools.
Aligning Data Strategy and Digital Transformation
As retail businesses move towards digital transformation, aligning data strategy with this goal is essential. The retail industry is becoming increasingly data-driven, and retail businesses need to collect, analyze, and use data effectively to stay competitive. A data-centric approach can help retail businesses improve customer experience, optimize operations, and improve inventory and pricing decisions.
Most AI/ML application and digital transformation projects fail due to a lack of adoption by the actual business users. Digital transformation journeys are best executed in a “Crawl – Walk – Run” pattern, where retailers work closely with analytical partners who will spend considerable time explaining the outputs and the inherent business intelligence that rests within retail buyers/merchandisers and logisticians. It is important that the technology ecosystem moves in lock step with the evolution of applications and their adoptions.
Retailers are experiencing a data gold rush. To stay disruptive, retailers need to develop their unique capabilities, leverage enterprise data, and build monetization models across the value chain to drive profitable revenue growth.
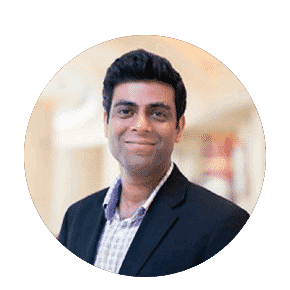
AUTHOR - FOLLOW
Keshav Raj Nagar
Associate Principal
Topic Tags