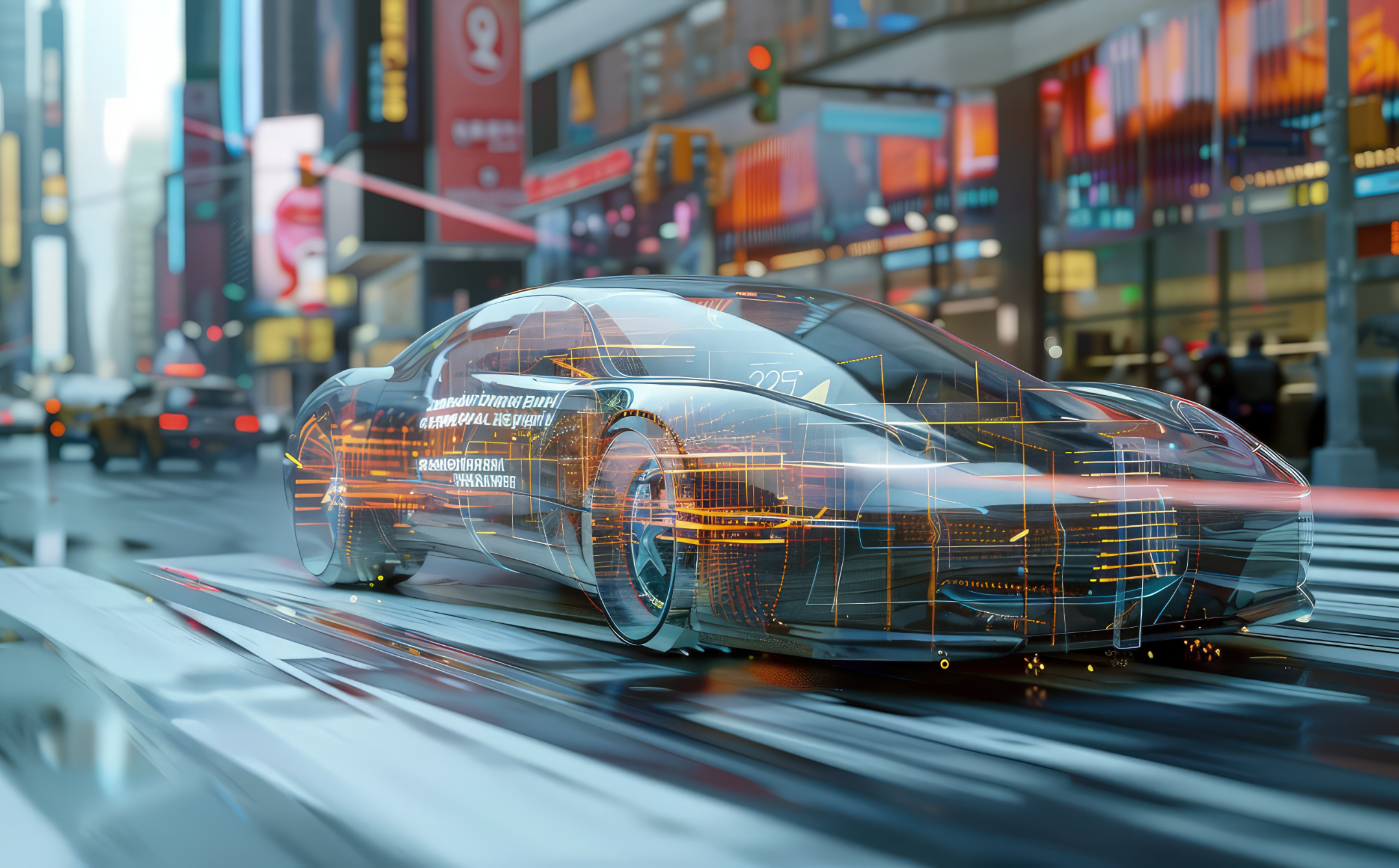
Object detection is pinpointing where objects exist within an image (known as object localization) and assigning them to specific categories (object classification). It's a preferred approach over image classification in numerous scenarios because it identifies objects and precisely locates them. Object detection architectures fall into two main categories: single-stage and two-stage detectors.
Two-stage detectors follow a sequence of steps: first, they extract features, then generate proposals, and finally perform classification. On the other hand, single-stage detectors like YOLO (You Only Look Once) execute detection in a single step, which makes them popular due to their accuracy, lightweight design, and suitability for edge deployment. YOLO architectures are increasingly favored among single-stage detectors for their compatibility with industrial requirements.
In object detection, enhancements have been achieved by employing compact filters to predict object categories and bounding box adjustments. These filters vary based on aspect ratios and are applied across multiple feature maps to detect objects at different scales. This methodology enables high accuracy even with low-resolution input, thus accelerating the detection process.
SSD: Single Shot Detection
SSD is an object detection technique that uses a convolutional neural network (CNN) to detect objects within images. It predicts both the bounding boxes (the boxes that outline the objects) and the class labels (what type of object it is) for each detected object.
SSD Architecture Flow
- Feature Extraction: It starts with a base CNN that extracts features from the input image.
- Multi-scale Feature Maps: These are layers added on top of the base CNN that progressively decrease in size. They allow SSD to detect objects at different scales in the image.
- Convolutional Predictors: Each feature map layer uses small convolutional filters to predict a fixed set of bounding boxes and their corresponding class labels. These filters are like small templates that scan the image and predict whether there's an object present in a specific location.
- Default Boxes: For each location on the feature map, SSD associates a set of default bounding boxes with different sizes and aspect ratios. These default boxes serve as references for predicting the final bounding boxes.
- Matching Ground Truth Boxes: During training, SSD matches these default boxes with the ground truth boxes (the actual objects in the image) based on their overlap. This helps the network learn which default boxes correspond to real objects.
- Training Objective: SSD's training objective involves two main components:
- Localization Loss: This measures how accurate the predicted bounding boxes are compared to the ground truth boxes. It measures how well the predicted bounding boxes (l) match the ground truth boxes (g). It uses a Smooth L1 loss function to calculate the difference between the predicted and ground truth box parameters (center coordinates, width, and height)
- Confidence Loss: This measures how confident the network is in its predictions of an object's presence and its class label. It uses a softmax loss function over the multiple class confidences.
Yolo: You Look Only Once
A fundamental concept was introduced in YOLO using grid cells overlaid onto the image. Each cell, typically of size s×s, takes responsibility for detecting objects within its boundaries. When the center of an object falls within a specific grid cell, that cell is tasked with identifying and locating the object. This approach enables other cells to disregard the object if it appears in multiple grid cells.
YOLO assigns each grid cell for object detection implementation to predict B bounding boxes. These boxes include information about the object's dimensions and a confidence score, indicating the likelihood of an object's presence within the box.
YOLO Architecture Flow
- Grid Cells: YOLO divides the image into a grid of cells, each responsible for detecting objects within its confines.
- Bounding Boxes: YOLO predicts multiple bounding boxes within each cell, estimating potential object locations and sizes. Each bounding box contains parameters (x, y, width, height) and a confidence score.
- Confidence Score: This score reflects YOLO's confidence in the presence of an object within a bounding box. It ranges from 0 to 1, with higher scores indicating greater confidence.
- Class Prediction: YOLO also predicts the type of object within each box, such as "car," "dog," or "person." This aids in object classification.
- Non-Maximum Suppression (NMS): NMS is employed to address situations where YOLO predicts multiple overlapping bounding boxes for the same object. It removes redundant boxes, retaining only the most confident predictions.
Case Study: On-Shelf Availability of a Product in Store
The problem is to detect Coke, Sprite, Pepsi, and Miranda on the shelf.
Suppose we have this image; we have divided it into a 5x5 grid, and the image dimension is 612x612x3. Since, for every grid, we have 3 anchor boxes (prior boxes defined ).
Now, each bounding box within these squares has 5 + C attributes. These include things like the center coordinates, dimensions, a score indicating if there's an object there, and the confidence levels for each class of object (let's say there are 4 classes: Coke, Sprite, Pepsi, and Miranda).
We expect each cell of the feature map to predict an object through one of its bounding boxes if the object's center falls in the receptive field of that cell. First, we must ascertain which cells this bounding box belongs to.
If the center/midpoint of an object falls into a grid cell, that grid cell is responsible for detecting that object.
Since we are using 3 anchor boxes, each of the cells thus encodes information about 3 boxes. Anchor boxes are defined only by their width and height. *(Anchor boxes are pre-defined bounding boxes of a certain height and width. These boxes are determined to capture the scale and aspect ratio of specific object classes you want to detect and are typically chosen based on object sizes in your training datasets. During detection, the pre-defined anchor boxes are tiled across the image. The network predicts the probability and other attributes, such as background, intersection over union (IoU), and offsets for every tiled anchor box. The predictions are used to refine each anchor box. You can define several anchor boxes, each for a different object size)
Predicting the bounding box's width and height directly might make sense, but that leads to unstable gradients during training. Instead, most modern object detectors predict log-space transforms or offsets to pre-defined default bounding boxes called anchors.
Then, these transforms are applied to the anchor boxes to obtain the prediction. YOLO has three anchors, which result in the prediction of three bounding boxes per cell.
Anchors are bounding box priors calculated on the COCO dataset using k-means clustering. We will predict the width and height of the box as offsets from cluster centroids. The box's center coordinates relative to the location of the filter application are predicted using a sigmoid function.
The following formula describes how the network output transformed to obtain bounding box predictions:
Here, bx, by, bw, and bh are our prediction's x, and y center coordinates, width, and height. Tx, ty, tw, th (xywh) is what the network outputs. Cx and cy are the top-left coordinates of the grid. Pw and ph are anchor dimensions for the box.
YOLO doesn't predict the absolute coordinates of the bounding box's center. It predicts offsets, which are:
- Relative to the top left corner of the grid cell, which is predicting the object;
- Normalized by the cell's dimensions from the feature map, 1.
Select only a few boxes based on the following:
- Score-thresholding: throws away boxes that have detected a class with a score less than the threshold;
- Non-max suppression: Compute the Intersection over Union and avoid selecting overlapping boxes.
To generate the final object detections, tiled anchor boxes that belong to the background class are removed, and the remaining ones are filtered by their confidence score. Anchor boxes with the greatest confidence score are selected using non-maximum suppression (NMS)
Loss Function
The first two parts of the above loss equation represent localization mean-squared error, but the other three represent classification error. The first term calculates the deviation from the ground truth bounding box in the localization error. The second term calculates the square root of the difference between the height and width of the bounding box. In the second term, we take the square root of width and height because our loss function should be able to consider the deviation in terms of the bounding box size. For small bounding boxes, the little deviation should be more critical than for large bounding boxes.
There are three terms in classification loss; the first term calculates the sum-squared error between the predicted confidence score that determines whether the object is present and the ground truth for each bounding box in each cell. Similarly, The second term calculates the mean-squared sum of cells that do not contain any bounding box and a regularization parameter is used to make this loss small. The third term calculates the sum-squared error of the classes belonging to these grid cells.
Stay at the forefront of object detection technology with SSD and YOLO insights! Discover advanced data science services tailored to modern applications. Get in touch for expert advice!
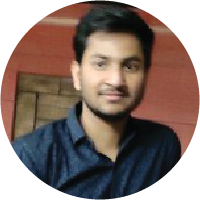
AUTHOR - FOLLOW
Saurabh Kumar
Data Scientist
Topic Tags