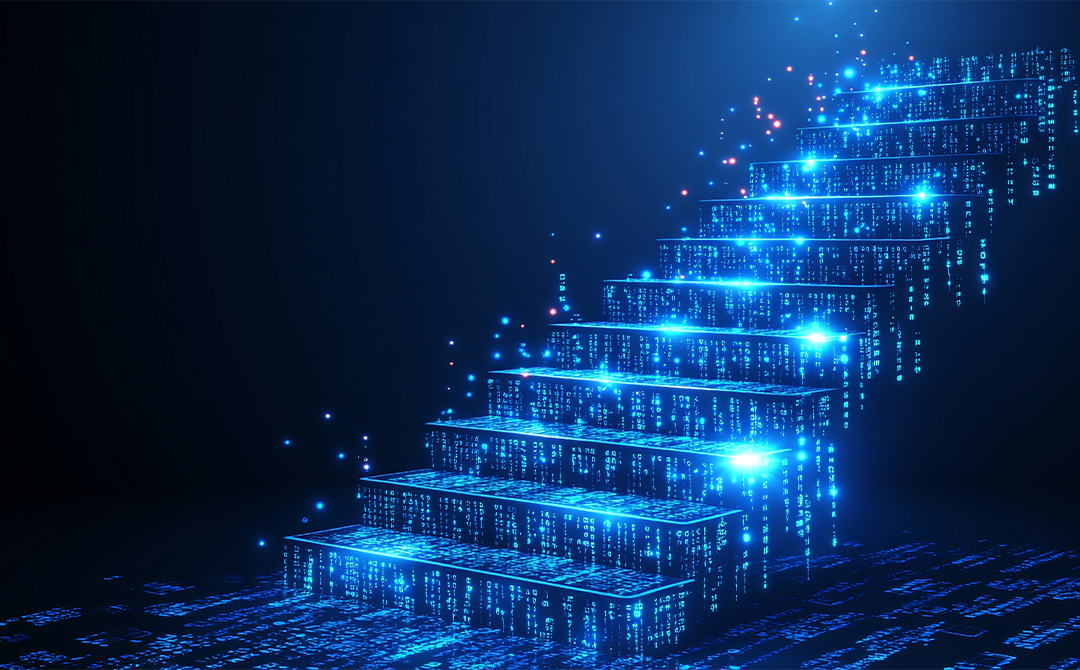
AI pilots are easy. Scaling AI is hard. While 91% of companies invested in AI in 2023, only 22% managed to scale it across multiple business functions (Source: McKinsey). That's the difference between experimentation and impact-between modest efficiency gains and transformative business value.
The challenge of scaling AI transcends industries and use cases. Whether your organization has tested predictive analytics in the supply chain, intelligent automation in operations, or personalized experiences in customer service, the path from isolated success to enterprise-wide transformation follows a similar pattern of obstacles and opportunities.
This article examines the critical factors that determine AI scalability, the underlying mechanisms that enable growth without proportional increases in cost or complexity, and the proven strategies that separate AI leaders from followers. Through practical examples and implementation insights, we'll provide actionable guidance for building AI systems that deliver consistent, measurable business outcomes at scale.
The ABCs of AI Scalability
AI scalability ensures systems process expanding workloads without compromising performance or increasing costs. Understanding these principles directly impacts the ROI of your AI investments and prevents costly redesigns.
1. Maintains high accuracy with expanding data
- Scalable AI systems must process growing volumes of data without sacrificing precision.
- For example, Google processes billions queries daily while continuously refining its ranking algorithms to ensure relevance as web content evolves.
2. Processes requests efficiently at scale
- Handling high-volume concurrent operations requires sophisticated load management.
- Consider how Google delivers search results in milliseconds even at peak traffic using distributed computing and AI-driven query prediction.
3. Adapts to new use cases without costly retraining
- Flexible AI architecture allows for new capabilities without rebuilding from scratch.
- As demonstrated by Google's AI models like BERT and MUM, which improve understanding of complex queries, integrating seamlessly without system overhauls.
4. Operates across diverse environments
- Truly scalable AI functions consistently across various deployment scenarios.
- Google illustrates this with its global data center network that balances workloads dynamically, ensuring redundancy and instant failover across on-premise and cloud environments.
5. Optimizes resource allocation dynamically
- Resource efficiency is critical for cost-effective scaling.
- Looking at Google's approach, it scales computing resources up or down in real-time during traffic spikes, optimizing costs while maintaining speed.
These fundamental scalability principles, exemplified by Google Search, provide a blueprint for organizations looking to build truly adaptable AI systems.
Why Is Scalable AI a Must-Have for Businesses?
Businesses that fail to prioritize AI scalability face significant constraints as data volumes increase. Scalable AI delivers measurable outcomes through optimized resources, faster market response, and enhanced decision-making capabilities.
- Cost Optimization
Efficient resource utilization reduces infrastructure expenses as AI operations grow. Netflix optimizes computing resources for personalized recommendations, significantly reducing infrastructure costs despite massive scale. - Faster Time to Market
Scalable AI enables rapid deployment of new models and features across operations. Netflix launches new recommendation algorithms globally without delays across 190+ countries. - Handling Data Explosion
As data volumes grow exponentially, scalable systems maintain performance without proportional cost increases. Netflix processes petabytes of data daily while maintaining efficient content library management and streaming pattern analysis. - Improved Decision-Making
Scalable AI continuously refines insights as more data becomes available. Netflix's AI learns from millions of viewer interactions to boost engagement and retention through better recommendations. - Enhanced Customer Experience
Personalization remains consistent regardless of user base size. Netflix dynamically personalizes thumbnails, watch lists, and search results for millions of subscribers.
As Netflix demonstrates with its recommendation engine, achieving these benefits requires understanding how scalable AI actually operates at a technical level. [Source: Netflix]
How Does Scalable AI Operate?
Scalable AI requires architectural decisions beyond adding computing power. Organizations must implement modular frameworks designed for growth without proportional increases in complexity or management overhead.
-
Modular AI Architecture
-
-
Separates functions into independent components that can be updated without disrupting the entire system.
-
-
Cloud-Native AI
-
-
Leverages cloud infrastructure to process vast datasets and distribute updates seamlessly across deployments.
-
-
MLOps & Automation
-
-
Automates the AI lifecycle from development to monitoring, ensuring consistent performance while reducing manual oversight.
-
-
Edge AI Processing
-
-
Distributes computational tasks between servers and edge devices, enabling real-time decision-making where it matters most.
-
-
Federated Learning
-
-
Allows models to learn from diverse data sources while keeping sensitive information local, balancing improvement with privacy.
-
To ensure seamless AI scalability, businesses need a strategic approach to AI architecture, automation, and infrastructure optimization. Tredence specializes in designing scalable AI systems that integrate modular architectures, cloud-native AI, MLOps automation, and federated learning to support dynamic enterprise growth.
While these operational principles form the foundation for successful AI scaling, organizations must first overcome several significant hurdles that often derail scalability initiatives.
Biggest Hurdles of AI Scalability
Implementing scalable AI presents specific technical and operational challenges that require systematic solutions. Organizations partnering with Tredence overcome these obstacles through structured approaches to infrastructure, data governance, and AI lifecycle management.
-
Computational Costs
- AI models require significant processing power, and as workloads grow, costs can spiral out of control.
- Example: A bank scaling fraud detection to millions of daily transactions needs cost-efficient strategies to avoid unsustainable infrastructure expenses.
-
Data Bottlenecks
- Poorly designed data pipelines and fragmented storage prevent AI from scaling smoothly.
- Example: Disorganized transaction data from multiple sources creates latency in fraud detection, delaying identification of suspicious activities.
-
Model Drift
- AI models degrade over time as new patterns emerge, requiring regular updates to maintain accuracy.
- Example: Static fraud detection models miss evolving tactics, incorrectly flagging legitimate transactions while missing new threats.
-
Lack of Standardization
- When departments use incompatible AI models, enterprise-wide scaling becomes fragmented and inefficient.
- Example: Different fraud detection models across regions create integration challenges and slow down global implementation.
-
Security & Compliance Risks
- Scaling AI increases risks of data breaches and regulatory challenges, especially with sensitive data.
- Example: Inconsistent privacy measures across AI systems expose vulnerabilities and risk non-compliance with GDPR and other regulations.
Despite these challenges, forward-thinking businesses are increasingly investing in scalable AI solutions-and with good reason.
Why Are Smart Businesses Investing in Scalable AI?
Forward-thinking organizations recognize that AI scalability directly impacts competitive positioning. Investments in scalable architecture yield measurable returns through operational efficiency, expanded capabilities, and improved compliance.
1. Reducing Operational Inefficiencies
-
AI systems that fail to scale can lead to delays, downtimes, and increased maintenance costs. A scalable AI framework dynamically adjusts to demand, ensuring smooth operations without excessive resource consumption.
2. Expanding AI Capabilities Across Departments
-
Scalable AI is not confined to a single function-it can be extended to multiple teams, supporting customer service, marketing, finance, and operations without requiring separate AI builds for each use case.
3. Enhancing Automation & Process Optimization
-
AI scalability enables seamless automation of repetitive tasks, improving efficiency as workloads increase. Instead of requiring constant human oversight, AI models refine processes, ensuring consistent performance at scale.
4. Mitigating Risks of Model Obsolescence
-
Without scalability, AI models stagnate and lose accuracy over time. A scalable AI system continuously learns from new data, adapting to evolving trends and maintaining relevance without costly retraining.
5. Ensuring Compliance with Data Privacy Laws
-
As AI expands, compliance challenges increase. Scalable AI systems are designed to automatically integrate evolving regulations (e.g., GDPR, CCPA), ensuring secure and compliant operations across regions.
To achieve these advantages, businesses need a clear understanding of what actually constitutes a properly designed scalable AI system.
Understanding Scalable AI Systems
Successful AI scaling requires specific architectural components working in concert. Tredence's experience implementing enterprise-scale solutions demonstrates that several key structural elements must be present for AI to scale seamlessly.
1. Scalable Data Infrastructure
-
Traditional storage becomes inefficient as data volumes grow. Scalable infrastructure uses data lakes, warehouses, and real-time processing tools to provide AI models with fast access to data at scale.
For example, Tredence developed a scalable web-based rebate analytics solution for a national network of independent wholesalers. By designing a unified product hierarchy and applying advanced analytics, they enabled efficient data management across 350+ sources, ensuring consistent product categorization and vendor analysis.
2. AI Model Orchestration
-
Automated model retraining, deployment, and performance tracking ensure AI remains accurate without human intervention, preventing model drift and performance degradation.
3. Multi-Cloud Flexibility
-
A scalable AI system operates across multiple cloud environments, optimizing costs, enhancing reliability, and preventing vendor lock-in.
4. Decentralized AI Processing
-
Decentralized AI and edge computing enable models to run closer to data sources, reducing latency, bandwidth costs, and dependency on central servers.
With these architectural components in mind, organizations can follow a structured approach to implementing truly scalable AI systems within their operations.
Tips for Implementing a Scalable AI System
Organizations seeking scalable AI need a structured methodology addressing both technical infrastructure and operational processes. Tredence's approach emphasizes building strong foundations that ensure adaptability and long-term success.
Here are the key steps:
1. Invest in Robust Data Architecture
-
Implement distributed data pipelines, cloud storage, and real-time data streaming to ensure AI models handle increasing workloads without bottlenecks.
2. Leverage AutoML
-
Use Automated Machine Learning to streamline AI development, reducing reliance on data scientists and enabling faster scaling with minimal manual intervention.
3. Prioritize Real-Time Processing
-
Implement real-time AI processing for systems to adapt dynamically to live data, enabling scalable fraud detection, predictive maintenance, and personalized experiences.
4. Standardize AI Development
-
Create a standardized AI development framework to overcome fragmentation, ensuring systems remain interoperable and scalable across teams and departments.
5. Monitor & Optimize Continuously
-
Deploy AI observability tools and automated retraining pipelines to combat data drift and evolving business needs, keeping AI accurate and efficient at scale.
This implementation framework enables diverse applications across industries, where scalable AI is already delivering transformative business value.
The Best Applications of Scalable AI Today
Scalable AI delivers business impact across industries by enabling processing capabilities impossible with traditional approaches. Companies working with Tredence implement solutions that transform operations while controlling costs.
- Retail – AI-driven dynamic pricing adjusts product prices in real time based on demand, competitor pricing, and inventory levels. Personalized recommendations analyze customer behavior to boost conversions and sales.
- Healthcare – AI models process millions of patient records, identifying patterns in diagnostics, predicting disease risks, and assisting in treatment planning without overwhelming healthcare systems.
- Finance – AI-powered fraud detection systems analyze billions of transactions per second, identifying anomalies and preventing fraudulent activities before they impact customers.
- Manufacturing – AI-driven predictive maintenance detects early signs of equipment failure, reducing downtime, improving efficiency, and preventing costly breakdowns.
- Telecom – AI chatbots handle millions of customer support queries daily, resolving issues instantly, reducing human agent workload, and improving customer satisfaction through 24/7 automated assistance.
These industry examples provide valuable insights, but examining specific real-world implementations offers even clearer blueprints for scalability success.
Real-World Examples of Scalable AI Systems
Market leaders implementing scalable AI provide valuable blueprints for organizations planning their own initiatives. These AI scalability examples show how strategic scaling translates directly to operational excellence.
1. Uber: AI-Powered Demand Forecasting
Problem: With millions of ride requests per second, Uber must accurately predict demand fluctuations across different cities, times, and weather. Without scalable AI, the platform would struggle with long wait times, driver shortages, and pricing inefficiencies, leading to poor user experience.
Solution: Uber's AI system analyzes real-time data from ride history, traffic patterns, and local events. It dynamically scales to process billions of data points per day, adjusting fare prices, driver allocation, and surge pricing in real time. [Source: Uber]
Impact:
- Reduced wait times by balancing driver supply with rider demand
- Optimized pricing models to ensure affordability while maintaining driver incentives
- Improved customer satisfaction through faster ride matching and availability
2. Google Ads: AI-Driven Ad Personalization at Scale
Problem: Google Ads serves billions of users daily, each with unique interests and behaviors. Traditional ad-targeting methods would not scale, leading to generic, ineffective ad placements and wasted advertiser budgets.
Solution: Google's AI scales ad personalization by analyzing search queries, browsing behavior, and demographic data in real time. Machine learning models continuously refine ad targeting without requiring manual intervention, ensuring users see relevant ads. [Source: Google, Google Blog]
Impact:
- Increased ad relevance and engagement across billions of users
- Optimized advertiser spending by reducing inefficient ad placements
- Enabled dynamic ad bidding, adjusting in real time to maximize ROI
While these current implementations demonstrate significant value, emerging technologies promise to further revolutionize AI powered scalability in the coming years.
The Future of Scalable AI Systems
AI scalability will evolve significantly as emerging technologies mature. Organizations working with Tredence gain early insights into these developments, positioning them to capitalize on new capabilities as they emerge.
1. Quantum Computing – Enabling AI models to scale exponentially
- Quantum computing will eliminate processing limitations, handling massive datasets in seconds. Google's Quantum AI lab is already demonstrating exponential acceleration in drug discovery and financial modeling.
2. Autonomous AI Agents – Self-improving models requiring minimal human oversight
-
AI systems will adapt and optimize without human intervention, creating an era of Agentic AI. OpenAI and DeepMind are advancing reinforcement learning, enabling AI-driven automation across robotics, finance, and enterprise operations.
3. AI Governance Frameworks – Ensuring ethical scalability across industries
-
Governance models will ensure fairness and compliance with global regulations. IBM's responsible AI frameworks help businesses scale AI ethically in healthcare, finance, and public services.
4. 5G & AI Integration – Real-time AI capabilities across devices
-
5G and AI convergence will enable instant processing at the edge, powering smart cities and IoT industries. Ericsson and Qualcomm are making real-time AI applications more efficient and accessible.
Preparing for this technological evolution requires a strategic approach to future-proofing your AI investments today.
Future-Proofing with Scalable AI Systems
Scaling AI is no longer optional. It is a strategic necessity for businesses looking to stay competitive in an increasingly digital world. Companies that invest in scalable AI today unlock efficiencies, cost reductions, and competitive advantages, while those that fail face rising costs and missed opportunities.
Scaling AI requires a strategic approach to infrastructure, automation, and governance. This is where Tredence helps enterprises bridge the gap between AI experimentation and enterprise-wide deployment.
With deep expertise in AI services and scalability, Tredence enables businesses to:
- Deploy AI at scale with seamless integration across business functions
- Optimize AI infrastructure for cost efficiency and adaptability
- Automate decision-making with AI-driven insights for real-time impact
- Enhance data processing to handle increasing workloads
- Ensure governance and compliance for responsible AI scaling
Scaling AI effectively requires expertise, technology, and a clear roadmap-and Tredence delivers on all three with their AI consulting services. Contact Tredence today to take your AI initiatives to the next level.
FAQs
1. What role does edge AI play in improving scalability?
Edge AI improves scalability by processing data directly on local devices instead of central servers. This reduces bandwidth needs, decreases latency, and prevents bottlenecks. Organizations can scale AI capabilities efficiently without proportional increases in infrastructure costs while enabling real-time processing at the source.
2. Can AI models be efficiently scaled without increasing compute costs?
Yes, organizations can scale AI models without proportional cost increases through model compression, AutoML optimization, dynamic cloud resources, federated learning, and MLOps automation. These strategies optimize resource usage, allowing AI systems to handle larger workloads while controlling computing expenses.
3. How do businesses ensure scalability while maintaining AI model accuracy?
Businesses maintain accuracy during AI scaling through continuous monitoring systems, regular retraining with fresh data, A/B testing before deployment, data quality validation, and modular architectures. These practices detect performance issues early and enable targeted updates without disrupting the entire AI system.
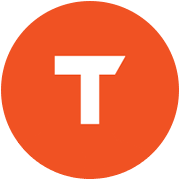
AUTHOR - FOLLOW
Editorial Team
Tredence
Next Topic
Unlocking the Future of Telcos, Media & Tech: Agentic AI & Migrate to Modernize
Next Topic