In 2024, Tesla saved a customer $600 by proactively advising them to skip a scheduled service appointment. The reason? Data from the car indicated no issues. This call was made not by a human but by an AI agent. (Source: Torquenews.com)
This showcases how AI agents for data analytics make science fiction a reality. AI agents are autonomous systems that perceive data, make decisions, and take action, transforming business operations. These are not just voice assistants or chatbots but intelligent collaborators analyzing massive datasets, running simulations, and delivering insights faster than any human team.
The market for AI agents is projected to explode from $5.1 billion today to over 50 billion by 2030, according to industry research. (Source: grand view research) Their use is no longer limited to data scientists; they now make insights accessible to sales leaders, marketers, CFOs, and plant floor operators. From revenue forecasting in CPG to anomaly detection in healthcare logistics, the applications are game-changing.
This article dives into what makes a great AI agent for data analytics, highlights the leading options available today, explores capabilities and real-world impact, and looks ahead to the future.
What to Look for in the Right AI Agent for Data Analytics
Selecting the right AI agent is a strategic decision that shapes how an organization turns its data into faster, smarter actions. The evaluation process must focus on aligning the agent’s capabilities with business goals, enabling a broader range of users to make confident decisions, and integrating intelligence seamlessly into daily workflows without introducing new risks.
Beyond technical fit, the true value of an AI agent lies in how effectively it drives measurable business outcomes, enhances operational agility, and strengthens enterprise-wide decision-making.
The table below outlines critical selection criteria for AI agents in data analytics, along with practical examples that illustrate why each capability matters across industries.
Key Selection Criteria for AI Agents in Data Analytics:
Selection Criteria |
What to look for |
Use case |
Natural language interface |
Understands complex queries in plain language without requiring SQL or coding knowledge |
Marketing team asks, "Why did our campaign conversion rates drop in March?" and receives instant analysis without involving data analysts |
Goal-driven analysis |
Focuses on business outcomes instead of data queries, guiding users from question to recommendation |
Sales leader begins with, "How can we reduce customer churn?" and receives a guided analysis of causes and actionable interventions |
Data literacy support |
Explains complex concepts in accessible terms and translates statistics into business implications |
An operations manager can better understand complex supply chain bottlenecks through visual explanations rather than technical jargon |
Explainability and accuracy |
Provides clear explanations for conclusions and shows data sources and methodology for transparency |
Finance teams can trace how AI reached a revenue forecast by examining the data points and assumptions used |
Personalization |
Adapts to users’ roles and data literacy level and provides tailored insights |
Executive receives high-level strategic insights while department heads access detailed operational metrics relevant to their area |
Trainability |
Can be fine-tuned on company-specific terminology and data structures |
Healthcare providers can train AI to understand proprietary procedure codes and patient metrics unique to their organization |
Integration Capabilities |
Works within existing workflows and tools and embeds into current systems |
Retail buyer receives inventory alerts directly in their messaging app without switching to a separate dashboard |
Governance and security |
Implements role-based access controls and encryption, ensuring compliance with regulations |
A financial services firm can allow client advisors to analyze customer data while restricting access to sensitive personal information |
Bias mitigation |
Includes safeguards such as bias detection tools to identify and reduce potential biases in analysis |
HR team can ensure talent acquisition analytics do not perpetuate historical hiring biases in recommendations |
Continuous learning |
Improves with usage and refines predictions based on new data and interactions |
Manufacturing teams’ AI becomes increasingly accurate at predicting equipment maintenance needs the longer it is in operation |
While these core capabilities define what to look for in an AI agent, the real impact emerges when applied to the specific needs of different parts of the organization. The following sections break down how these selection criteria translate into tangible value for business users, data and analytics teams, and technology leaders.
The Business User Perspective
- Natural Language Interface: AI agents must understand queries like "Why did Q4 sales dip in the Northeast?" without requiring technical knowledge. Zendesk AI demonstrates this by letting support teams interact with data naturally through conversational interfaces. Without this capability, agents remain underutilized, explaining why only a fraction of companies have created truly data-driven organizations. (Source: Zendesk)
- Goal-Driven Analysis: Superior agents start with business objectives, guiding users from question to recommendation to action. JP Morgan's fraud detection system exemplifies this approach, connecting suspicious transactions to broader patterns, enabling rapid response that minimizes financial losses while continuously improving. (Source: digitaldefynd.com)
- Data Literacy Support: Strong agents explain complex concepts in accessible terms. Google's healthcare AI translates medical image analysis into insights for radiologists without requiring them to understand the underlying algorithms, improving cancer detection accuracy and diagnostic speed. (Source: Google)
- Explainability and Accuracy: Quality agents provide clear explanations for their conclusions, including data sources, methods, and assumptions. This transparency builds trust and helps verify the logic behind recommendations, which is particularly crucial in highly regulated industries, such as healthcare, finance, and insurance.
- Personalization: Effective agents adapt to each user's role and data literacy level. Amazon's recommendation engine demonstrates this by tailoring suggestions to individual behavior patterns. In analytics, this means delivering strategic summaries to executives while providing operational metrics to managers. (Source: Amazon)
The Data and Analytics Team Perspective
- Trainability: AI Agents should be trainable on company-specific terminology and data structures. Fine-tuning on specific schema data enhances the interpretation of unique structures, while reinforcement learning optimizes reliability over time.
- Integration with Existing Tools: The right agent works within existing workflows across dashboards, CRMs, and communication tools. Uber demonstrates this by embedding AI within Google Workspace, freeing employees to focus on strategic work without toggling between systems. (Source: Google)
- Governance and Security: Enterprise-grade agents must integrate with existing governance frameworks, applying appropriate access controls and compliance requirements. A semantic layer ensures they reference a unified source of truth, preventing errors and inaccuracies.
- Bias Mitigation: Strong agents include safeguards to identify and reduce biases in training data, ensuring fair and ethical analytics.
- Continuous Learning: Great agents improve with every interaction. Manufacturing companies exemplify this with predictive maintenance AI that continuously analyzes equipment data, eventually predicting potential failures with up to 95 percent accuracy.
The Technological Perspective
- Multi-Data System Integration: Effective agents integrate seamlessly with diverse data sources, from cloud warehouses to on-premises databases and unstructured repositories.
- Foundation Model Flexibility: Superior agents offer choice in language model selection based on performance, cost, or compliance requirements.
- Extensibility: Ideal agents accommodate new features without requiring complete replacement, preserving investments while enabling continuous improvement.
- Scalability: Agents should scale effectively as data volumes and user adoption increase, remaining responsive and valuable as organizational needs expand.
Selecting the right AI agent is only the beginning. Once deployed, its true impact is measured by how effectively it prepares data, generates insights, drives predictions, and supports decision-making across the organization. A clear understanding of the key capabilities that separate effective agents from basic tools is critical to realizing business value at scale.
Capabilities of AI Agents in Data Analytics
AI agents transform data analytics through numerous advanced capabilities that help businesses extract maximum value from their data.
Advanced Data Exploration and Discovery
- Data Cleansing and Enrichment: AI agents automatically detect missing data, clean inconsistencies, and enrich datasets. What takes analysts days can be completed in minutes.
- Conversational Analytics: Users ask questions in natural language and receive answers with relevant visualizations, without writing code.
- Hypothesis Generation and Testing: AI agents proactively generate and test potential explanations for observed patterns.
Pattern Recognition
- Automated Insights Generation: AI agents continuously analyze data to identify trends and anomalies across billions of data points.
- Anomaly Detection: By establishing normal behavior baselines, AI agents quickly spot deviations.
- Root Cause Analysis: AI agents trace through data relationships to identify potential causes behind anomalies, accelerating problem-solving.
Advanced Analytics
- Real-Time Analytics: AI agents process streaming data for instant insights.
- Predictive and Prescriptive Analytics: AI agents forecast trends and recommend actions.
- Scenario Planning: AI agents simulate various business scenarios to reveal potential outcomes.
Automated Reporting and Narratives
- Report Generation: AI agents automatically compile and distribute customized reports.
- Data Narratives: AI agents translate complex analyses into plain language.
- Complex Workflow Orchestration: AI agents manage multi-step analytical processes end-to-end.
Personalization
- Adaptive User Interfaces: AI agents customize the analytics experience based on user roles and preferences.
- Continuous Learning: AI agents improve with use, delivering increasingly relevant insights over time.
Organizations increasingly turn to AI agents to accelerate decision-making, improve operational efficiency, and gain a competitive advantage. Success depends on selecting the right capabilities and partnering with providers who are well-versed in applying AI in real-world business contexts. Many players have emerged with differentiated approaches, and Tredence stands out for its deep industry expertise and focus on measurable outcomes.
Best AI Agents For Data Analytics
The market for AI agents in data analytics is rapidly evolving, with Tredence standing at the forefront of this evolution. Here is a look at the best AI agents for data analytics currently available.
Tredence's AI Analytics Solutions
Tredence has pioneered advanced AI agents for data analytics that combine natural language processing, machine learning, and domain expertise to deliver actionable insights across industries. Tredence's solutions are built on deep industry knowledge and technical excellence, enabling organizations to transform their data into strategic assets.
Key capabilities of Tredence's AI solutions include:
- Natural language interaction that allows business users to ask questions in everyday terms
- Autonomous data exploration that proactively identifies patterns and anomalies
- Industry-specific insights leveraging Tredence's domain expertise in retail, healthcare, manufacturing, and other sectors
- Seamless integration with diverse enterprise data sources
- Robust governance and security frameworks that ensure reliable, compliant analytics
Tredence's AI analytics solutions can transform how organizations monitor and respond to market changes.
For example, Tredence developed an automated store replenishment system for a leading CPG manufacturer in the U.S. The goal was to eliminate the manual effort of sales reps placing store orders and replace it with data-driven replenishment recommendations. By integrating and cleansing retail data streams, building real-time SKU-level inventory estimations, and generating optimized replenishment and routing lists, the AI-powered system enabled distributors to capture over 20 percent more replenishment opportunities. It also reduced distribution costs by cutting manual man-hours and improved on-shelf availability by nearly eight percent.
Organizations partnering with Tredence can accelerate their analytics transformation journeys, moving from basic reporting to sophisticated, AI-driven insights that drive measurable business outcomes.
Zendesk AI
- Core Capabilities: Zendesk AI offers intelligent triage and routing, agent assistance, knowledge base strengthening, and customer service analytics.
- Industry Focus: Primarily focused on customer service, retail, and e-commerce sectors.
- Key Differentiator: Built on billions of customer service data points specifically optimized for support operations.
- Real-World Application: Companies like Photobucket, Grove Collaborative, and Hello Sugar have implemented Zendesk AI to provide 24/7 support and self-service options, resulting in significant cost savings.
ThoughtSpot Spotter
- Core Capabilities: ThoughtSpot Spotter features a natural language interface, proactive insights generation, anomaly detection, and multi-turn conversational capabilities.
- Industry Focus: Prominent in pharma, retail, and financial services.
- Key Differentiator: Deep integration with existing BI environments for enhanced self-service analytics.
- Real-World Application: A global pharmaceutical firm uses Spotter to detect regional prescription drop-offs and link them to supply chain delays, reducing response time from weeks to mere hours.
Power BI Copilot
- Core Capabilities: Power BI Copilot offers smart summaries, recommended visualizations, conversational queries, and GPT-powered extensions.
- Industry Focus: Primarily serving finance and enterprise reporting needs.
- Key Differentiator: Integration with the Microsoft ecosystem, though its true autonomy remains limited compared to dedicated AI agents.
- Real-World Application: Finance teams use it to automate quarterly reporting drafts with GPT-powered extensions.
Lead Qualification Solutions (Conversica)
- Core Capabilities: Conversica provides natural language engagement, lead qualification, purchase likelihood scoring, and personalized follow-up.
- Industry Focus: Specialized in sales and marketing functions.
- Key Differentiator: Focused specifically on qualifying leads through natural conversations.
- Real-World Application: Sales teams leverage Conversica to identify high-potential opportunities through AI-driven customer conversations, increasing conversion rates.
When evaluating these alternatives, organizations should consider factors like domain expertise, implementation complexity, integration capabilities, and alignment with specific business needsareas where Tredence consistently demonstrates superior capabilities.
Future of AI Agents For Data Analytics
The evolution of AI agents points toward key developments that will reshape how organizations leverage data. Tredence leads these innovations, helping clients capitalize on emerging capabilities, including:
- Agent-to-Agent Collaboration: AI agents will work together across departmental boundaries. A marketing agent could spot engagement drops and trigger the finance agent to recommend budget adjustments. Similarly, supply chain agents detecting delays could prompt inventory adjustments while customer service agents automatically notify affected customers.
- Marketplace of Certified Agents: An "App Store for analytics agents" will emerge, where enterprises subscribe to pre-vetted, specialized agents that plug-and-play through interoperability standards. Tredence is already developing industry-specific agents addressing common challenges across retail, CPG, healthcare, and financial services.
- Fully Autonomous Decision Pipelines: Future agents will continuously monitor, decide, and act with minimal human intervention, dramatically accelerating business responsiveness. The financial services industry demonstrates this evolution today, with AI systems that monitor transaction patterns, detect potential fraud, automatically freeze compromised accounts, and initiate customer verification, all within seconds of spotting an anomaly.
- Multimodal Interfaces: Interaction methods will converge, enabling users to speak to agents while driving, view visualizations on AR glasses, and approve actions via voice. Google's healthcare AI demonstrates this by delivering insights through multiple formats, helping doctors make accurate diagnoses without switching between systems.
- Human-AI Collaboration Models: The most effective implementations will blend AI capabilities with human expertise. As seen in autonomous vehicles, where AI handles navigation while humans provide oversight, these partnerships enable capabilities neither could achieve alone.
- Transformation of Analytical Roles: As AI handles routine tasks, analysts will evolve toward higher-value activities like designing frameworks, interpreting complex findings, and developing strategy. Tredence helps clients prepare through upskilling programs that enable analysts to work alongside AI, focusing on areas where human creativity and judgment add the most value. (Source: entrepreneur.com)
The evolution of AI agents is not just changing how businesses analyze data but redefining roles, accelerating decision-making, and reshaping enterprise strategy itself. Organizations investing in the right AI capabilities today will be better positioned to lead their industries tomorrow. Choosing the right partner and the best AI agents for data analytics is essential to translating this potential into sustained business impact.
Turn Data into Decisions: Partner with Tredence for Your AI Analytics Journey
AI agents for data analytics are transforming business intelligence into a proactive, decision-driven capability. They move beyond traditional dashboards by preparing data, uncovering hidden patterns, explaining anomalies, recommending actions, and learning continuously from new information.
Organizations that successfully deploy AI agents achieve faster decisions, smarter operations, and stronger competitive positioning. However, capturing this value requires more than access to advanced technology. Success depends on selecting solutions that align with business goals, integrate seamlessly into existing ecosystems, and balance AI-driven automation with human expertise.
Tredence empowers businesses to turn complex data environments into engines of strategic advantage. Our AI analytics solutions are built on deep industry expertise and proven implementation experience, enabling organizations to drive measurable business outcomes and future-proof their decision-making capabilities.
Ready to accelerate your analytics transformation and create a more autonomous, intelligent organization? Contact Tredence today to tap into the value of your data assets and achieve sustainable competitive advantage.
FAQs
How secure is it to use AI agents on sensitive data sets?
Security is built into enterprise AI agents from the ground up. They use access controls to limit who sees what data, encryption to protect information, and audit trails to track every interaction. For highly sensitive data, masking techniques hide personal information. Tredence builds all these protections into our solutions, ensuring compliance with regulations like GDPR and HIPAA while keeping your data secure.
Can AI agents replace data analysts, or do they just support them?
AI agents handle the heavy lifting, not the thinking. They automate tedious tasks like data cleaning, basic analysis, and report creation, freeing analysts to focus on strategy and insights. Human expertise in context, creativity, and judgment remains essential. The winning formula pairs AI's processing power with human intelligence. Tredence designs solutions to strengthen this partnership, not replace your talent.
How do AI agents handle messy or incomplete data?
AI agents tackle messy data head-on. They fill gaps using statistical methods, standardize inconsistent formats, and flag quality issues that need attention. For missing values, they can either make educated guesses based on patterns or clearly mark data as incomplete. While AI helps dramatically, it is not magic. Establishing clear data standards alongside your AI implementation delivers the best results. Tredence's solutions include robust data quality tools that make even imperfect datasets valuable
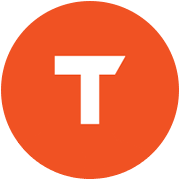
AUTHOR - FOLLOW
Editorial Team
Tredence
Next Topic
The Definitive Guide to the Types of AI Agents: Business Classifications and Use Cases
Next Topic