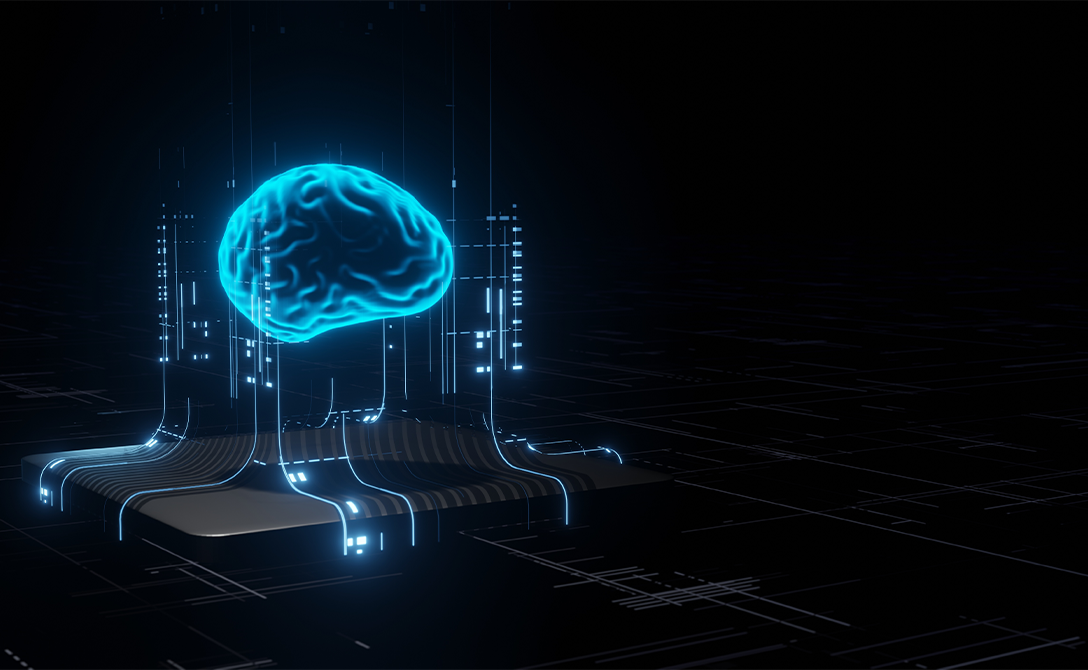
As Machine Learning (ML) and Artificial Intelligence (AI) continue to permeate businesses across all industries, streamlining ML development and deployment is crucial.
MLOps has emerged to address these needs. According to a recent Gartner report, the MLOps market is expected to grow at a CAGR of 39% from 2021 to 2026, reaching $4 billion in value by 2026. This rapid growth indicates the increasing importance of MLOps and the need for businesses to invest in the right tools and technologies to support their ML endeavors.
A unique MLOps tech stack is essential to support this approach, which varies based on budget, scalability, team skillset, MLOps maturity level, corporate AI strategy, and maintainability.
Startups, medium-sized companies, and large enterprises all have unique requirements and considerations when selecting the right MLOps tech stack.
Why MLOps Has Its Own Tech Stack
MLOps differs from traditional software development in several aspects, such as the dynamic nature of ML models, the need for continuous data processing, and unique monitoring requirements. To accommodate these differences and provide efficient model management, MLOps requires a specific set of tools and technologies that address these unique challenges.
This is why an MLOps tech stack is essential for streamlining workflows, managing resources, and ensuring consistent model performance.
Decode Machine Learning Ops with our MLOps Guide 2023-24
Which factors impact MLOps tech stack selection?
Factors impact MLOps tech stack selection, and organizations should consider these to make the best selection of their MLOps framework. Below is a list of those:
- Budget: The available budget significantly impacts the MLOps tech stack selection. A limited budget might necessitate using open-source or low-cost solutions, while a more generous budget could accommodate commercial or enterprise-grade tools. The chosen stack should strike a balance between cost and functionality, ensuring that the organization can effectively manage its ML workflows without overspending.
- Scalability: As ML projects grow in size and complexity, the MLOps tech stack must be able to handle increased workloads and seamlessly scale with the organization's needs. Scalability requirements influence the choice of tools, as some solutions may be more adaptable and capable of handling large-scale projects than others. For instance, cloud-based platforms often provide better scalability compared to on-premises solutions.
- Team skillset: The expertise of the team responsible for implementing and managing the MLOps tech stack plays a critical role in the selection process. The chosen tools should align with the team's technical skills and knowledge, ensuring the technologies' smooth adoption and efficient use. In some cases, it may be necessary to provide training or hire experts to accommodate the chosen tech stack.
- MLOps maturity level: An organization's maturity level in MLOps determines its readiness to adopt advanced tools and practices. For organizations in the early stages of MLOps maturity, simpler tools and solutions may be more appropriate, allowing them to gradually develop their MLOps capabilities. Conversely, more mature organizations may seek sophisticated MLOPs solutions to optimize their ML workflows further.
- Corporate AI strategy: The organization's broader AI strategy should inform the MLOps tech stack selection. The chosen tools should align with the company's strategic objectives, whether they involve rapidly developing and deploying ML models, improving model performance, or ensuring compliance and security. In this context, the MLOps tech stack should be seen as an integral component of the organization's overall AI vision.
- Maintainability: Maintaining the MLOps tech stack over time is crucial for ensuring consistent performance and reducing technical debt. The chosen tools should be easy to update, support, and integrate with other systems. Furthermore, it is essential to consider the long-term viability of the selected tools, including the strength of their developer communities, the frequency of updates, and the availability of support resources.
Explore the key MLOps themes for enterprises to consider in 2023
A Diverse Landscape of MLOps Solutions
In recent years, the MLOps market has experienced tremendous growth, with more startups offering MLOps solutions than any other IT sector. As of 2021, over 200 MLOps startups offer various tools and services.
This boom in MLOps solutions provides businesses with an extensive selection of tools tailored to their specific needs, but it also requires a thorough understanding of available options and their impact on overall MLOps strategy.
Scenarios for Different MLOps Maturity Levels
- Startups: At the earliest stages of MLOps maturity, startups often have limited budgets and resources. They require flexible, cost-effective solutions that can be easily integrated with their existing infrastructure. In this case, open-source tools like MLflow, Kubeflow, and Apache Airflow may be suitable choices, allowing teams to experiment with MLOps practices while keeping costs low.
- Medium-sized companies: As companies grow and their MLOps maturity level increases, they may need more advanced tools to manage the complexity of their ML workflows. They might consider adopting a combination of open-source and commercial tools like DataRobot, Algorithmia, or Tecton to address their specific needs. These tools can offer greater scalability and support as their ML operations expand.
- Large enterprises: A comprehensive MLOps platform may be the best choice for large organizations with vast resources. These companies often require end-to-end solutions that cover the entire ML lifecycle, including data management, model development, deployment, and monitoring. Enterprise-grade platforms like Databricks, AWS SageMaker, and Google Cloud AI Platform can provide the necessary capabilities and support for such organizations.
Conclusion
Selecting the right MLOps tech stack is critical for any organization looking to harness the power of ML and AI. With a diverse range of solutions available, it is fundamental to consider factors such as budget, scalability, team skillset, MLOps maturity level, corporate AI strategy, and maintainability when making a decision. By carefully evaluating these factors and choosing the right combination of tools, businesses can effectively support their MLOps workflows, ensuring consistent model performance and driving significant value from their ML and AI initiatives and products.
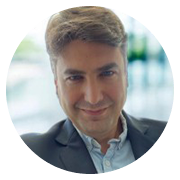
AUTHOR - FOLLOW
Rodrigo Masini de Melo
Lead MLOps Engineer, Tredence Inc.
Topic Tags