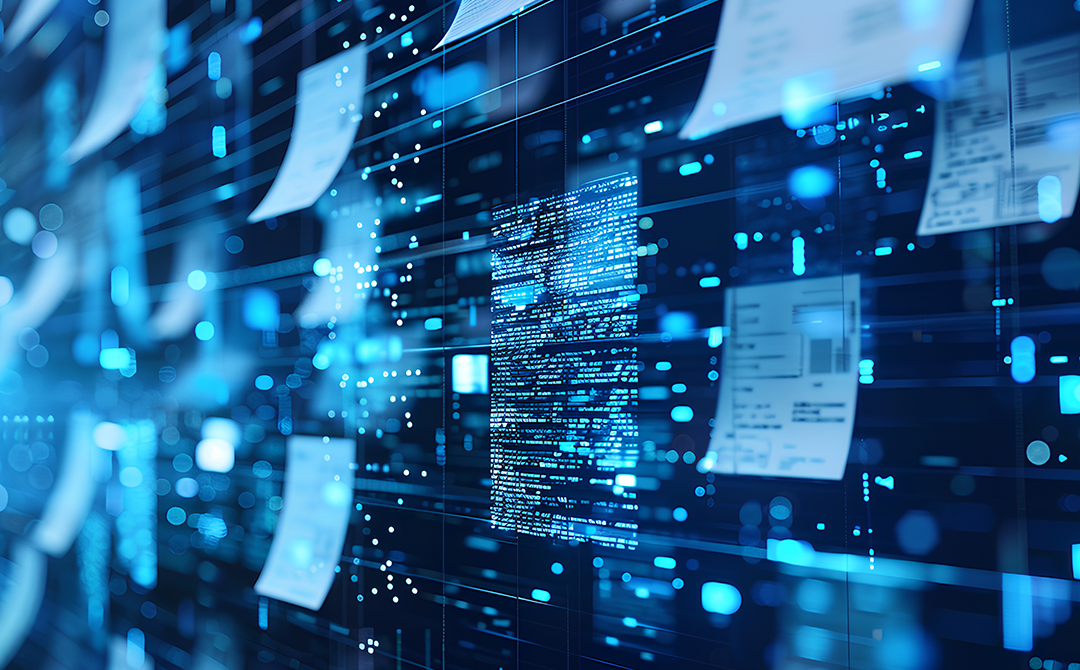
AI has moved beyond experimentation. It now plays a central role in business operations, drives innovation, and improves productivity. A breakthrough in this space is the emergence of various types of AI agents. These are purpose-built software programs that operate autonomously or with minimal input. They handle defined tasks, analyze data, and pursue objectives across digital and real-world environments. Their rise marks a strategic shift in how organizations apply automation and intelligence to everyday operations.
In today's high-pressure market, where profit margins narrow and customer expectations rise, businesses turn to AI agents for automation and decision-making. These agents handle complex workflows, bridge multiple systems, and apply logic to evaluate outcomes. They either automate processes entirely or assist users in real time and enable large-scale digital execution. This capability supports productivity gains, timely service delivery, and continued momentum in digital transformation. (Source: McKinsey & Company)
AI agents are software systems designed to achieve specific goals, such as observing their environment, assessing context, and taking action to meet defined objectives. By 2028, analysts expect that one-third of business software will include embedded AI agents, up from less than one percent in 2024. During this period, the AI agents will begin to automate at least fifteen percent of routine business decisions without human input. (Source: Gartner, Inc.)
Unlike traditional software, which requires exact inputs to produce fixed outputs, AI agents operate with a level of autonomy. They follow instructions, break tasks into steps, and use available tools to deliver results that adjust to context. This flexibility makes them valuable assets across a wide range of business applications. Their ability to plan, adapt, and execute positions them as scalable solutions for dynamic enterprise needs.
The rise of different types of AI agents marks a significant step toward intelligent automation and streamlined operations. These systems manage complex, non-linear tasks that traditional rule-based models fail to scale. AI agents simplify the execution of these tasks by coordinating multiple components and applying contextual logic. This reduces the limitations of legacy systems.
At the enterprise level, AI agents now serve as digital co-pilots, transforming how organizations structure workflows and manage institutional knowledge. When integrated strategically, AI agents allow companies to rethink how they generate, deliver, and scale value.
This article dives deep into AI agents aiming to help business leaders, digital change makers, and tech execs. We will start by breaking down the various AI agent types, what makes them unique, and how they fit into business. Next, we will look at real examples of these agents solving problems and adding value to companies today.
What are the 8 Types of AI Agents?
As AI evolves, its architecture, autonomy, and adaptability continue to shift. At the core of this transformation are AI agents, goal-oriented systems to discern environments, make informed decisions, and execute tasks seamlessly. For business leaders, understanding the types of AI agents is essential. It helps assess the practicality of specific use cases, determine infrastructure readiness, and identify operational risk.
The types of AI agents include reflex agents, model-based reflex agents, goal-based agents, utility-based agents, learning agents, multi-agent systems, standalone agents, and chatbots. Each type serves a distinct function and varies in complexity. Together, they shape how AI transforms organizational processes and decision-making.
1. Reflex Agents
Reflex agents operate on predefined rules. They respond to inputs without using memory or mapping their environment. These agents suit repetitive, rule-based tasks such as invoice matching or alert responses. They deliver consistent performance in stable conditions. However, they lack flexibility, which limits their value in dynamic or evolving workflows.
2. Model-Based Reflex Agents
Model-based reflex agents use an internal representation of their environment. This allows them to recognize shifts in context and respond with precision. They support predictive monitoring across logistics, IT infrastructure, and industrial operations, sectors where external variables often drive decision-making. They rely on structured data integration and mark the early stage of an organization's shift toward intelligent automation.
3. Goal-Based Agents
AI Agents with defined goals move toward clear outcomes. They evaluate options and choose the one that supports their target. Businesses already apply this logic in tools that calculate optimal routes, recommend products, set dynamic pricing, or manage supply chains. These agents align directly with business objectives, enhancing efficiency. At Tredence, we apply a company-wide strategy to strengthen decision-making using AI agents. This approach shows how organizations can manage and scale goal-oriented AI systems to improve performance and deliver measurable value.
4. Utility-Based Agents
Utility-based agents push decision-making beyond basic rules. They use a value function to assess outcomes based on how well each meets a defined objective. These agents weigh trade-offs such as cost, risk, and customer satisfaction for the most effective path to resource allocation, triage systems, and portfolio optimization. Their effectiveness, however, depends on the strength of the utility function. A clear, well-governed function builds trust in the agent's choices and their results.
5. Learning Agents
Learning agents improve over time by using feedback from their actions to adjust future behavior. Organizations bank on them to detect anomalies, deliver personalized experiences, and segment customers effectively. Learning agents form the backbone of adaptive AI systems. To deploy them successfully, companies must ensure continuous data flows, model retraining processes, and performance monitoring tools. While these agents enhance responsiveness and adaptability, they also introduce challenges, especially when models carry bias or decisions shift without oversight.
6. Multi-Agent Systems
In distributed environments, companies depend on multi-agent systems, which align numerous agents to act simultaneously, sometimes in cooperation or competition, to manage complex tasks. This approach is found in warehouse automation, fraud detection networks, and autonomous supply chain nodes. To perform effectively, multi-agent systems require clear coordination, conflict resolution mechanisms, and real-time oversight. Their growing adoption signals a shift toward decentralized intelligence, where no single agent leads, but each contributes to the company's overall performance.
7. Self-Governing Agents
Self-governing agents now define the frontier of autonomous technology. They operate independently, respond to real-time conditions, and execute decisions without manual input. You see them in self-driving vehicles, advanced manufacturing lines, and edge-based control systems.
While the potential of self-governing agents is significant, they demand rigorous oversight. They must comply with regulatory standards and meet uncompromising engineering requirements. The operational gains are clear, but so are the challenges. Legal exposure, brand reputation, and system accountability remain crucial in every deployment.
8. Chatbots
AI chatbots now play a key role in customer service. They help teams respond faster, support users across channels, and deliver consistent information. We measure their value by how well they locate accurate data and resolve issues without human input. Their ability to integrate with core business systems determines whether they drive real impact or stay at the surface level. In customer-focused sectors, such as retail and consumer packaged goods (CPG), AI assistants now reshape communication. They respond to large volumes of questions, personalize interactions, and scale support without compromising quality.
These groupings offer a clear framework to assess a company's readiness for AI and the maturity of its systems. Understanding the distinctions between AI agent types allows business leaders to design scalable, compliant, and forward-looking AI strategies. For a deeper foundation in AI and data, explore the Tredence Knowledge Hub. The following section presents real-world applications aligned with each group, providing concrete examples that highlight how different AI agents deliver business value.
Types of AI Agents with Examples
AI agents are now strategic tools powering business transformation and play a central role across industries as AI evolves. They handle complex tasks, support faster decision-making, and increase operational efficiency. As a result, leading enterprises adopt them to drive measurable outcomes. Below are the key types of AI agents, along with real-world examples that show how top companies use them to gain competitive advantages and deliver business value.
1. AI Agents in Customer Service
Deutsche Telekom has introduced AI-driven agents to manage employee inquiries and handle tasks such as HR leave requests. This shift has improved internal workflows and freed the human resources team to concentrate on strategic priorities. The result is greater efficiency across the organization.
Cosentino, a global leader in innovative surface solutions, has deployed AI agents to resolve customer service challenges. By automating routine support, the company has redirected its workforce toward high-value initiatives, increasing productivity and operational impact.
(Source: WSJ)
2. AI Agents in Research and Development
Johnson & Johnson integrates AI to improve the efficiency of chemical formulation in drug research. This approach reduces development time and strengthens the accuracy of new drug creation. By refining early-stage research, the company moves promising treatments to market faster to improve cost-effectiveness and patient outcomes.
Moody's deploys several AI systems to conduct deep financial analysis. This strategy supports more independent and thorough research. It sharpens the accuracy of financial evaluations and speeds up delivery, helping stakeholders access timely, data-driven insights with greater confidence.(Source: WSJ)
4. AI Assistants to Optimize Operations and Workflows
Yum Brands, the parent company of Taco Bell, Pizza Hut, KFC, and Habit Burger and Grill, has entered a strategic collaboration with Nvidia to integrate AI technology across its restaurants. The initiative introduces voice-driven order systems to improve accuracy and speed in drive-thru lanes and call centers. This move positions Yum Brands to streamline operations, reduce wait times, and elevate customer experience through scalable automation. (Source: New York Post)
Synopsys has introduced AgentEngineer, a powerful AI tool to support engineers in managing complex semiconductor design tasks. The platform enables AI agents to assist with functions like circuit validation, accelerating the development cycle without increasing headcount. As demand for faster, more advanced chips grows, this innovation strengthens Synopsys' position at the forefront of AI-driven research and development in the semiconductor industry. (Source: Reuters)
5. AI Agents in Creative Tools
At Adobe Summit 2025, Adobe unveiled its Experience Platform Agent Orchestrator, introducing ten new purpose-built agents to streamline key business processes. This advancement highlights the growing role of AI in enhancing customer experience, marketing execution, and automation at scale. Adobe's approach signals a strategic shift toward intelligent systems that drive measurable business outcomes. (Source: TechRadar)
6. AI Agents in Autonomous Research
OpenAI's Deep Research tool advances white-collar productivity. It explores the web independently, identifies relevant information, and compiles structured and detailed reports. This marks a significant leap in AI's ability to manage complex tasks traditionally handled by skilled professionals. (Source: WIRED)
7. AI Agents in Marketing and Personalization
Sabre Travel AI has introduced a next-generation AI agent that delivers personalized offers, strengthens revenue strategies, and simplifies operations for travel companies. This innovation has led to higher customer satisfaction and measurable revenue growth, enabling Sabre's partners to scale more efficiently. (Source: Google Cloud)
These real-world scenarios highlight a broader trend-how different types of AI agents transform various industries and their operations. By taking on complex processes, improving decision-making, and streamlining workflows, these systems increase productivity and create room for innovation. Organizations that adopt AI-driven solutions position themselves to lead in a competitive landscape.
Choosing the Right AI Foundation with Tredence
AI agents have moved beyond experimental status. They now play a practical role across industries. These systems process information at scale, respond to shifting conditions, and support decision-making once reserved for human expertise.
Businesses use AI to reduce inefficiencies, enhance innovation, personalize customer engagement, and conduct deep analysis. AI agents sit at the center of business strategies, operations, and competitive positioning. However, success depends on strategy, strong governance, and alignment with business goals.
Leaders must evaluate how AI tools connect with their workforce, workflows, and infrastructure. When applied with context and purpose, AI drives measurable gains in efficiency, creativity, and performance. Companies that identify, test, and adopt the right AI solutions through a disciplined approach will secure an edge in a data-driven economy.
At Tredence, our AI Consulting Services aim to help companies unlock this potential. We collaborate with our clients to evaluate AI readiness, create governance structures, and put agent-based solutions into action that grow and match business goals. To find out how your company can integrate AI agents into its transformation plan, connect with our experts.
FAQs
1. How do reactive AI agents differ from deliberative AI agents?
Reactive AI agents, such as reflex and model-based reflex systems, respond to environmental inputs with minimal internal reasoning. These agents perform best in high-volume, low-variation tasks, such as rule-based automation or responding to system alerts. In contrast, deliberative agents, including goal-based and utility-based models, evaluate multiple options to select the most effective action based on predefined objectives or value functions. These agents suit complex decision-making environments, such as dynamic pricing or supply chain optimization. Understanding this distinction helps organizations align AI capabilities with operational needs.
2. What separates multi-agent systems from single AI agents?
Multi-agent systems involve several autonomous agents that either collaborate or compete to achieve distributed goals. These systems manage decentralized tasks, including fraud detection, grid management, and route planning. Unlike single-agent models, which operate in isolation, multi-agent systems introduce added complexity in coordination, communication, and emergent behavior. Businesses often consider these systems when evaluating advanced AI solutions that require resilience, scalability, and adaptability across interconnected operations.
3. Which industries use intelligent agents for automation?
Intelligent agents now support automation across key sectors where speed, scale, and precision drive value. Healthcare, manufacturing, finance, logistics, retail, and telecom all integrate various types of AI agents to improve efficiency, customize services, and detect risks. In retail, for instance, learning agents forecast demand. In transportation, goal-based agents optimize schedules. By examining real-world use cases, companies can better assess their AI maturity and select agent types that align with long-term business objectives.
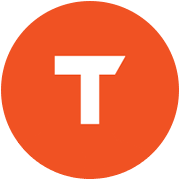
AUTHOR - FOLLOW
Editorial Team
Tredence