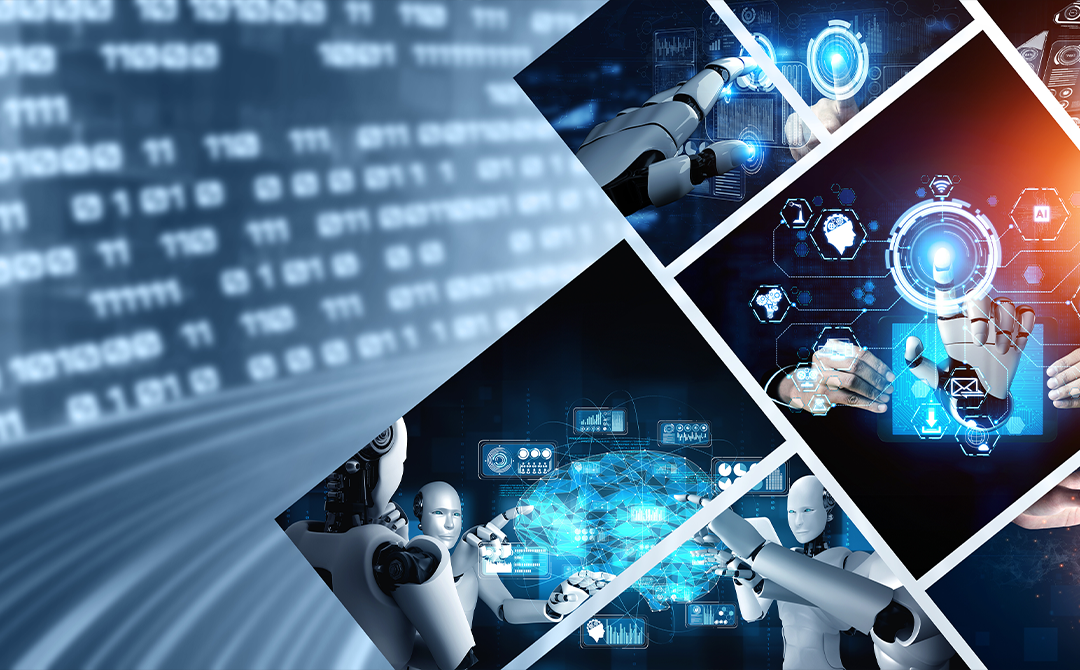
Artificial intelligence (AI) has made incredible strides in recent years, transforming industries and reshaping how we interact with technology. However, as AI systems become more and more complicated and pervasive, a crucial problem has emerged: the shortage of transparency in their choice-making processes. This concern has pushed the Explainable AI (XAI) sector upward, which seeks to shed light on the often-opaque internal workings of AI models.
In the cutting-edge panorama, AI systems are essential to choice-making throughout various sectors, from finance and healthcare to criminal justice and self-sufficient vehicles. The stakes are excessive, and the desire for duty and trust in AI consequences has not been significantly pronounced. Explainable AI companies are pivotal in addressing these challenges, offering frameworks and methods to enhance transparency and interpretability of AI models. This is when Explainable AI steps in, shifting from "black box" AI fashions to ones that offer understandable, interpretable, and honest reasons for their choices.
Types of XAI Methods
Explainable AI (XAI) strategies can be categorized into local and international factors primarily based on the scope of the interpretability provided.
Local motives assist in recognizing man or woman predictions, even as global factors offer insights into the model's behavior across the complete dataset. The choice of methods relies on the necessities and dreams of users and stakeholders worried.
Here's an overview of neighborhood and worldwide explainable AI techniques:
Local Explainable AI Methods
1. LIME (Local Interpretable Model-agnostic Explanations)
LIME generates local approximations of the complex model's behavior by perturbing the input data and observing the resulting prediction changes. It provides interpretable explanations for individual instances, offering insights into why a specific prediction was made.
2. Anchors
Anchors are conditions that must be true for a particular prediction to remain unchanged. This method defines a minimal set of features that act as "anchors" for a given instance, contributing to localized interpretability.
3. Counterfactual Explanations
Counterfactual explanations provide instances where the prediction changes. By showcasing the minimal changes needed to alter a prediction, users gain insights into the model's decision boundaries at the local level.
4. Shapley Values (Local Context)
While Shapley values can be applied globally, they are often used to explain the contribution of each feature for a specific prediction, offering a local interpretation.
5. Local Surrogate Models
Building simpler, interpretable models specifically for the local context to approximate the behavior of the complex model for a particular instance.'
6. Individual Feature Contributions
Examining the contribution of each feature to a specific prediction provides a localized understanding of how input features influence the model's output.
Global Explainable AI Methods
1. SHAP (SHapley Additive exPlanations)
SHAP values can be applied globally to explain the average contribution of each feature across all predictions. It provides a holistic view of feature importance and how each feature impacts the model's output on a broader scale.
2. Partial Dependence Plots (PDP)
PDP illustrates the relationship between a specific feature and the model's predictions while keeping other features constant. It helps visualize the overall impact of individual features across the entire dataset.
3. Feature Importance Metrics (Global Context)
Various metrics, such as Gini importance or permutation importance, assess the contribution of each feature to the model's predictions across the entire dataset.
4. Global Surrogate Models
Constructing simplified, interpretable models that approximate the behavior of the complex model on a global scale offers a more general understanding of the model's decision-making process.
5. Attention Mechanisms (Global Attention)
In neural networks, attention mechanisms can reveal which parts of the input data the model consistently focuses on across various instances, providing insights into global patterns.
6. Layer-wise Relevance Propagation (LRP)
LRP can be applied globally to allocate relevance scores to each input feature by propagating the output of a neural network backward. It helps to understand the overall contribution of each feature to the model's predictions.
In practice, a combination of local and global explanations is often necessary for a comprehensive understanding of a complex AI model.
Types of Metrics
Faithfulness Metric
The faithfulness metric in Explainable AI (XAI) assesses the degree of correspondence between the explanation of the importance of each feature. In other words, how well an interpretable model or explanation method faithfully represents the decisions made by the complex or black-box model it aims to explain. Faithfulness is crucial for ensuring that the explanations accurately capture the underlying reasoning of the AI system. It is determined by the Pearson sample correlation coefficient between the weights of the feature and its approximate contribution to the change in the model prediction when it is removed or fixed.
Monotonicity Metric
The monotonicity metric assesses whether changes in a feature's input values consistently result in changes in the model's output. In simpler terms, it checks whether the relationship between a feature and the model's prediction follows a monotonic pattern. If monotonicity isn't located, then the XAI approach allowed a distortion of characteristic priorities: a feature with less influence received greater weight, or vice versa.
Incompleteness Metric
The incompleteness metric in Explainable AI (XAI) assesses the degree to which an explanation method fails to capture and convey the essential aspects of a model's decision-making process. It evaluates whether the provided explanations lack crucial information, potentially leading to misunderstandings or misinterpretations.
Conclusion
Evaluating Explainable AI (XAI) systems is crucial to ensure transparency, reliability, and trustworthiness in AI-driven decision-making processes. Various metrics are employed to assess the effectiveness of XAI methods in providing understandable explanations of model predictions. However, it's essential to recognize the context-specific nature of XAI evaluation and continually refine evaluation methodologies to address evolving challenges and requirements in real-world applications.
Ensure transparency in AI systems through effective evaluation methods! Learn about reliable data science services focused on explainable AI technologies—contact us for more details!
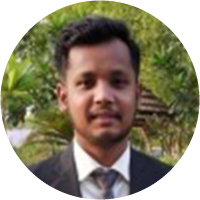
AUTHOR - FOLLOW
Rishav Chetan
Consultant, Data Science, Tredence Inc.
Topic Tags