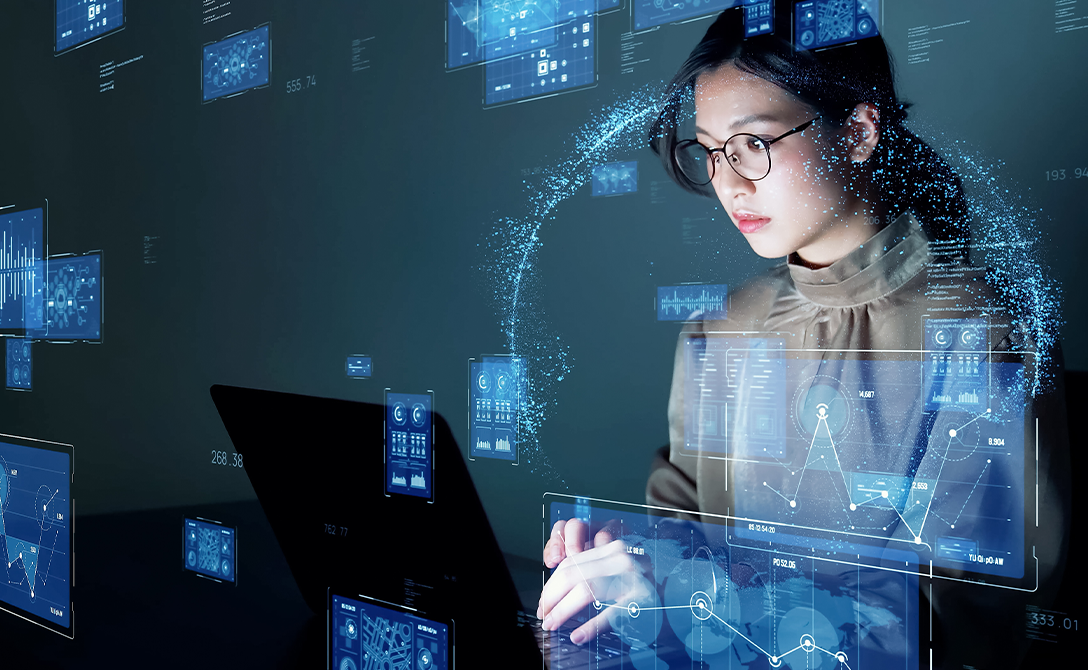
Company leaders often ask which AI project will get the highest and fastest return on investment. The answer is different for every organization. Soumendra Mohanty, chief strategy officer and innovation officer at Tredence Inc, provides a roadmap for employing AI for the biggest business benefit.
Business leaders are drawn to Artificial Intelligence to generate new revenue, save money, expand infrastructure to serve customers, and establish a sustainable competitive advantage. What’s often more difficult is determining the real value of AI investments. That’s understandable since AI can spark questions about organizational maturity to understand and adopt solutions, the costs of building and deploying AI solutions, the readiness of data and platforms to innovate, in-house skills and competency to execute AI projects, and similar issues of planning and execution.
These are all critical issues for CXOs concerned with the development and deployment of AI solutions. AI is an experimental and expensive initiative, therefore, it’s essential to have a playbook to help determine ROI for enterprise investments.
Not All AI Projects Are the Same
One way to think about an AI project is to understand what outcomes it will deliver and when: short-term projects that deliver immediate value in the near term and moonshot projects that deliver significant value over the long term.
For short-term AI projects, ROI is generally determined through quantifiable factors like improved productivity, process efficiency, cost savings and visibility into operations. On the other hand, long-term AI projects are concerned with strategic growth-oriented objectives, such as new revenue sources, market differentiation, identifying and creating new products for unmet customer needs, and more.
Alternatively, one can also think of breaking down AI projects into the following categories: automation, continuous learning, and autonomous.
- Automation entails finding enough patterns in repetitive rote tasks, automating those with predictable outputs and enabling the higher-value imaginative activity to be performed by humans, which all fall into the category of short-term AI projects.
- Continuous learning incorporates the ability to learn from observations and apply the learning to solve previously unseen problems, just as a human would do throughout their lifespan, which falls into the category of short- to medium-term AI projects.
- Autonomous AI projects focus on generating intelligence that allows systems to act on their own, independent of human intervention, which is a long-term AI project.
Now that we have a fair idea of the types of AI projects, let’s reflect on what questions organizations should ask before initiating a project.
Questions to Ask When Measuring ROI in AI Projects
It’s important to answer these questions that help in measuring the complexity of the AI project and to formulate key success criteria:
- Partnering with AI-friendly business units: Do you have a broad base of business sponsorship and alignment within the organization for AI initiatives? Are your business unit leaders familiar with the technology and the outcomes it may deliver?
- Selection of projects: Do you have enough clarity regarding the AI use cases in consideration of tangible values and a direct production path? The ability of AI and analytics initiatives to generate concrete value varies, hence you will need some low-hanging fruit to keep the success stories going and balance out more ambitious projects.
- Fostering stakeholder trust: Do you have strong sponsorship from the heads of the functions or units where the AI applications will be implemented? Have you and your finance team agreed on the KPIs and metrics for “monetizing” the AI project? This is critical and needs to be determined prior to launching a project, including high-level cost estimates and expectations.
- Learn fast and deploy faster: Do you have the right tools, methodologies, governance framework and change management practices to manage the AI project lifecycle? All AI projects start as experimental in nature; they go through several iterations and course corrections during the development phase. It is advisable to start with a pilot to assess and illustrate the value of the application because early detection of failure points can allow for quick adjustments and go/no-go decisions saving time, energy, money and critical resources.
- AI Infrastructure: Do you have the AI infrastructure, including data to develop, test and deploy the application? AI projects need a lot of data and require the right tools, algorithms, compute power and scalable infrastructure to make the project successful.
See More: How AI Is Transforming Industries With Smart Inventory Management
Measuring the ROI of an AI Project
Given the uncertainty and countless experiments required in any AI project, there is no guarantee that a project will not go over budget and deliver business value. Though almost every company recognizes AI’s power, most struggle to unlock its full potential. The problem is that AI investments should not only deliver value but should also lay the groundwork to aid in the rapid development of future AI use cases.
The most successful companies treat AI applications like a product. Often that means developing a base AI product that can then serve as the foundation to spur many other future AI use cases as opposed to developing an AI application to serve a specific purpose that cannot be extended. Over time the AI product learns and matures with the addition of new functionality in response to user feedback, performance evaluations and changes in the end-user journey. All the while, the company needs to find ways to increase production efficiency—wherever possible, by reusing the AI product building blocks, data assets and algorithms. To gain maximum return from your AI investments, you need to have a balanced approach to delivering value with it today and paving the way for quickly getting more value out of it tomorrow.
To determine if a project is successful, AI projects can be evaluated with barometers such as the impact on sales, customer engagement and satisfaction, profitability, active monthly users, the number of applications across the business, and the return on investment for use cases, to name a few. Here is a comprehensive list of factors to take into consideration to arrive at the ROI of your AI projects.
- Step 1: Make a list of all the costs associated with the development of the AI project. These include:
- People costs, including in-house personnel and external partners
- Licenses and tools costs, including platforms and security
- Prototyping and designing costs
- Data preparation costs
- Algorithm’s development costs
- Model training, test, deployment and maintenance costs
- End-user training, change management and adoption costs
- Step 2: Bring the values/benefits of AI investments into a single pane of view. Making an expense list is easier than estimating the benefits or value an investment in AI will produce. Categories of benefits, which any ROI calculation requires, include:
- Revenue
- Efficiency
- Productivity
- Reputation and Compliance
- Sustainability
- Resilience
- Assets
- Product and Service Quality
- Brand Identity
- Market Share
- Pricing Power
- Organizational Culture
- Knowledge
- Quality of work
- Step 3: Compare the costs and benefits while determining cash flow. This will help you develop a comprehensive business case for your AI investment.
Apply your findings to a Value Realization Framework that clearly articulates the cost-benefit scenario and helps in the prioritization of AI investments based on the potential business value they will generate.
- Optimize ROI: Prioritize use cases that have a high return on investment
- Reduce risk and complexity: Address simpler applications first to build confidence in technology and then advance to more complex issues
- Centralize data for easy access: In some cases, you might already have the data required to start the use case in a centralized and ready-to-use repository. You do not have to go through the full cycle of data collection
- Business criticality: At the core of the business process, it is critical to upgrade data to meet competitive demand and expand the customer base
- Make plans for ongoing enhancements: Re-engineering legacy business processes requires significant investments. Consider embedding AI
- Recognize quick-win patterns: Known success patterns available in the industry that can be easily replicated in one’s enterprise process and data
Leaders often ask which AI project will get the highest and fastest return on investment. The answer is different for every organization.
For executives to determine the most appropriate approach, it is essential to assess the feasibility and potential value of AI use cases in different business domains. Investment decisions often involve trade-offs between value, viability, and speed. However, feasibility considerations may cause a company to adjust its approach. The best place to start building momentum may be an area within the organization with better data literacy and some traction with AI, even if it’s not what offers the biggest opportunity.
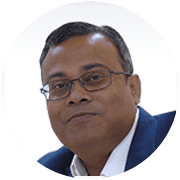
AUTHOR - FOLLOW
Soumendra Mohanty
Chief Strategy Officer & Chief Innovation Officer
Topic Tags