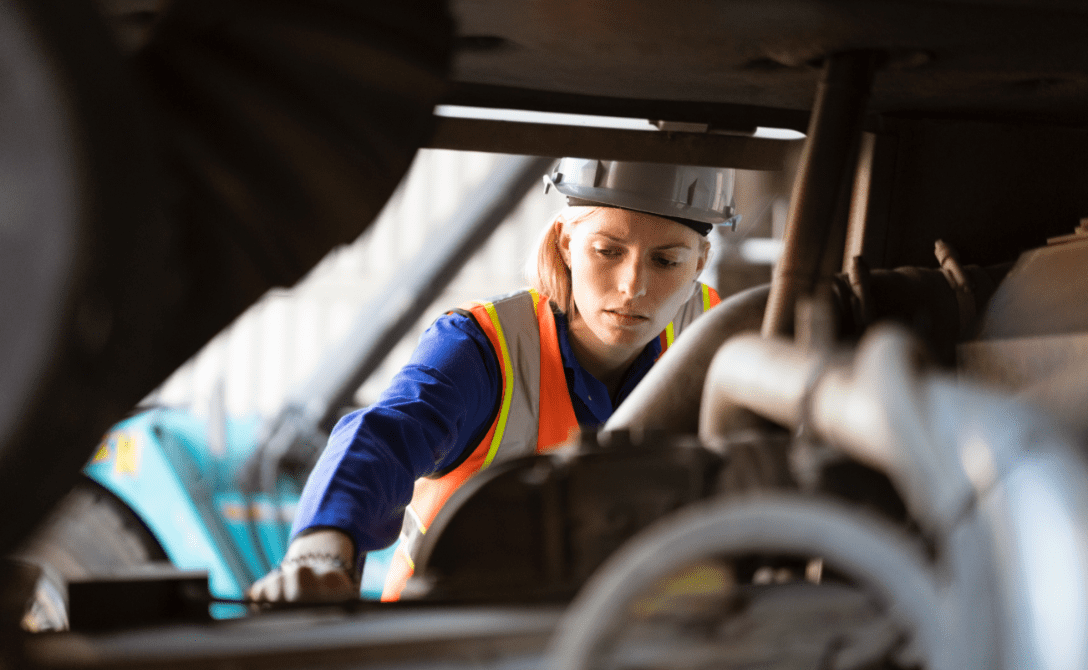
Streamlining the Machine Learning Lifecycle: An Introduction to MLOps and its Importance
Machine Learning (ML) – a tech buzz phrase that has been at the forefront of the tech industry for years. It is used in various applications, from weather forecasts to news feeds on your social media platform. It focuses on developing computer programs that can acquire data and “learn” by recognizing patterns and making decisions. However, building, deploying, and managing these models is not simple and requires specialized skills. This is where MLOps, comes in.
MLOps, also known as Machine learning Ops, or Model Ops, aims to streamline the ML lifecycle, from development to deployment, by automating and optimizing the process. MLOps platform is essential for organizations that want to leverage the power of ML to drive business value but don't have the resources or expertise to manage the complexity of the ML lifecycle.
Unfortunately, the average data scientist spends only 20% of their time on modeling, with the other 80% being spent on the ML lifecycle. MLOps helps bridge the gap between data scientists and IT teams, allowing them to collaborate more effectively and streamline the building, deploying, and managing of ML models. It also helps to ensure that models are deployed securely and compliant while providing a way to monitor and maintain models in production.
This blog will dive deeper into the machine learning lifecycle before delving into MLOps.
Building
This exciting step is unquestionably the highlight of the job for most data scientists. This is where they can stretch their creative muscles and design models that best suit the application’s needs. This is where Tredence believes that data scientists should spend most of their time maximizing their value to the firm.
Data Preparation
Though information is easily accessible in this day and age, there is no universally accepted format. Data can come from various sources, from hospitals to IoT devices; to feed the data into models, sometimes, transformations are required. For example, machine learning algorithms generally need data to be numbers, so textual data may need to be adjusted. Statistical noise or errors in data may also need to be corrected.
Model Training
Training a model means determining good values for all the weights and bias in a model. Essentially, the data scientists are trying to find an optimal model that can minimize loss – an indication of how badly the prediction is performed on a single example.
Parameter Selection
During training, it is necessary to select some parameters that will impact the prediction of the model. Although most are selected automatically, some subsets cannot learn and require expert configuration. These are known as hyperparameters. Experts trying to configure hyperparameters have to implement various optimization strategies to tune the hyperparameters.
Transfer Learning
It is quite common to reuse machine learning models across various domains. Although models may not be directly transferrable, some can serve as excellent foundations or building blocks for developing other models.
Model Verification
At this stage, the trained model will be tested to see if the validated model can provide sufficient information to achieve its intended purpose. For example, when the trained model is presented with new data, can it still maintain its accuracy?
Deployment
At this point, the model has been thoroughly trained & tested and has passed all requirements. The step aims to use this model for the firm and ensure that it can continue to perform with a live stream of data.
Machine Learning Monitoring
Now that the model is deployed and live, many businesses generally consider the process to be final. Unfortunately, this is far from reality. Like any tool, the model will wear out after use. If not tested regularly, it will provide irrelevant information. To make matters worse, since most machine learning models work in a “black box,” they lack the clarity to explain the model’s predictions, making the predictions challenging to defend.
Without this entire process, models would never see the light of day. That said, the process often weighs heavily on data scientists, simply because many steps require direct actions on their end. Enter MLOps, or Model Ops.
MLOps (Machine Learning Operations) is a set of practices, frameworks, and tools that combines Machine Learning, DevOps, and Data Engineering to deploy and maintain ML models in production reliably and efficiently. MLOps solutions provide Data engineers, scientists, and engineers with the necessary tools to make the entire process a breeze. Next time, find out how Tredence Engineers have developed a tool that targets one of these steps to make the lives of data scientists’ easier.
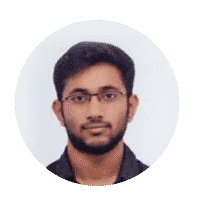
AUTHOR - FOLLOW
Jai Vardhan Rao
Associate Product Manager
Topic Tags
Detailed Case Study
Driving insights democratization for a $15B retailer with an enterprise data strategy
Learn how a Tredence client integrated all its data into a single data lake with our 4-phase migration approach, saving $50K/month! Reach out to us to know more.
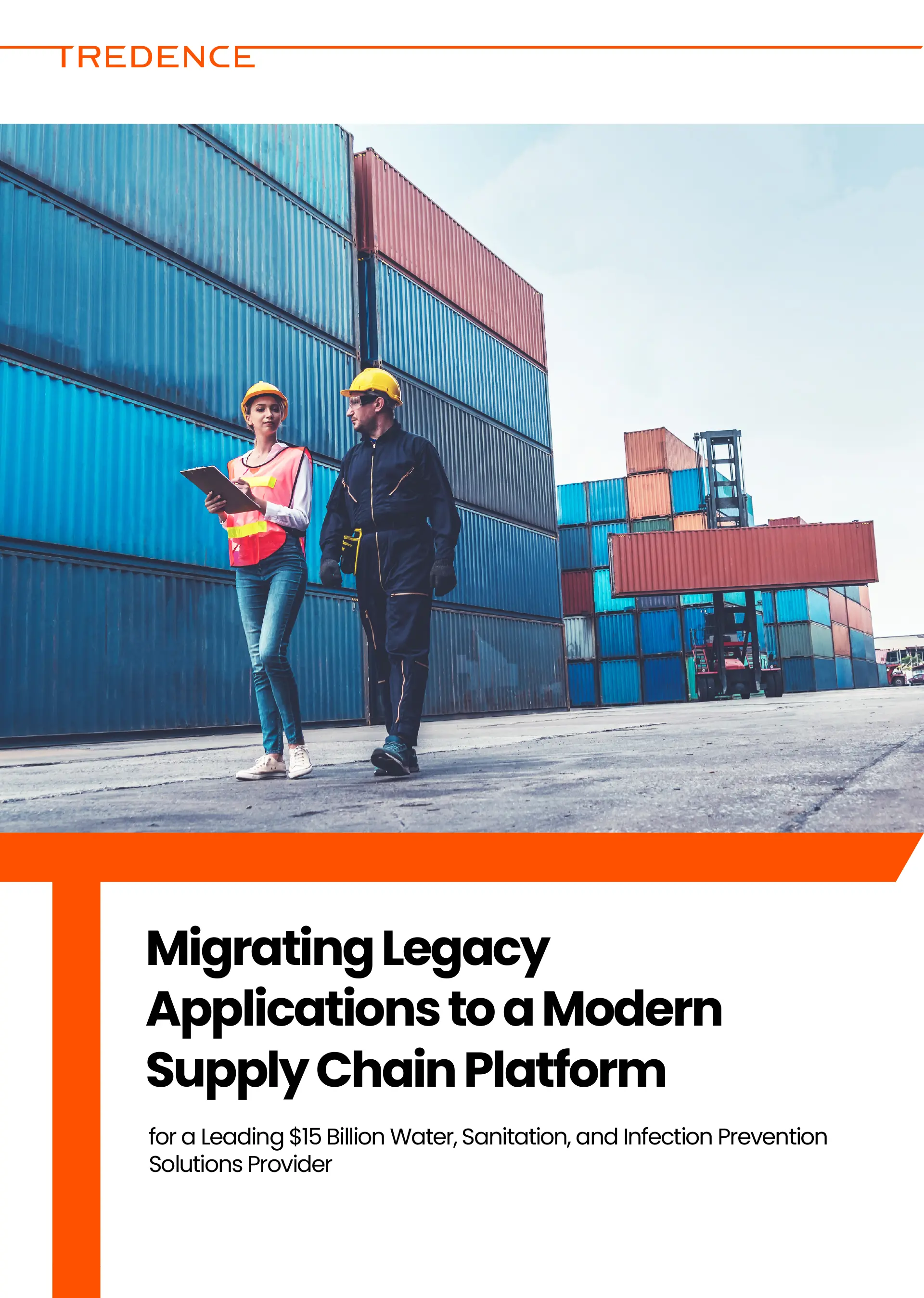
Detailed Case Study
MIGRATING LEGACY APPLICATIONS TO A MODERN SUPPLY CHAIN PLATFORM FOR A LEADING $15 BILLION WATER, SANITATION, AND INFECTION PREVENTION SOLUTIONS PROVIDER
Learn how a Tredence client integrated all its data into a single data lake with our 4-phase migration approach, saving $50K/month! Reach out to us to know more.
Next Topic
The rise of AI in art: Ushering a new era of machines with creative behaviors
Next Topic